Home>Finance>Sampling Errors In Statistics: Definition, Types, And Calculation
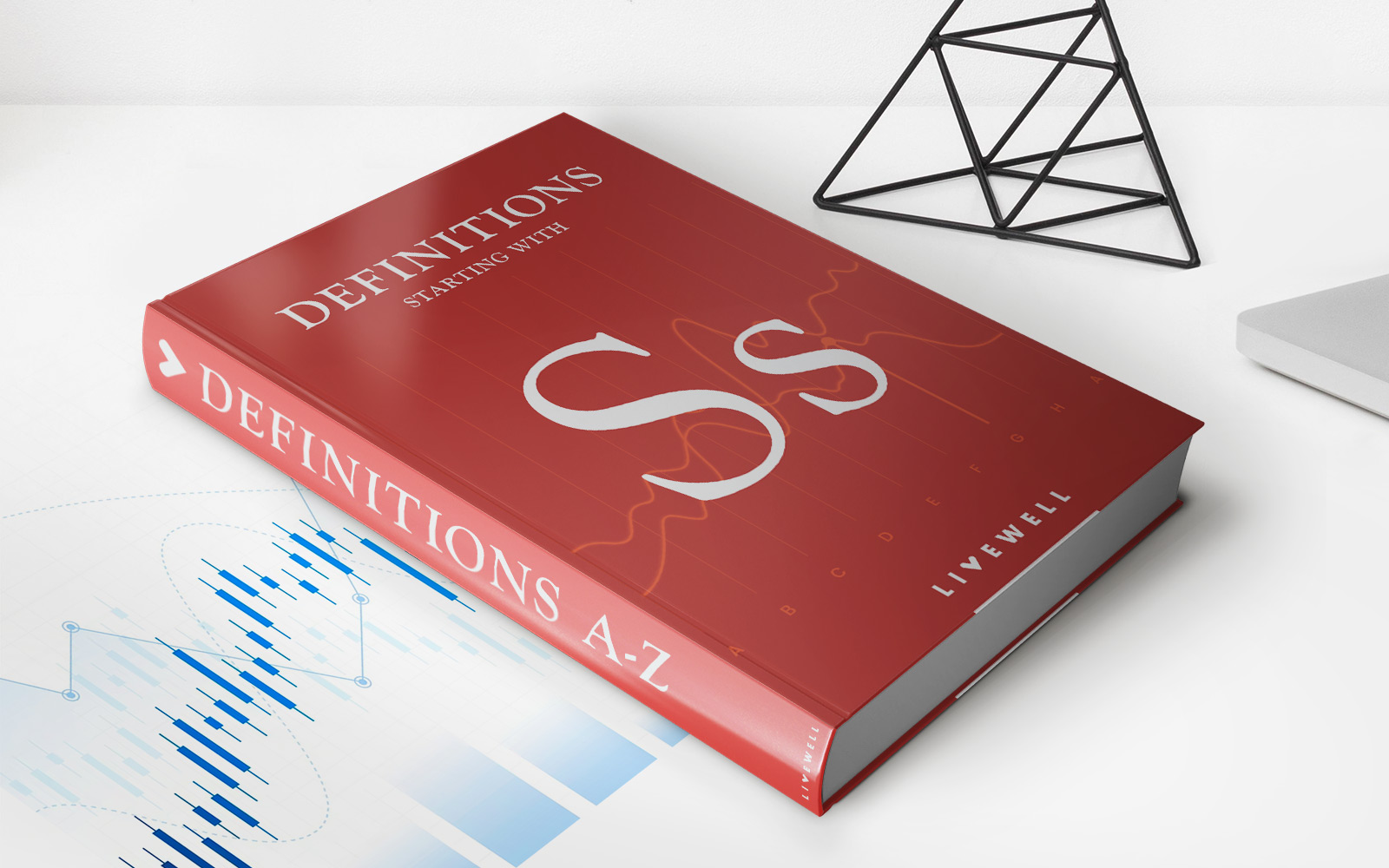
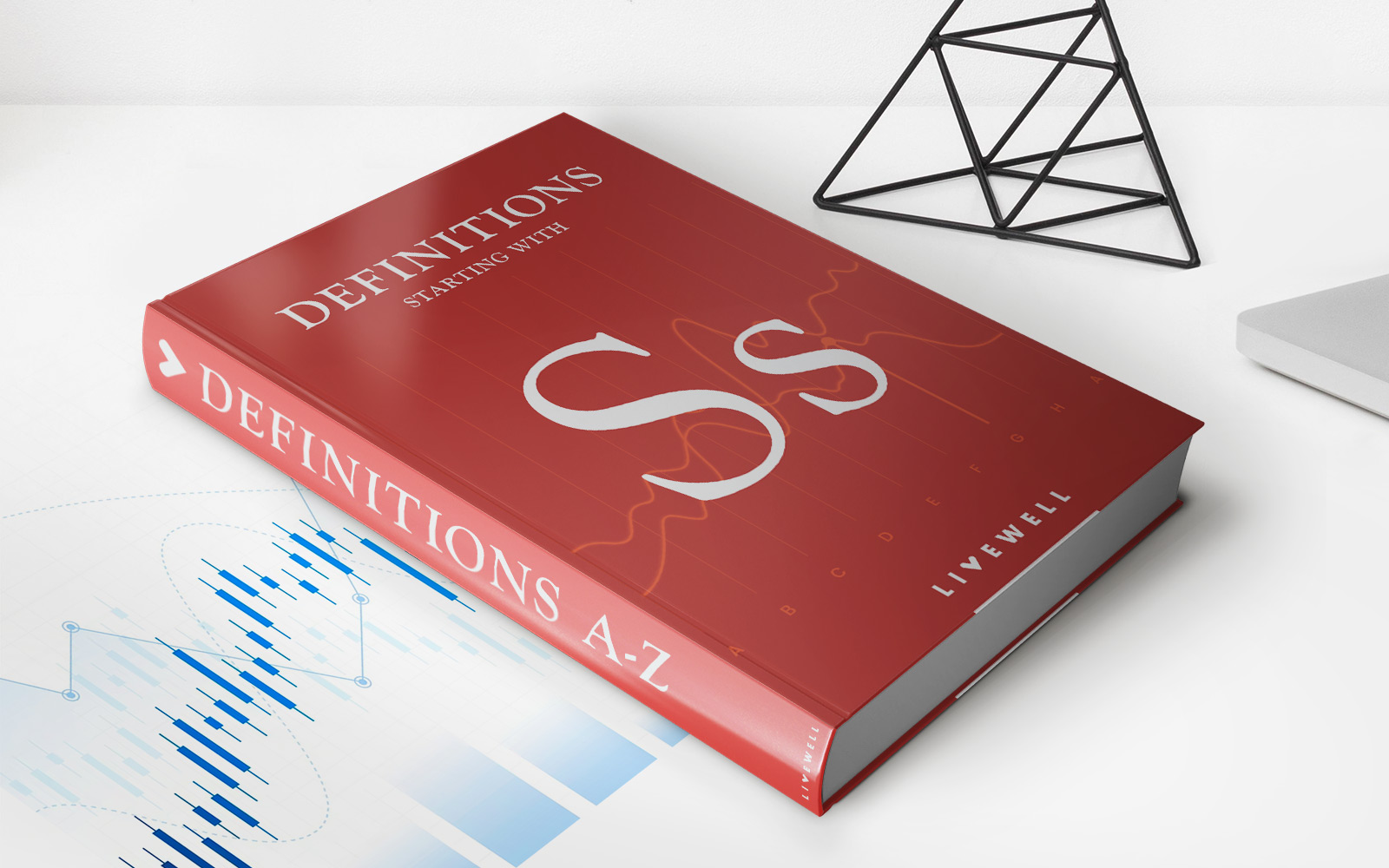
Finance
Sampling Errors In Statistics: Definition, Types, And Calculation
Published: January 23, 2024
Learn about sampling errors in statistics, including their definition, types, and how to calculate them. Find out how they impact finance.
