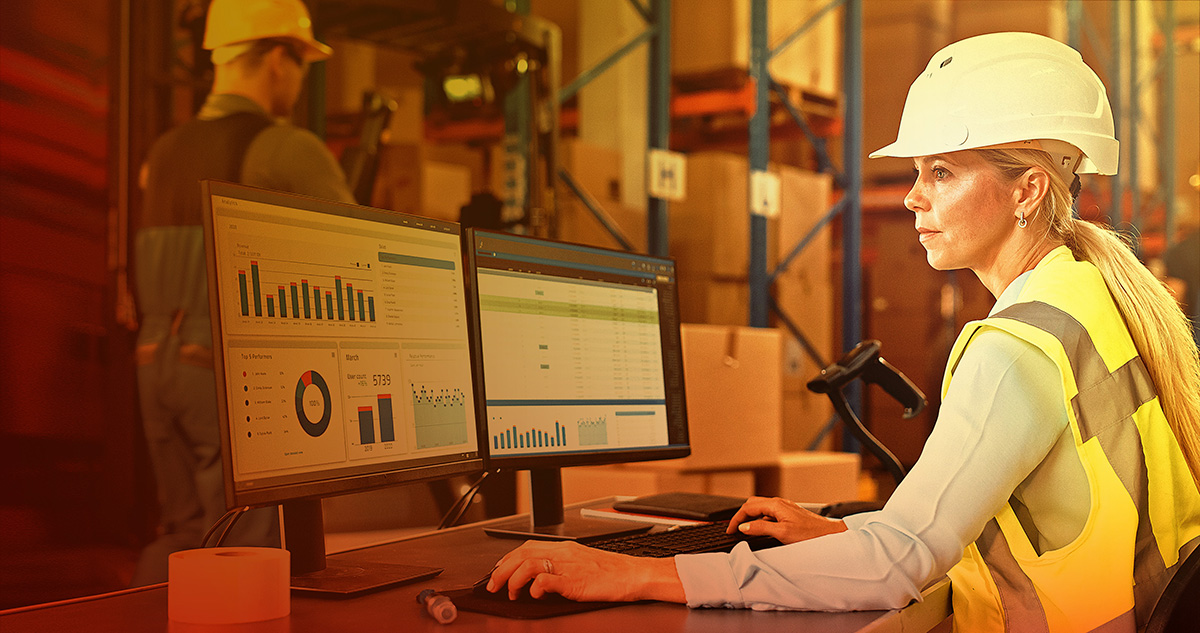
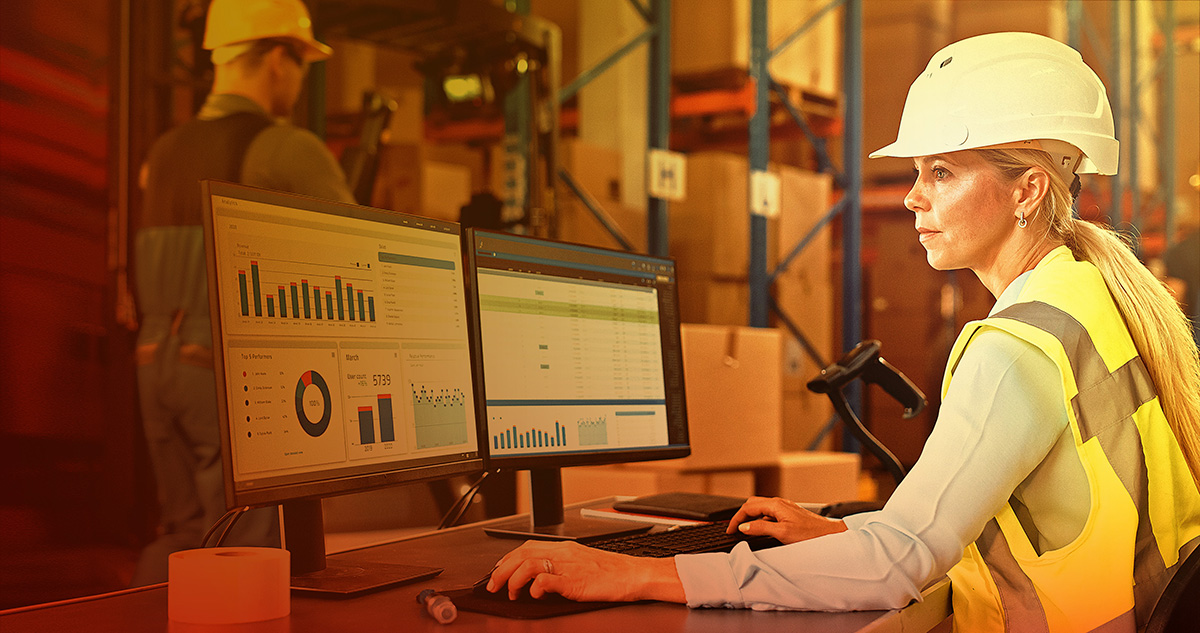
Finance
What Is Supply Chain Analytics?
