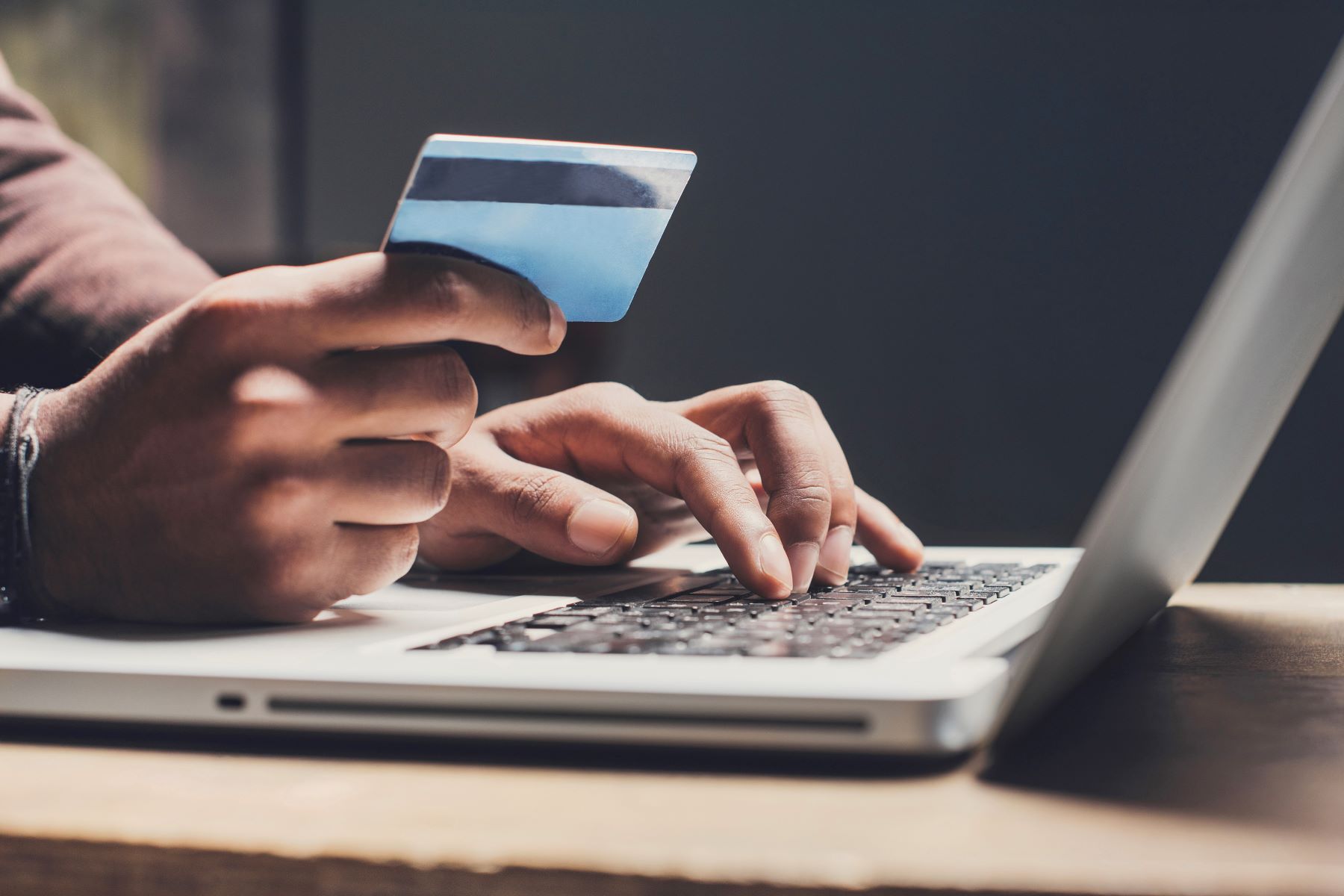
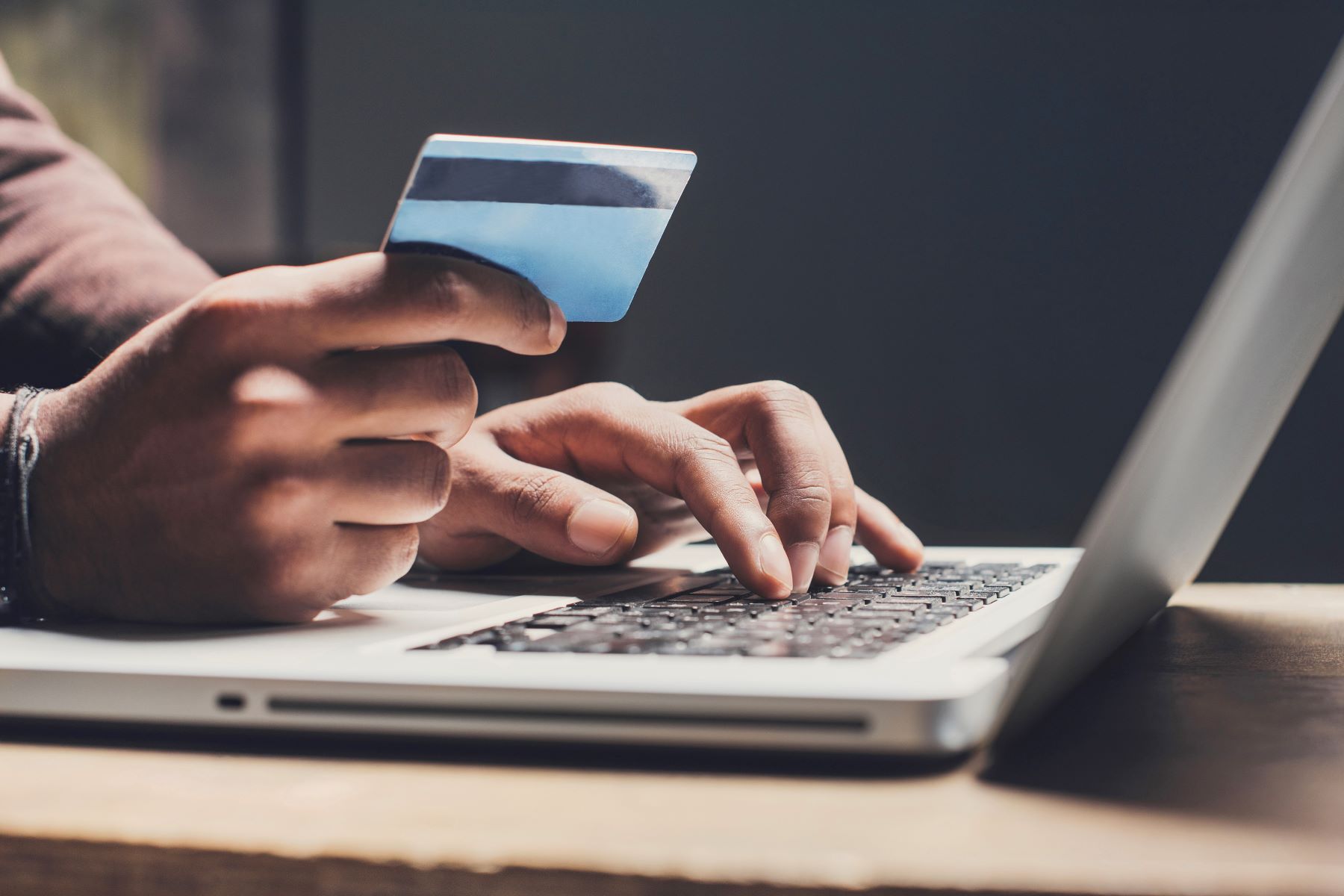
Finance
What Is Credit Card Fraud Detection System
Published: October 27, 2023
Learn how finance professionals use credit card fraud detection systems to protect against fraudulent transactions and safeguard financial information.
(Many of the links in this article redirect to a specific reviewed product. Your purchase of these products through affiliate links helps to generate commission for LiveWell, at no extra cost. Learn more)
Table of Contents
- Introduction
- Understanding Credit Card Fraud
- Importance of Credit Card Fraud Detection Systems
- Components of a Credit Card Fraud Detection System
- Data Collection and Monitoring
- Fraud Detection Techniques
- Machine Learning Algorithms for Fraud Detection
- Real-time Monitoring and Alerting Systems
- Case Studies of Successful Credit Card Fraud Detection Systems
- Challenges and Limitations of Credit Card Fraud Detection Systems
- Future Trends in Credit Card Fraud Detection
- Conclusion
Introduction
Welcome to the world of finance, where the convenience of credit cards has transformed the way we make transactions. However, along with the ease of use and accessibility comes the risk of credit card fraud. In recent years, credit card fraud has become a major concern for both consumers and financial institutions alike.
