Home>Finance>Stepwise Regression: Definition, Uses, Example, And Limitations
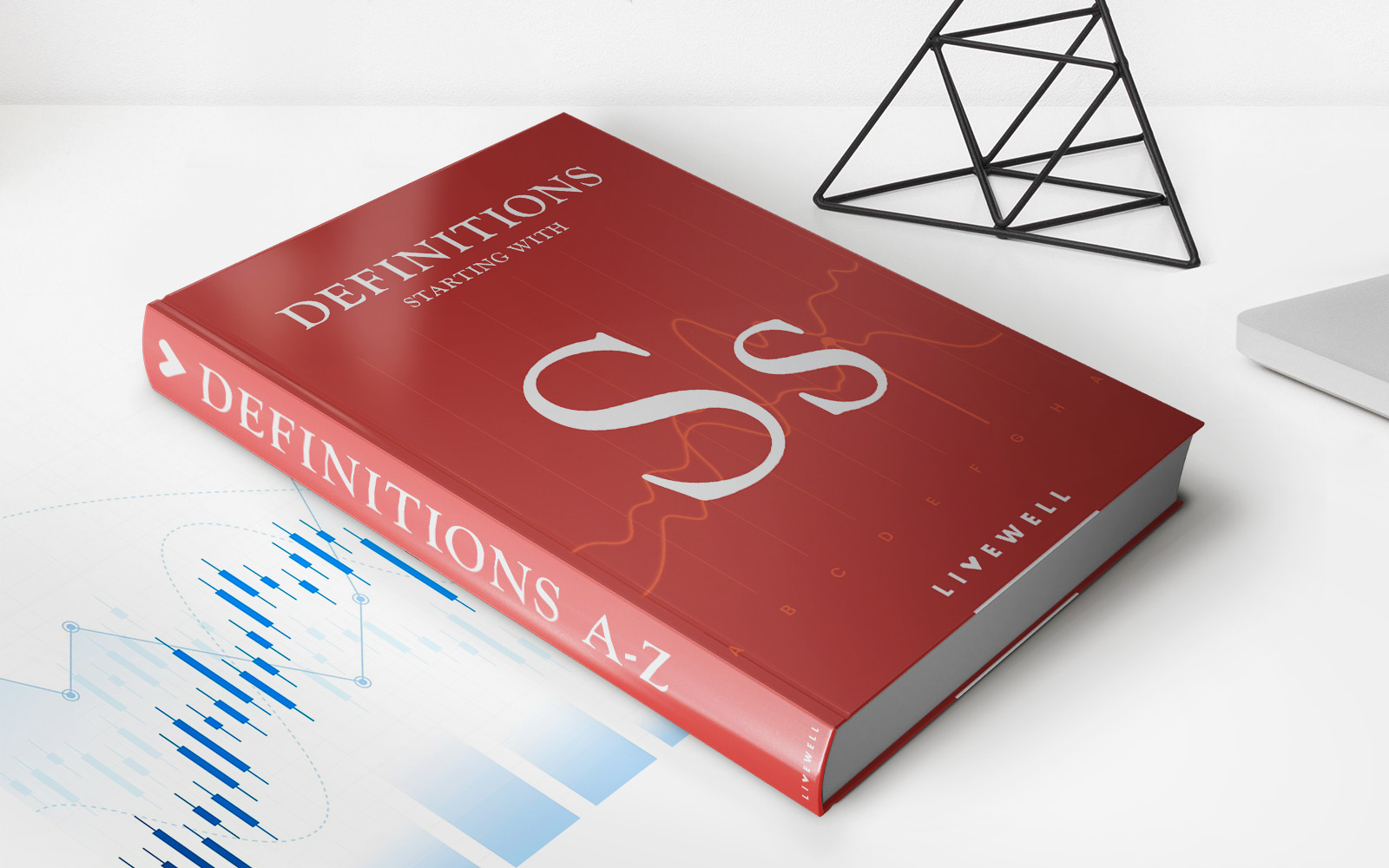
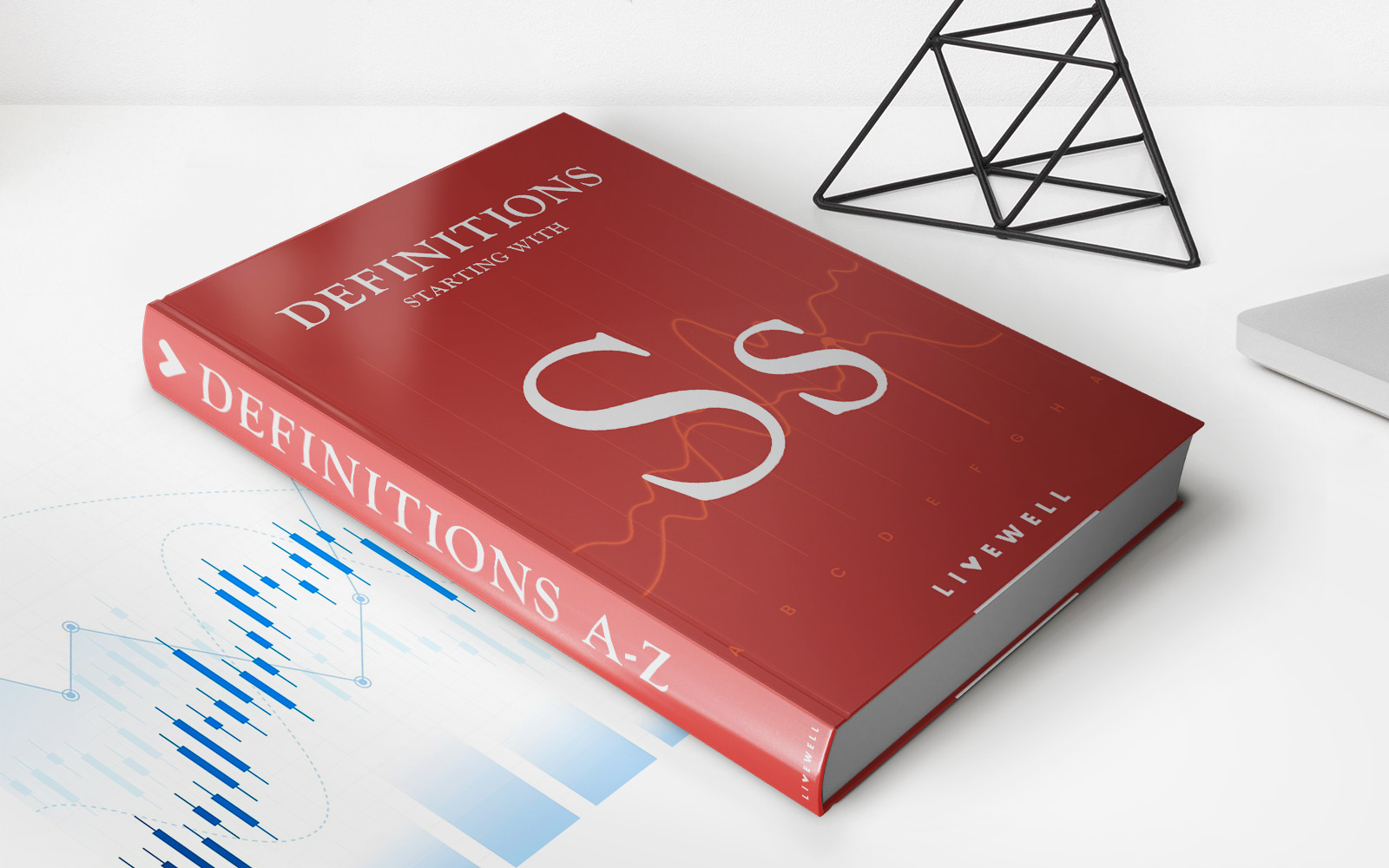
Finance
Stepwise Regression: Definition, Uses, Example, And Limitations
Published: February 2, 2024
Learn about stepwise regression in finance, its definition, uses, example, and limitations. Enhance your understanding of this statistical technique for financial analysis.