Modified: February 21, 2024
Discover the power of supply chain analytics in finance. Unlock valuable insights and optimize operations with data-driven decision making.
(Many of the links in this article redirect to a specific reviewed product. Your purchase of these products through affiliate links helps to generate commission for LiveWell, at no extra cost. Learn more)
Table of Contents
- Introduction
- Definition of Supply Chain Analytics
- Importance of Supply Chain Analytics
- Benefits of Supply Chain Analytics
- Key Components of Supply Chain Analytics
- Techniques Used in Supply Chain Analytics
- Challenges in Implementing Supply Chain Analytics
- Applications of Supply Chain Analytics
- Case Studies on Supply Chain Analytics
- Future Trends in Supply Chain Analytics
- Conclusion
Introduction
Supply chain management is a critical function that involves the coordination of multiple processes, from procurement and production to inventory management and distribution. In today’s fast-paced and competitive business landscape, organizations need effective strategies to optimize their supply chains and gain a competitive edge. This is where supply chain analytics comes into play.
Supply chain analytics is the process of collecting, analyzing, and leveraging data to improve supply chain performance and decision-making. By harnessing the power of data analytics, organizations can gain valuable insights into their supply chain operations, identify bottlenecks, and optimize processes, ultimately driving efficiency and enhancing customer satisfaction.
At its core, supply chain analytics combines the principles of supply chain management with advanced analytical techniques. It involves the use of statistical analysis, machine learning, optimization models, and predictive modeling to uncover patterns, trends, and anomalies within the supply chain data.
In recent years, the increasing availability of data and advancements in technology have fueled the growth of supply chain analytics. Organizations now have access to higher volumes of data, generated from various sources such as logistics systems, point-of-sale terminals, and IoT devices. This data provides a wealth of information that can be used to gain deeper visibility into the supply chain and make data-driven decisions.
The main objective of supply chain analytics is to enable organizations to make proactive and informed decisions in real-time. By analyzing historical and real-time data, organizations can optimize inventory levels, minimize stockouts, reduce lead times, and improve forecasting accuracy. Additionally, supply chain analytics can help identify potential risks and disruptions, allowing organizations to take preemptive action and mitigate potential setbacks.
In the following sections, we will delve deeper into the definition, importance, benefits, key components, techniques, challenges, applications, case studies, and future trends of supply chain analytics. By examining these aspects, we can gain a comprehensive understanding of how organizations utilize supply chain analytics to drive operational excellence and achieve strategic objectives.
Definition of Supply Chain Analytics
Supply chain analytics refers to the use of data analysis techniques and statistical modeling to gain insights into supply chain operations, identify patterns, and drive informed decision-making. It involves collecting and analyzing data from various sources within the supply chain, including suppliers, manufacturers, distributors, and customers.
At its core, supply chain analytics aims to improve the efficiency, effectiveness, and overall performance of the supply chain. It provides organizations with valuable insights and actionable recommendations to optimize processes, enhance inventory management, reduce costs, and improve customer satisfaction.