Credit card fraud refers to the unauthorized use of someone’s credit card information for fraudulent purposes, such as making purchases, withdrawing cash, or even opening new accounts. The impact of credit card fraud can be devastating, leading to financial loss, damaged credit scores, and personal stress.
To combat this ever-evolving threat, financial institutions and payment processors have developed sophisticated credit card fraud detection systems. These systems leverage advanced technologies and analysis techniques to identify and prevent fraudulent activities in real-time.
The importance of credit card fraud detection systems cannot be overstated. As the digital world continues to expand, the methods employed by fraudsters become increasingly sophisticated. Therefore, financial institutions and businesses must stay one step ahead to safeguard their customers’ sensitive information.
In this article, we will delve into the world of credit card fraud detection systems. We will explore the various components that make up these systems, the techniques used to detect and prevent fraud, and the challenges faced in implementing effective fraud detection mechanisms. Additionally, we will discuss real-world case studies and the future trends shaping the field of credit card fraud detection.
Before we dive deeper into the subject, let’s take a moment to acknowledge the significance of these systems and the role they play in ensuring secure transactions for individuals and organizations alike.
Understanding Credit Card Fraud
Credit card fraud is a form of financial fraud that involves the unauthorized use of someone’s credit card information. Fraudsters use various techniques to obtain credit card details, including phishing scams, skimming devices, and data breaches. Once they have access to this information, they can make purchases, withdraw cash, or sell the data to other criminals.
There are several types of credit card fraud, including:
- Lost or Stolen Card Fraud: This occurs when a credit card is physically lost or stolen, and the perpetrator makes unauthorized purchases.
- Application Fraud: In this type of fraud, individuals use someone else’s identity to open credit card accounts.
- Card-Not-Present Fraud: With the rise of online shopping, card-not-present fraud has become more prevalent. It involves using stolen credit card information to make purchases online, over the phone, or through mail-order transactions.
- Counterfeit Card Fraud: Fraudsters create fake credit cards using stolen information and use them to make purchases in person.
Credit card fraud can have severe consequences for victims, including financial losses and damage to their credit history. It can also impact businesses and financial institutions, leading to increased costs, damaged reputation, and customer mistrust.
To combat credit card fraud, it is crucial to understand the common red flags and warning signs. Some indicators of potential fraudulent activity include:
- Unusual or large transactions that are not consistent with the cardholder’s spending patterns.
- Multiple declined transactions in a short period.
- Unfamiliar billing or shipping addresses.
- Unauthorized changes to account information, such as contact details or PIN numbers.
- Unusual overseas transactions.
By being vigilant and monitoring for these warning signs, individuals and organizations can take steps to mitigate the risk of credit card fraud. However, it’s important to remember that prevention alone is not enough. To effectively combat credit card fraud, advanced detection and prevention systems are necessary.
Importance of Credit Card Fraud Detection Systems
Credit card fraud is a serious and pervasive problem that can have far-reaching consequences for individuals and businesses. This is where credit card fraud detection systems play a vital role in safeguarding financial transactions and protecting consumers.
1. Financial Loss Prevention: Credit card fraud detection systems are essential for preventing financial losses. By identifying suspicious or fraudulent transactions in real-time, these systems can stop fraudulent activity before it can cause significant damage.
2. Protection of Consumer Data: Credit card fraud detection systems protect sensitive consumer data. By constantly monitoring transactions and analyzing patterns, these systems can identify and block unauthorized access to credit card information, preventing potential data breaches.
3. Maintaining Trust and Reputation: Implementing effective fraud detection systems demonstrates a commitment to customer protection. It helps maintain trust between consumers and businesses, ensuring that customers feel confident and secure when making transactions.
4. Reducing Chargebacks: Chargebacks occur when customers dispute a transaction and request a refund directly from the credit card company. By detecting and preventing fraudulent transactions, credit card fraud detection systems can reduce the number of chargebacks, saving businesses time and money.
5. Compliance with Regulations: Financial institutions and businesses are subject to various regulations related to data security and fraud prevention. Implementing credit card fraud detection systems helps businesses adhere to these regulations, avoiding potential fines and penalties.
6. Early Fraud Detection: Fraudsters are constantly evolving their techniques, making it essential to have advanced fraud detection systems in place. These systems can identify patterns and anomalies indicative of fraudulent activity, enabling early detection and prevention.