(Many of the links in this article redirect to a specific reviewed product. Your purchase of these products through affiliate links helps to generate commission for LiveWell, at no extra cost. Learn more)
Welcome to the World of Sampling Errors in Statistics!
Have you ever wondered how statisticians draw conclusions about a large population without examining every single individual? Well, this is where sampling comes into play. Statistical sampling involves selecting a subset, or sample, from a larger group, or population, to represent the characteristics of the whole. It allows for efficient data collection and analysis, but it’s essential to understand that sampling is not error-proof. In fact, it introduces its own set of potential errors known as sampling errors.
Key Takeaways
- Sampling errors occur when the selected sample does not accurately represent the entire population.
- Random sampling, stratified sampling, and cluster sampling are common methods used to minimize sampling errors.
Sampling errors can arise due to various factors, including inadequate sample size, biased sampling techniques, and natural variability within the population. It is important to acknowledge and account for these errors to ensure the validity and reliability of statistical conclusions. So, let’s dive deeper into the definition, types, and calculation of sampling errors!
Definition of Sampling Errors
Sampling errors are the differences between the characteristics of a sample and the characteristics of the population it is supposed to represent. In other words, they occur when the selected sample does not accurately reflect the true values, patterns, or relationships present in the entire population. Sampling errors can lead to misleading or inaccurate conclusions, posing a challenge to researchers and statisticians.
Types of Sampling Errors
Sampling errors can manifest in several ways. Let’s explore some common types:
- Random Sampling Errors: Random sampling errors occur due to chance and natural variability within the population. Even with a perfectly random sample, there will always be some level of variation. While it is impossible to eliminate random sampling errors entirely, increasing the sample size generally reduces their impact.
- Systematic Sampling Errors: Systematic sampling errors arise from biases in the sampling process. This can happen when certain groups or individuals in the population are more likely to be included or excluded from the sample. For example, if surveys are conducted only during business hours, it might exclude individuals with different lifestyles or occupations. These errors can distort the representation of the population and lead to biased conclusions.
- Non-Response Errors: Non-response errors occur when selected individuals or groups fail to participate in the study. This can happen for various reasons, such as refusal, inability to reach the respondent, or not completing the survey. Non-response can introduce bias if non-respondents differ systematically from respondents in terms of the characteristics being studied.
- Measurement Errors: While not strictly a sampling error, measurement errors can still impact the validity and reliability of statistical conclusions. Measurement errors occur when there are inaccuracies in data collection methods or instruments. For example, if a measuring scale is not properly calibrated, it can introduce errors that affect the accuracy of the results.
Calculating Sampling Errors
Calculating sampling errors involves comparing the observed characteristics of the sample to the known or estimated characteristics of the entire population. To quantify these errors, various statistical methods and techniques can be employed. Some common measures used to calculate sampling errors include:
- Confidence Intervals: Confidence intervals provide a range of values within which the population parameter is likely to fall. The width of the confidence interval is influenced by the sample size and the desired level of confidence. A wider interval indicates a higher level of uncertainty or sampling error.
- Margin of Error: The margin of error is the maximum expected difference between the sample statistic and the true population parameter. It is usually expressed as a percentage and is influenced by factors such as the sample size and the level of confidence selected.
By understanding the potential types of sampling errors and employing appropriate statistical measures, statisticians can minimize the impact of these errors and ensure more accurate and reliable conclusions. It is essential to remember that sampling errors are an inherent part of statistical sampling, but with careful consideration and analysis, we can navigate through them with confidence.
Now that you have a solid grasp of sampling errors in statistics, it’s time to put this knowledge to use in your own research endeavors! Remember to pay attention to factors like sample size, sampling techniques, and variation within the population to minimize errors and draw accurate conclusions. Happy researching!