Supply chain analytics encompasses a wide range of analytical techniques and tools. These include descriptive analytics, which helps understand past performance and historical trends, diagnostic analytics, which identifies the root cause of supply chain issues, predictive analytics, which forecasts future outcomes, and prescriptive analytics, which recommends the best course of action.
Some key components of supply chain analytics include data collection, data cleansing and integration, data analysis and modeling, and data visualization. Organizations need to have robust data infrastructure and systems in place to effectively collect and analyze relevant data. This data can come from a variety of sources, such as enterprise resource planning (ERP) systems, transportation management systems (TMS), warehouse management systems (WMS), and sensors and IoT devices.
Supply chain analytics leverages advanced analytical techniques, such as machine learning, optimization modeling, simulation, and network analysis, to uncover insights from the vast amount of data generated by the supply chain. These techniques help identify patterns, optimize inventory levels, forecast demand accurately, minimize supply chain disruptions, and improve overall operational efficiency.
In summary, supply chain analytics is an essential tool for organizations looking to gain a competitive advantage in today’s complex and dynamic business environment. By leveraging the power of data analytics, organizations can make more informed and proactive decisions, streamline processes, and achieve better supply chain visibility and agility. With the increasing availability of data and advancements in analytics technology, supply chain analytics is set to play a crucial role in driving operational excellence and ensuring business success.
Importance of Supply Chain Analytics
Supply chain analytics plays a crucial role in today’s business landscape, where organizations are faced with complex supply chain networks, demanding customers, and intense competition. Here are some key reasons why supply chain analytics is important:
- Optimizing Efficiency: Supply chain analytics enables organizations to identify inefficiencies and bottlenecks within their supply chain processes. By analyzing data and uncovering insights, organizations can streamline operations, reduce lead times, minimize stockouts, and improve overall efficiency.
- Enhancing Customer Satisfaction: Supply chain analytics empowers organizations to better understand customer demands and expectations. By analyzing customer data, organizations can improve demand forecasting, optimize inventory levels, and ensure timely delivery of products, resulting in higher customer satisfaction and loyalty.
- Making Informed Decisions: Supply chain analytics provides organizations with real-time insights into supply chain performance. By monitoring key metrics and analyzing trends, organizations can make data-driven decisions to address issues, mitigate risks, and capitalize on opportunities.
- Mitigating Risks: Supply chain analytics helps organizations identify potential risks and disruptions within the supply chain. By analyzing historical and real-time data, organizations can anticipate and proactively respond to supply chain disruptions, such as demand fluctuations, supplier issues, or natural disasters, minimizing the impact on operations and ensuring continuity.
- Reducing Costs: Supply chain analytics enables organizations to identify cost-saving opportunities. By analyzing data and optimizing processes, organizations can reduce transportation costs, optimize inventory levels, minimize waste and inefficiencies, and negotiate better terms with suppliers, resulting in significant cost savings.
- Improving Collaboration: Supply chain analytics facilitates collaboration and information sharing across supply chain partners. By analyzing and sharing relevant data, organizations can build stronger relationships with suppliers and customers, enhance communication, and improve overall supply chain visibility and coordination.
Overall, supply chain analytics is crucial for organizations seeking to enhance their operational efficiency, customer satisfaction, and competitive advantage. By leveraging data-driven insights, organizations can make informed decisions, streamline processes, mitigate risks, and drive continuous improvement within their supply chains.
Benefits of Supply Chain Analytics
Supply chain analytics offers numerous benefits to organizations across various industries. By harnessing the power of data and analytics, organizations can optimize their supply chain operations and drive strategic value. Here are some key benefits of supply chain analytics:
- Improved Decision-Making: Supply chain analytics provides organizations with valuable insights and actionable recommendations, enabling them to make informed and data-driven decisions. By analyzing historical and real-time data, organizations can identify trends, patterns, and relationships within the supply chain, helping them make better decisions on inventory management, procurement, production, and distribution.