7. Improving Operational Efficiency: Credit card fraud detection systems automate the process of monitoring transactions and identifying potential fraud, reducing the need for manual intervention. This improves operational efficiency and allows businesses to allocate resources more effectively.
Given the wide range of benefits that credit card fraud detection systems offer, it is clear that investing in robust and sophisticated systems is crucial in today’s digital landscape. These systems provide the necessary protection to consumers, businesses, and financial institutions, ensuring secure and trustworthy financial transactions.
Components of a Credit Card Fraud Detection System
A credit card fraud detection system comprises various components that work together to detect and prevent fraudulent transactions. These components include:
- Data Collection: The first step in a fraud detection system is the collection of data. This includes gathering transactional data, historical customer data, and external data sources such as blacklists and fraud databases.
- Data Pre-processing: Once the data is collected, it needs to be pre-processed to prepare it for analysis. This involves cleaning the data, removing duplicates, handling missing values, and transforming it into a suitable format for analysis.
- Feature Extraction: Feature extraction involves selecting relevant features from the data that will be used to detect fraudulent transactions. These features can include transaction amount, location, time of day, IP address, and behavioral patterns.
- Model Building: Model building is a crucial component of fraud detection systems. It involves developing algorithms or models that can analyze the data and identify patterns indicative of fraud. Commonly used models include machine learning algorithms, rule-based systems, and anomaly detection algorithms.
- Model Training: Before the models can be used in production, they need to be trained using historical data. This allows the models to learn from past fraudulent and non-fraudulent transactions, enabling them to make accurate predictions in real-time.
- Real-time Monitoring: Real-time monitoring involves the continuous monitoring of incoming transactions in real-time. This allows the system to identify suspicious patterns or anomalies and flag them for further investigation.
- Alerting and Reporting: When a potentially fraudulent transaction is detected, the system generates an alert or notification to notify the appropriate personnel. This enables immediate action to be taken to prevent further fraudulent activity. Reporting capabilities are also essential for tracking and analyzing fraud patterns and trends.
- Case Management: Case management tools are used to manage and track the investigation process when a fraudulent transaction is flagged. This includes assigning cases to investigators, documenting findings, and tracking the resolution of cases.
- Feedback Loop: A feedback loop is crucial for the continuous improvement of the fraud detection system. It involves gathering feedback from investigators, analyzing false positives and false negatives, and refining the models and rules to enhance accuracy and performance.
These components work together to create a comprehensive credit card fraud detection system. By combining data collection, analysis, real-time monitoring, and investigation management, businesses can effectively detect and prevent fraudulent transactions, protecting themselves and their customers from financial loss and reputational damage.
Data Collection and Monitoring
Data collection and monitoring are critical components of a credit card fraud detection system. These processes involve gathering and analyzing data from various sources to identify patterns and anomalies indicative of fraudulent activity.
1. Data Sources: The data collection phase involves gathering transactional data from credit card transactions, including information such as transaction amount, merchant, date and time, location, and customer details. Additional data sources may include historical customer data, external data feeds from fraud databases or blacklists, and social media monitoring.
2. Data Pre-processing: Before the data can be analyzed, it needs to be cleaned and transformed into a format suitable for analysis. This process involves removing duplicates, handling missing values, and standardizing the data. Data pre-processing ensures that the data is accurate and reliable for further analysis.
3. Feature Engineering: Feature engineering involves selecting and creating relevant features from the collected data that can be used to identify fraud patterns. These features can include transaction frequency, transaction amounts, time gaps between transactions, cardholder location, and behavioral patterns. Feature engineering plays a crucial role in improving the accuracy and performance of fraud detection models.
4. Real-time Monitoring: Real-time monitoring is the constant monitoring of incoming transactions in real time. It involves analyzing transactional data against predefined rules, machine learning algorithms, or anomaly detection techniques to identify suspicious patterns or anomalies. Real-time monitoring enables immediate action to be taken when a potentially fraudulent transaction is detected.
5. Rule-based Systems: Rule-based systems apply predefined rules or thresholds to transactional data. These rules can specify conditions such as transaction amount limits, geographic restrictions, or transaction frequency thresholds. If a transaction violates any of these rules, an alert is generated for further investigation.
6. Machine Learning Algorithms: Machine learning algorithms are used to analyze transactional data and learn from historical data to detect patterns indicative of fraud. These algorithms can be supervised or unsupervised, depending on the availability of labeled training data. Commonly used machine learning algorithms for fraud detection include logistic regression, decision trees, random forests, and neural networks.