(Many of the links in this article redirect to a specific reviewed product. Your purchase of these products through affiliate links helps to generate commission for LiveWell, at no extra cost. Learn more)
Stepwise Regression: Definition, Uses, Example, and Limitations
If you find yourself tackling a complex financial analysis or modeling problem, stepwise regression might be just the tool you need to bring order and clarity to your analysis. In this blog post, we will explore the definition, uses, and limitations of stepwise regression, and provide an example to help you understand how it can be applied in the realm of finance.
Key Takeaways:
- Stepwise regression is a statistical technique used to identify the most significant variables in a regression model.
- It can be used in financial analysis to determine the key factors influencing a specific financial outcome, such as predicting stock prices or analyzing the factors affecting a company’s profitability.
What is Stepwise Regression?
Stepwise regression is a regression modeling method that aims to find the best combination of variables to include in a model. It works by iteratively selecting variables to add or remove from the model based on their statistical significance. The goal is to build a model that maximizes predictive accuracy while minimizing the number of variables included.
Stepwise regression is particularly useful when dealing with large datasets where there are numerous potential predictor variables. By automating the selection process, it saves time and helps to identify the most important variables driving the outcome of interest.
Uses of Stepwise Regression in Finance
Stepwise regression has a wide range of applications in the field of finance. Here are a few examples:
- Stock Price Prediction: Stepwise regression can be used to identify the key variables that influence stock prices. By analyzing factors such as company financials, market conditions, and industry trends, stepwise regression can help forecast future stock prices.
- Financial Risk Assessment: Stepwise regression can assist in determining the key factors influencing financial risk. By examining variables such as debt levels, credit ratings, and market volatility, stepwise regression can aid in assessing the likelihood of financial distress.
- Profitability Analysis: Stepwise regression can be used to analyze the key drivers of profitability in a company. By examining factors such as sales, expenses, and market conditions, stepwise regression can identify the variables that have the greatest impact on a company’s bottom line.
Example of Stepwise Regression
Let’s consider an example of stepwise regression in the context of stock pricing. Assume we have a dataset that includes variables such as company earnings, interest rates, and stock market indices. We want to build a regression model that predicts stock prices based on these variables.
Using stepwise regression, we can iteratively add or remove variables from our model, based on their statistical significance. The algorithm evaluates each variable’s contribution and decides whether to include it or exclude it from the model.
After several iterations, the stepwise regression process selects the most relevant variables for our model. These variables may include earnings per share, bond yields, and the S&P 500 index. The final model then provides insights into how these variables impact stock prices and can be used to make predictions.
Limitations of Stepwise Regression
While stepwise regression can be a powerful tool in financial analysis, it is not without its limitations. Here are a few key considerations:
- Stepwise regression assumes that the relationship between variables is linear and that there are no interactions among them. This assumption may not hold true in all cases.
- It is susceptible to overfitting the data, especially when dealing with large datasets and a large number of potential predictor variables. This can result in a model that performs well on the training data but fails to generalize to new data.
- Stepwise regression can be computationally intensive, especially when dealing with a large number of predictor variables. It may not be suitable for large-scale datasets with limited computing resources.
Despite these limitations, stepwise regression remains a valuable technique in the field of finance. When used appropriately and with careful consideration of its limitations, it can provide insights and aid in decision-making processes.
In conclusion, stepwise regression is a statistical tool that can help bring order and clarity to complex financial analysis problems. By automating the variable selection process, it enables analysts to identify the most significant variables in a model and gain valuable insights into the factors driving financial outcomes. Remember to use stepwise regression as a guide, considering its limitations and interpreting the results with caution.