- Enhanced Forecasting Accuracy: Accurate demand forecasting is essential for optimizing inventory levels, reducing stockouts, and improving customer satisfaction. Supply chain analytics leverages advanced forecasting models and techniques, such as machine learning and time series analysis, to improve the accuracy of demand forecasts. This enables organizations to align their supply with customer demand, ensuring the right products are available at the right time.
- Optimized Inventory Management: Excessive inventory levels can tie up working capital and lead to increased carrying costs, while inadequate inventory levels can result in stockouts and lost sales opportunities. Supply chain analytics helps organizations optimize their inventory by analyzing demand patterns, lead times, and historical data. By accurately predicting demand and optimizing reorder points, organizations can reduce inventory holding costs while maintaining high service levels.
- Cost Savings: Supply chain analytics identifies cost-saving opportunities within the supply chain. By analyzing data on transportation, procurement, and production, organizations can identify inefficiencies, bottlenecks, and areas for improvement. This enables them to streamline processes, negotiate better terms with suppliers, optimize transportation routes, and reduce overall supply chain costs.
- Improved Supply Chain Visibility: Supply chain analytics provides organizations with real-time visibility into their supply chain operations. By integrating data from various sources, such as ERP systems, TMS, and WMS, organizations can obtain a holistic view of their supply chain performance. This visibility enables proactive monitoring of key metrics, identification of issues, and timely intervention to prevent disruptions and improve overall supply chain performance.
- Enhanced Collaboration and Communication: Effective collaboration and communication are essential for a successful and responsive supply chain. Supply chain analytics facilitates data sharing and collaboration among supply chain partners. By sharing relevant data and insights, organizations can improve coordination, reduce lead times, and enhance overall supply chain efficiency.
- Risk Mitigation: Supply chain analytics helps organizations identify potential risks and disruptions within the supply chain. By analyzing historical and real-time data, organizations can predict and mitigate risks associated with demand variability, supplier disruptions, and transportation delays. This enables proactive risk management and ensures business continuity.
In summary, supply chain analytics offers a wide range of benefits, including improved decision-making, enhanced forecasting accuracy, optimized inventory management, cost savings, improved visibility, better collaboration, and risk mitigation. By leveraging these benefits, organizations can achieve operational excellence, deliver better customer satisfaction, and gain a competitive advantage in today’s dynamic business environment.
Key Components of Supply Chain Analytics
Supply chain analytics combines various components to analyze and utilize data effectively for supply chain optimization. These key components work together to provide organizations with valuable insights and actionable recommendations. Here are the main components of supply chain analytics:
- Data Collection: The foundation of supply chain analytics is data collection. Organizations gather data from various sources within the supply chain, including internal systems such as ERP, TMS, and WMS, as well as external sources like customer feedback, market trends, and social media. This data includes information about demand patterns, inventory levels, order fulfillment, transportation, and more.
- Data Cleansing and Integration: Once data is collected, it needs to be cleansed and integrated to ensure accuracy and consistency. Data cleansing involves removing errors, duplicate entries, and inconsistencies. Data integration involves combining data from multiple sources into a unified and usable format. This process ensures that the data used for analysis is reliable and consistent.
- Data Analysis and Modeling: The next step in supply chain analytics is analyzing the collected data using various analytical techniques. This involves applying statistical analysis, machine learning, optimization models, simulation, and other methodologies to uncover patterns, trends, and relationships within the data. Data modeling helps organizations understand how different factors and variables impact the supply chain performance and enables scenario planning and predictive analysis.
- Data Visualization: Supply chain analytics utilizes data visualization techniques to present complex information in a visual and easily understandable format. This includes charts, graphs, dashboards, and interactive reports. Data visualization helps stakeholders, decision-makers, and supply chain professionals quickly grasp insights and make informed decisions based on the analyzed data.
- Performance Metrics: Key performance indicators (KPIs) play a vital role in supply chain analytics. Organizations define and track performance metrics such as order fill rate, on-time delivery, inventory turnover, and supply chain costs. These metrics provide a quantitative measure of supply chain performance and serve as benchmarks for improvement.