7. Anomaly Detection: Anomaly detection techniques identify deviations or outliers from normal behavior. These techniques use statistical methods or machine learning algorithms to detect transactions that deviate significantly from the expected behavior of the cardholder. Anomaly detection is particularly effective in identifying previously unseen or evolving fraud patterns.
Data collection and monitoring play a vital role in the early detection of credit card fraud. By gathering and analyzing transactional data in real-time, businesses can proactively identify and respond to potential fraudulent activities, protecting both themselves and their customers from financial loss and reputational damage.
Fraud Detection Techniques
Fraud detection is a complex task that requires the application of various techniques to identify and prevent fraudulent activities. These techniques leverage data analysis, statistical algorithms, and machine learning to detect patterns and anomalies indicative of fraud. Let’s explore some of the commonly used fraud detection techniques:
1. Rule-based System: Rule-based systems utilize predefined rules or thresholds to flag suspicious transactions. These rules can be based on transaction amount limits, geographic restrictions, or unusual spending patterns. While rule-based systems can be effective for detecting known fraud patterns, they may struggle to detect emerging or complex fraud schemes.
2. Anomaly Detection: Anomaly detection techniques aim to identify transactions or behaviors that deviate significantly from the norm. These deviations can indicate fraudulent activity. Anomaly detection can be based on statistical methods, such as clustering or density estimation, or machine learning algorithms that learn from historical data to identify abnormal patterns.
3. Supervised Machine Learning: Supervised machine learning algorithms learn from labeled training data to classify transactions as either fraudulent or non-fraudulent. These algorithms are trained on historical data, where past fraudulent transactions are labeled as such. The algorithm then predicts the likelihood of a new transaction being fraudulent based on the learned patterns.
4. Unsupervised Machine Learning: Unsupervised machine learning algorithms are used when labeled training data is scarce or unavailable. These algorithms identify patterns and anomalies in the data without prior knowledge of fraudulent transactions. Unsupervised learning can uncover previously unknown fraud patterns and adapt to evolving fraud schemes.
5. Hybrid Models: Hybrid models combine multiple fraud detection techniques to improve accuracy and reduce false positives. This approach may involve combining rule-based systems with machine learning algorithms or combining supervised and unsupervised learning methods. Hybrid models can leverage the strengths of different techniques to enhance fraud detection capabilities.
6. Behavioral Analysis: Behavioral analysis involves analyzing cardholder behavior patterns over time to detect deviations and anomalies. This technique establishes baselines for normal behavior and identifies transactions or behavioral changes that deviate from the established patterns. Behavioral analysis can help detect fraudulent activities such as account takeover or identity theft.
7. Social Network Analysis: Social network analysis examines the relationships and connections between different entities, such as cardholders, merchants, and their interactions. By analyzing transaction data and mapping social networks, this technique can identify suspicious networks or patterns of fraudulent activity.
It’s important to note that no single fraud detection technique is foolproof. Combining multiple techniques and utilizing advanced technologies such as machine learning can enhance the accuracy and effectiveness of fraud detection systems. Regularly updating and adapting these techniques to keep up with evolving fraud strategies is crucial for staying one step ahead of fraudsters.
Machine Learning Algorithms for Fraud Detection
Machine learning algorithms have revolutionized the field of fraud detection by enabling automated, data-driven approaches to identify and prevent fraudulent activities. These algorithms can effectively analyze large volumes of transactional data and learn from historical patterns to detect anomalies indicative of fraud. Let’s explore some commonly used machine learning algorithms for fraud detection:
1. Logistic Regression: Logistic regression is a popular algorithm used for binary classification problems, making it suitable for distinguishing between fraudulent and non-fraudulent transactions. It models the relationship between the transaction features and the probability of fraud. Logistic regression is relatively simple and can provide interpretable results.
2. Decision Trees: Decision trees are versatile and explainable machine learning models that can handle both categorical and numerical features. They partition the dataset based on different features, creating a tree-like structure to classify transactions as fraudulent or non-fraudulent. Decision trees are easy to interpret but prone to overfitting.
3. Random Forests: Random forests are an ensemble learning technique that combines multiple decision trees to improve accuracy and reduce overfitting. Each tree in the random forest independently classifies transactions, and the final prediction is determined through voting or averaging of individual tree predictions.
4. Support Vector Machines (SVM): SVM is a supervised learning algorithm that can separate transactions into different classes by creating an optimal hyperplane. By mapping the data into a higher-dimensional feature space, SVM can detect complex fraud patterns that are not linearly separable in the original feature space.