- Advanced Analytics Techniques: Supply chain analytics leverages advanced analytical techniques to gain deeper insights and solve complex supply chain problems. Machine learning algorithms can predict demand patterns, optimize inventory levels, and detect anomalies. Optimization models can optimize transportation routes and production schedules. Simulation models can help simulate different scenarios and identify the best course of action.
- Real-Time Monitoring and Alerts: Supply chain analytics involves real-time monitoring of supply chain activities and the implementation of automated alerts. This allows organizations to quickly identify deviations, disruptions, and potential issues. Real-time monitoring helps organizations proactively manage the supply chain, mitigate risks, and take timely corrective actions.
By integrating these key components, organizations can harness the power of supply chain analytics to gain insights, optimize operations, make data-driven decisions, and drive continuous improvement in their supply chain performance.
Techniques Used in Supply Chain Analytics
Supply chain analytics utilizes a variety of techniques and methodologies to analyze data, uncover insights, and optimize supply chain operations. These techniques help organizations make data-driven decisions and improve overall supply chain performance. Here are some commonly used techniques in supply chain analytics:
- Descriptive Analytics: Descriptive analytics involves analyzing historical data to understand past trends and patterns. It helps organizations gain visibility into supply chain performance and identify areas for improvement. Descriptive analytics techniques include data aggregation, data visualization, and basic statistical analysis.
- Diagnostic Analytics: Diagnostic analytics focuses on understanding the root causes of supply chain issues and identifying the reasons behind certain outcomes. It involves analyzing data to uncover relationships and correlations between different variables. Techniques used in diagnostic analytics include regression analysis, correlation analysis, and root cause analysis. Diagnostic analytics helps organizations identify bottlenecks, inefficiencies, and areas for process improvement.
- Predictive Analytics: Predictive analytics uses historical and real-time data to forecast future outcomes and trends. Machine learning algorithms are commonly employed in predictive analytics to analyze historical data and make predictions based on patterns and trends. By accurately predicting demand, lead times, and other key factors, organizations can optimize inventory levels, plan production schedules, and minimize stockouts or excess inventory.
- Prescriptive Analytics: Prescriptive analytics takes predictive analytics a step further by recommending the best course of action to optimize supply chain decisions. It combines historical data, predictive models, optimization algorithms, and business rules to generate actionable recommendations. Prescriptive analytics helps organizations make proactive decisions that optimize inventory levels, distribution routes, production plans, and other supply chain parameters.
- Simulation Modeling: Simulation modeling involves creating virtual models of the supply chain to simulate different scenarios and evaluate the impact of potential changes or disruptions. By simulating various “what-if” scenarios, organizations can assess the impact on key performance indicators such as customer service levels, inventory costs, and transportation efficiency. Simulation modeling enables proactive decision-making and risk mitigation.
- Optimization Modeling: Optimization modeling uses mathematical and algorithmic techniques to optimize supply chain decisions. It involves defining objectives, constraints, and decision variables and finding the best solution that maximizes or minimizes a specific key performance indicator. Optimization modeling can be applied to various supply chain areas, such as production planning, inventory management, transportation routing, and supplier selection.
- Machine Learning: Machine learning algorithms play a crucial role in supply chain analytics. By training models on historical data, machine learning can identify patterns, trends, and anomalies within the supply chain data. Machine learning techniques include regression analysis, classification algorithms, cluster analysis, and neural networks. Machine learning helps organizations improve demand forecasting, optimize inventory levels, and detect fraud or anomalies in supply chain processes.
These techniques, when applied effectively, provide organizations with valuable insights, optimize supply chain decisions, and drive continuous improvement in supply chain operations. By leveraging these advanced analytical techniques, organizations can gain a competitive advantage, enhance customer satisfaction, and achieve operational excellence in their supply chain management.