5. Artificial Neural Networks: Artificial neural networks (ANN) are deep learning models inspired by the human brain’s neural networks. ANNs can learn intricate patterns and relationships in transactional data, making them well-suited for fraud detection. However, ANN models can be computationally intensive and require large amounts of training data.
6. Gradient Boosting: Gradient boosting algorithms, such as XGBoost or LightGBM, iteratively train weak decision trees to minimize the prediction errors. Gradient boosting algorithms are powerful and can handle complex feature interactions, leading to improved fraud detection performance.
7. Autoencoders: Autoencoders are unsupervised learning models that aim to recreate the input data, effectively learning a compressed representation of the data. Anomalies in the data, which may indicate fraudulent transactions, result in higher reconstruction errors. Autoencoders can uncover subtle fraud patterns and adapt to changing fraud behaviors.
It’s important to note that the choice of machine learning algorithm depends on various factors, including the nature of the data, available labeled training data, computational resources, and desired performance. In practice, a combination of multiple algorithms or ensemble models is often employed to achieve optimal fraud detection accuracy and minimize false positives.
Real-time Monitoring and Alerting Systems
Real-time monitoring and alerting systems are essential components of credit card fraud detection systems. These systems continuously analyze incoming transactions in real-time, identify suspicious patterns, and generate alerts for further investigation. Let’s explore how real-time monitoring and alerting systems contribute to effective fraud detection:
1. Continuous Transaction Monitoring: Real-time monitoring systems analyze incoming transactions as they occur, allowing for immediate detection of suspicious or fraudulent activity. By continuously monitoring transactions in real-time, fraudulent activities can be identified and halted before significant financial loss occurs.
2. Rule-Based Alerts: Real-time monitoring systems can be equipped with predefined rules that trigger alerts when certain conditions are met. These rules can include transaction amount thresholds, unusual transaction locations, or multiple transactions within a short timeframe. When a transaction violates any of the rules, an alert is generated for further investigation.
3. Machine Learning-Based Anomaly Detection: Real-time monitoring systems can leverage machine learning algorithms to identify anomalies in transactional data. These algorithms learn from historical patterns and detect deviations from normal behavior. When an anomaly is detected, an alert is generated to be investigated promptly.
4. Fraud Scoring: Real-time monitoring systems assign fraud scores to transactions based on their likelihood of being fraudulent. These scores can be derived from machine learning algorithms or rule-based systems. Transactions with high fraud scores are flagged for immediate attention, enabling prompt action to prevent further fraudulent activity.
5. Threshold Adjustments: Real-time monitoring systems allow for dynamic threshold adjustments based on evolving fraud patterns. As fraudsters adapt their techniques, the system can adapt by adjusting thresholds to ensure optimal detection rates. Regular monitoring and analysis of fraud trends and historical data help in fine-tuning the thresholds.
6. Integration with Case Management Systems: Real-time monitoring and alerting systems are seamlessly integrated with case management systems. When an alert is generated, relevant information about the flagged transaction is automatically transferred to the case management system for further investigation. This streamlined process ensures efficient handling of potential fraud cases.
7. Real-time Reporting and Dashboards: Real-time monitoring systems provide real-time reporting and dashboards that allow fraud analysts and investigators to track and visualize fraud trends. These reports and dashboards provide valuable insights into the overall fraud landscape, helping organizations make informed decisions to enhance fraud prevention.
By incorporating real-time monitoring and alerting systems into credit card fraud detection, financial institutions and businesses can proactively detect and respond to potential fraudulent activities. These systems provide timely alerts, enabling swift action to minimize financial losses, protect customers, and maintain the integrity of the financial ecosystem.
Case Studies of Successful Credit Card Fraud Detection Systems
Implementing effective credit card fraud detection systems is crucial for businesses and financial institutions to protect themselves and their customers from fraudulent activities. Let’s explore some case studies highlighting successful credit card fraud detection systems:
1. Case Study 1: PayPal: PayPal, a leading online payment platform, employs a robust fraud detection system that combines rule-based systems, anomaly detection algorithms, and machine learning models. By continuously analyzing transactional data in real-time, PayPal’s fraud detection system can identify abnormalities, detect fraudulent activity, and block suspicious transactions. This system has enabled PayPal to reduce fraudulent losses, enhance customer trust, and maintain a secure payment environment.