Challenges in Implementing Supply Chain Analytics
While supply chain analytics offers significant benefits to organizations, there are several challenges that can hinder its successful implementation. These challenges arise from various factors, including data quality, technology limitations, organizational barriers, and resource constraints. Here are some common challenges in implementing supply chain analytics:
- Data Quality and Availability: One of the primary challenges in supply chain analytics is the quality and availability of data. Supply chain data comes from various sources and systems, and ensuring its accuracy, completeness, and consistency can be a complex task. Poor data quality, incomplete or inconsistent data, and data silos can lead to inaccurate analysis and unreliable insights, hampering the effectiveness of supply chain analytics initiatives.
- Data Integration: Integrating data from different sources within the supply chain can be a complex and time-consuming process. Data integration involves harmonizing data formats, resolving inconsistencies, and merging data from various systems. Lack of data integration capabilities can impede the seamless flow of information and hinder the ability to analyze the entire supply chain, limiting the effectiveness of supply chain analytics.
- Technology Limitations: Supply chain analytics relies heavily on technology infrastructure and tools. Organizations need robust analytics platforms, data warehouses, visualization tools, and advanced analytics capabilities to support supply chain analytics initiatives. However, limitations in technology infrastructure, outdated systems, and limited IT resources can pose challenges in implementing and scaling up supply chain analytics capabilities.
- Organizational Culture and Change Management: Implementing supply chain analytics requires a shift in organizational culture and mindset. It may involve adopting new processes, embracing analytics-driven decision-making, and breaking down silos between departments. Resistance to change and lack of buy-in from key stakeholders can hinder the successful implementation of supply chain analytics initiatives.
- Skills and Talent Gap: Supply chain analytics requires skilled professionals with expertise in data analysis, statistical modeling, and advanced analytics techniques. However, there is often a shortage of professionals with the necessary skills and knowledge to effectively leverage supply chain analytics. Organizations may need to invest in training and development programs to overcome the skills and talent gap in supply chain analytics.
- Cost and Resource Constraints: Implementing and maintaining supply chain analytics capabilities can be a costly endeavor. It requires investments in technology infrastructure, analytics tools, data storage, and talent. Limited budget and resource constraints may pose challenges for organizations, particularly for smaller companies with limited financial resources and manpower.
- Data Security and Privacy: With the increasing use of data in supply chain analytics, organizations must ensure the security and privacy of sensitive information. Protecting data from unauthorized access, maintaining data integrity, and complying with data protection regulations present significant challenges. Organizations need robust data security measures and compliance frameworks to address these concerns and ensure the confidentiality and privacy of supply chain data.
Despite these challenges, organizations can overcome them through careful planning, investment in technology and talent, data governance strategies, and organizational change management. By addressing these challenges, organizations can unlock the full potential of supply chain analytics and gain a competitive advantage in today’s dynamic business environment.
Applications of Supply Chain Analytics
Supply chain analytics has a wide range of applications across various aspects of supply chain management. By harnessing the power of data analytics and advanced techniques, organizations can streamline their operations, improve decision-making, and drive continuous improvement. Here are some key applications of supply chain analytics:
- Demand Forecasting: Supply chain analytics aids organizations in accurate demand forecasting. By analyzing historical sales data, market trends, and external factors, organizations can generate reliable demand forecasts. These forecasts enable better inventory planning, production scheduling, and procurement decisions, preventing stockouts or excess inventory.
- Inventory Optimization: Supply chain analytics helps organizations strike the right balance between inventory levels and customer service. By analyzing demand patterns, lead times, and market dynamics, organizations can optimize inventory levels, reduce carrying costs, and improve order fulfillment rates.
- Supplier Performance Management: Supply chain analytics provides organizations with insights into supplier performance. By analyzing data on delivery times, quality, and cost, organizations can evaluate supplier performance and make informed decisions on supplier selection, negotiation, and relationship management.
- Transportation Optimization: Supply chain analytics optimizes transportation planning and routing. By analyzing data on shipment volumes, transportation costs, and delivery locations, organizations can identify the most efficient routes, consolidate shipments, and reduce transportation costs.
- Warehouse Operations Optimization: Supply chain analytics improves warehouse operations efficiency. By analyzing data on inventory levels, order volumes, and fulfillment rates, organizations can optimize warehouse layout, storage allocation, and picking processes. This reduces lead times, improves order accuracy, and maximizes space utilization.
- Supply Chain Risk Management: Supply chain analytics helps organizations identify and mitigate risks within the supply chain. By analyzing data on supplier performance, demand variability, and external factors, organizations can proactively identify and mitigate risks, such as supply disruptions, demand fluctuations, and regulatory changes.
- Sustainability and Environmental Impact: Supply chain analytics enables organizations to measure and improve their environmental sustainability. By analyzing data on carbon emissions, energy usage, and waste generation, organizations can identify areas for improvement, optimize transportation routes, and reduce their environmental footprint.
- Customer Segmentation and Satisfaction: Supply chain analytics aids organizations in understanding customer behavior and preferences. By analyzing customer data, such as purchase history, browsing patterns, and feedback, organizations can segment customers and personalize their offerings. This leads to enhanced customer satisfaction, higher customer retention rates, and increased sales.
These are just a few examples of the many applications of supply chain analytics. The versatility and wide-ranging impact of supply chain analytics make it a valuable tool for organizations seeking to optimize their supply chain operations, enhance customer satisfaction, and gain a competitive advantage in the marketplace.
Case Studies on Supply Chain Analytics
Case studies provide real-life examples of how organizations have successfully implemented supply chain analytics to drive improvements and achieve tangible results. Here are a few notable case studies highlighting the application and benefits of supply chain analytics:
-
Case Study 1: Walmart’s Demand Sensing Analytics
Walmart, a global retail giant, implemented demand sensing analytics to improve its inventory management and reduce stockouts. By analyzing various data sources such as sales data, point-of-sale data, and weather data, Walmart effectively forecasted customer demand at the store level. This enabled the company to optimize inventory levels across its vast supply chain, reducing inventory carrying costs and improving customer service levels. -
Case Study 2: Amazon’s Predictive Analytics for Supply Chain Optimization
Amazon, an industry leader in e-commerce, utilizes predictive analytics to optimize its supply chain operations. By analyzing vast amounts of customer data, order history, and market trends, Amazon accurately predicts customer demand and optimizes its inventory allocation and fulfillment network. This enables Amazon to deliver products faster, reduce transportation costs, and maximize customer satisfaction. -
Case Study 3: Procter & Gamble’s Supplier Risk Predictive Analytics
Procter & Gamble (P&G), a multinational consumer goods company, implemented predictive analytics to manage supplier risks effectively. P&G analyzed various data sources, including supplier performance data, market trends, and macroeconomic indicators. By predicting supplier risks and disruptions, P&G proactively engages with suppliers and takes preventive measures to ensure the continuity of its supply chain, minimizing the impact of potential disruptions. -
Case Study 4: UPS’s Routing Optimization Analytics
UPS, a global logistics company, employs routing optimization analytics to optimize package delivery routes and reduce transportation costs. By analyzing data on package weight, destination, traffic patterns, and delivery time windows, UPS can optimize its delivery routes, minimize mileage, and improve fuel efficiency. This allows UPS to deliver packages faster while reducing its carbon footprint. -
Case Study 5: Coca-Cola’s Sales and Operations Planning Analytics
Coca-Cola, a leading beverage company, utilizes sales and operations planning analytics to align its demand and supply planning processes. By analyzing historical and real-time data, including sales data, marketing campaigns, and production capabilities, Coca-Cola can forecast demand accurately and align its production, distribution, and inventory strategies accordingly. This improves overall supply chain efficiency and customer satisfaction.
These case studies demonstrate the benefits and effectiveness of supply chain analytics in driving improvements across various aspects of the supply chain. From inventory management and supplier risk mitigation to routing optimization and sales planning, organizations leverage supply chain analytics to enhance operational efficiency, reduce costs, and improve customer satisfaction.
Future Trends in Supply Chain Analytics
As technology continues to advance and organizations strive for greater supply chain optimization, several emerging trends are shaping the future of supply chain analytics:
- Internet of Things (IoT): The proliferation of IoT devices is generating massive amounts of data across the supply chain. IoT devices embedded in products, vehicles, warehouses, and equipment can provide real-time data on parameters such as temperature, humidity, location, and performance. Supply chain analytics will increasingly leverage this IoT-generated data to gain deeper insights, improve tracking and traceability, and enhance decision-making.