2. Case Study 2: JPMorgan Chase: JPMorgan Chase, a global financial services firm, has developed a sophisticated fraud detection system called “Falcon.” Falcon utilizes advanced machine learning algorithms to analyze patterns and behaviors across their vast network of credit card transactions. By detecting anomalies and suspicious activities, Falcon can quickly identify potential fraud and take immediate action to protect their customers. This system has significantly reduced fraud losses for JPMorgan Chase and has become an industry benchmark for effective fraud detection.
3. Case Study 3: Amazon: Amazon, the e-commerce giant, employs a powerful fraud detection system to protect customers and sellers on its platform. By leveraging machine learning algorithms and data analysis, Amazon’s system can analyze vast amounts of transactional data, customer profiles, and browsing patterns to detect fraudulent behaviors and transactions. This system allows Amazon to take proactive measures, such as blocking suspicious accounts and transactions, to minimize fraudulent activity and maintain a secure marketplace.
4. Case Study 4: American Express: American Express utilizes a comprehensive fraud detection system called “Advanced Authorization.” This system combines advanced machine learning algorithms with real-time monitoring to identify potential fraudulent transactions. American Express’s system analyzes various data points, including transaction history, customer spending patterns, and location data, to assess the risk level of a transaction. This system has successfully reduced fraud losses and improved the overall security of their cardholders’ accounts.
These case studies demonstrate the effectiveness of credit card fraud detection systems in safeguarding financial transactions. By implementing sophisticated technologies, leveraging data analysis, and utilizing machine learning algorithms, these companies have been able to stay ahead of fraudsters and protect their customers from financial losses and potential data breaches. The success of these systems serves as a testament to the importance of investing in robust fraud detection mechanisms to ensure secure and trustworthy financial transactions.
Challenges and Limitations of Credit Card Fraud Detection Systems
While credit card fraud detection systems have proven to be effective in combating fraudulent activities, they are not without challenges and limitations. Understanding these challenges is crucial for developing robust and adaptive fraud detection mechanisms. Let’s explore some of the common challenges and limitations:
1. Emerging Fraud Schemes: Fraudsters are constantly evolving their techniques to evade detection. As new fraud schemes emerge, fraud detection systems may struggle to detect and adapt to these evolving patterns. Continuous monitoring, regular updates to detection algorithms, and staying informed about the latest fraud trends are essential to tackle emerging fraud schemes.
2. False Positives and False Negatives: Fraud detection systems often face the challenge of balancing between accurately identifying fraudulent transactions and avoiding false positives or false negatives. False positives occur when legitimate transactions are mistakenly flagged as fraudulent, leading to inconvenience for customers. False negatives, on the other hand, occur when fraudulent transactions go undetected. Striking the right balance requires fine-tuning detection algorithms and minimizing the impact on genuine transactions.
3. Data Quality and Volume: The effectiveness of a fraud detection system heavily relies on the quality and volume of the data used for analysis. Incomplete or erroneous data can hinder the accuracy of the system. Moreover, as the volume of transactions increases, the system may struggle to process and analyze data in real-time, leading to delayed detection or increased false positives.
4. Imbalanced Data: Imbalanced data, where the number of non-fraudulent transactions far exceeds the fraudulent ones, poses a challenge for machine learning algorithms. The algorithms may be biased towards the majority class, resulting in suboptimal fraud detection. Techniques such as oversampling the minority class, undersampling the majority class, or using ensemble models can help address this challenge.
5. Privacy Concerns: Fraud detection systems need access to sensitive customer data to accurately analyze transactions. However, this raises privacy concerns and requires strict adherence to data protection regulations. Implementing robust security measures, anonymizing data where possible, and ensuring compliance with privacy laws are essential to address these concerns.
6. Complexity and Cost: Developing and maintaining effective fraud detection systems can be complex and resource-intensive. Implementing advanced analytics tools, hiring skilled analysts, and regularly updating the system require significant investments. Moreover, the cost of false positives, including customer dissatisfaction and potential revenue loss, should be carefully managed.
Overcoming these challenges requires a multi-faceted approach that involves continuous research and development, collaboration between industry stakeholders, and leveraging advanced technologies and data analysis techniques. By addressing these challenges and limitations, businesses and financial institutions can enhance the effectiveness of their credit card fraud detection systems and adapt to the ever-evolving landscape of fraudulent activities.