- Artificial Intelligence (AI) and Machine Learning (ML): AI and ML algorithms are revolutionizing supply chain analytics. These technologies enable self-learning systems that can continuously improve forecasting accuracy, optimize operations, and automatically detect anomalies or patterns that humans might miss. AI-powered supply chain analytics can help organizations make more proactive, data-driven decisions, automate routine tasks, and drive improved efficiency and responsiveness.
- Blockchain Technology: Blockchain technology offers enhanced transparency, security, and traceability in supply chain operations. By leveraging blockchain, supply chain analytics can enable end-to-end visibility of transactions, improve supply chain collaboration, and ensure the authenticity and integrity of information. Blockchain can provide a single source of truth for supply chain data, reducing disputes and enabling trust between supply chain partners.
- Predictive and Prescriptive Analytics Maturity: Predictive and prescriptive analytics will continue to advance, enabling more accurate and insightful decision-making. With the integration of real-time data, organizations can enhance demand forecasting, optimize inventory levels, automate decision-making, and proactively mitigate risks. More sophisticated optimization models and scenario planning capabilities will empower organizations to make strategic decisions with greater confidence.
- Big Data and Advanced Analytics: With the exponential growth of data, supply chain analytics will focus on managing, processing, and deriving insights from big data. Advanced analytics techniques, including natural language processing, sentiment analysis, and social media analytics, will enable organizations to tap into unstructured data sources to gain a holistic view of the supply chain. This data-driven approach will drive more accurate demand forecasting, improved customer segmentation, and better supply chain risk management.
- Supply Chain Integration and Collaboration: Supply chain analytics will increasingly foster collaboration and integration among supply chain partners. By sharing data and insights, organizations can achieve greater visibility, coordination, and responsiveness across the supply chain. This collaboration will lead to improved demand sensing, faster response to market changes, and more efficient allocation of resources.
These future trends in supply chain analytics hold significant potential to transform supply chain management. By leveraging IoT, AI, blockchain, and advanced analytics, organizations can make more informed decisions, optimize operations, improve customer satisfaction, and drive sustainable growth in an increasingly complex and interconnected business landscape.
Conclusion
Supply chain analytics has emerged as a powerful tool for organizations seeking to optimize their supply chain operations, enhance customer satisfaction, and gain a competitive edge. By harnessing the power of data analytics, organizations can unlock valuable insights, make data-driven decisions, and drive continuous improvement within their supply chains.
Supply chain analytics enables organizations to effectively analyze historical and real-time data, uncover patterns and trends, and identify opportunities for enhancement. With techniques such as descriptive, diagnostic, predictive, and prescriptive analytics, organizations can gain visibility into their supply chain, optimize inventory levels, streamline transportation routes, mitigate risks, and improve overall operational efficiency.
The benefits of supply chain analytics are far-reaching. From improving decision-making to enhancing forecasting accuracy, reducing costs, and enhancing customer satisfaction, organizations can achieve significant advantages by utilizing supply chain analytics. Furthermore, the emergence of technologies such as IoT, AI, and blockchain, along with the increasing availability of big data, presents even greater opportunities for supply chain analytics in the future.
However, implementing supply chain analytics does come with challenges. Organizations must address data quality, integration, and technology limitations. They must also navigate organizational changes, skill gaps, and resource constraints. Overcoming these challenges requires careful planning, investment in technology and talent, and the development of a data-driven culture.
In conclusion, supply chain analytics is a critical component for organizations looking to thrive in the modern business landscape. By leveraging data and advanced analytics techniques, organizations can optimize supply chain performance, drive efficiency and cost savings, mitigate risks, and deliver enhanced customer experiences. As technology continues to advance and organizations embrace a more data-driven approach, supply chain analytics will remain integral to achieving operational excellence and maintaining a competitive edge.