Future Trends in Credit Card Fraud Detection
The field of credit card fraud detection is constantly evolving to keep pace with the advancements in technology and the changing landscape of fraud. Several emerging trends show promise in enhancing the effectiveness of credit card fraud detection systems. Let’s explore some of these future trends:
1. Artificial Intelligence and Machine Learning: Artificial intelligence (AI) and machine learning (ML) will continue to play a significant role in fraud detection. Advanced ML algorithms can analyze large volumes of transactional data in real-time, improving the accuracy of fraud detection and reducing false positives. The integration of AI technologies, such as natural language processing and deep learning, will further enhance the ability to detect complex fraud patterns.
2. Behavioral Biometrics: Behavioral biometrics, such as fingerprint recognition, voice patterns, and keystroke dynamics, provide unique identifiers for individuals. By analyzing behavioral characteristics, fraud detection systems can authenticate users more securely and detect anomalies that may indicate fraud. These biometrics can add an extra layer of security to prevent account takeover and identity theft.
3. Real-time Data Streaming: Real-time data streaming technologies enable the analysis of data as it is generated, allowing for immediate detection of fraudulent activities. By leveraging streaming analytics platforms, fraud detection systems can process large volumes of data in real-time, enabling faster and more accurate fraud detection.
4. Social Media Analysis: Social media platforms are a treasure trove of information that can provide valuable insights into customer behavior. Integrating social media analysis into fraud detection systems can help identify connections between fraudsters, uncover hidden networks involved in fraudulent activities, and detect potential fraudulent behaviors based on social media posts or interactions.
5. Blockchain Technology: Blockchain technology offers transparent and immutable transaction records, making it an attractive solution for enhancing transparency and security in payment systems. By leveraging blockchain, fraud detection systems can verify the authenticity of transactions and prevent tampering with transactional data, reducing the risk of fraud.
6. Collaborative Fraud Detection: Collaboration between financial institutions, merchants, and payment processors can greatly enhance fraud detection. Shared databases, information sharing platforms, and collaborative analytics can enable the identification of patterns and fraud trends that span across different entities and industries, leading to more effective fraud prevention.
7. Enhanced Customer Authentication: Implementing stronger customer authentication methods, such as multi-factor authentication and biometrics, can significantly reduce the risk of fraud. Techniques like facial recognition, voice recognition, and fingerprint scanning can add an extra layer of security, making it harder for fraudsters to impersonate legitimate customers.
As technology continues to advance, credit card fraud detection systems will need to adapt and leverage these future trends to stay ahead of fraudsters. By embracing emerging technologies, collaborating with industry stakeholders, and continuously enhancing data analysis capabilities, businesses and financial institutions can build robust fraud detection systems that provide effective protection against evolving fraudulent activities.
Conclusion
In a world driven by digital transactions, credit card fraud has become a prevalent and ever-evolving threat. However, the development of sophisticated credit card fraud detection systems has provided a crucial line of defense for businesses and financial institutions. These systems employ advanced technologies, data analysis techniques, and machine learning algorithms to identify and prevent fraudulent activities in real-time.
From understanding the different types of credit card fraud to exploring the components and techniques used in fraud detection systems, it is evident that these systems play a vital role in protecting consumers and businesses alike. By continuously monitoring and analyzing transactional data, these systems can detect suspicious patterns, identify anomalies, and generate alerts for further investigation. This proactive approach helps prevent financial loss, minimize the impact on customers, and maintain the integrity of the payment ecosystem.
While credit card fraud detection systems have proven their effectiveness, they do face challenges and limitations. The rise of emerging fraud schemes, the need to balance accuracy with avoiding false positives and false negatives, and the importance of data quality and privacy are challenges that must be addressed to ensure the ongoing success of these systems.
Looking towards the future, there are exciting trends on the horizon that hold great potential for enhancing credit card fraud detection. Artificial intelligence, behavioral biometrics, real-time data streaming, social media analysis, blockchain technology, collaborative approaches, and enhanced customer authentication are among the trends that will shape the future of fraud detection. Through the adoption of these trends, businesses and financial institutions can strengthen their fraud prevention efforts and stay one step ahead of fraudsters.
In conclusion, credit card fraud detection systems are indispensable in today’s digital landscape. Their role in protecting financial transactions, safeguarding customer data, and maintaining trust between businesses and consumers cannot be overstated. By investing in robust systems, staying updated with emerging trends, and fostering collaboration, organizations can effectively combat credit card fraud and ensure secure and seamless transactions for all.