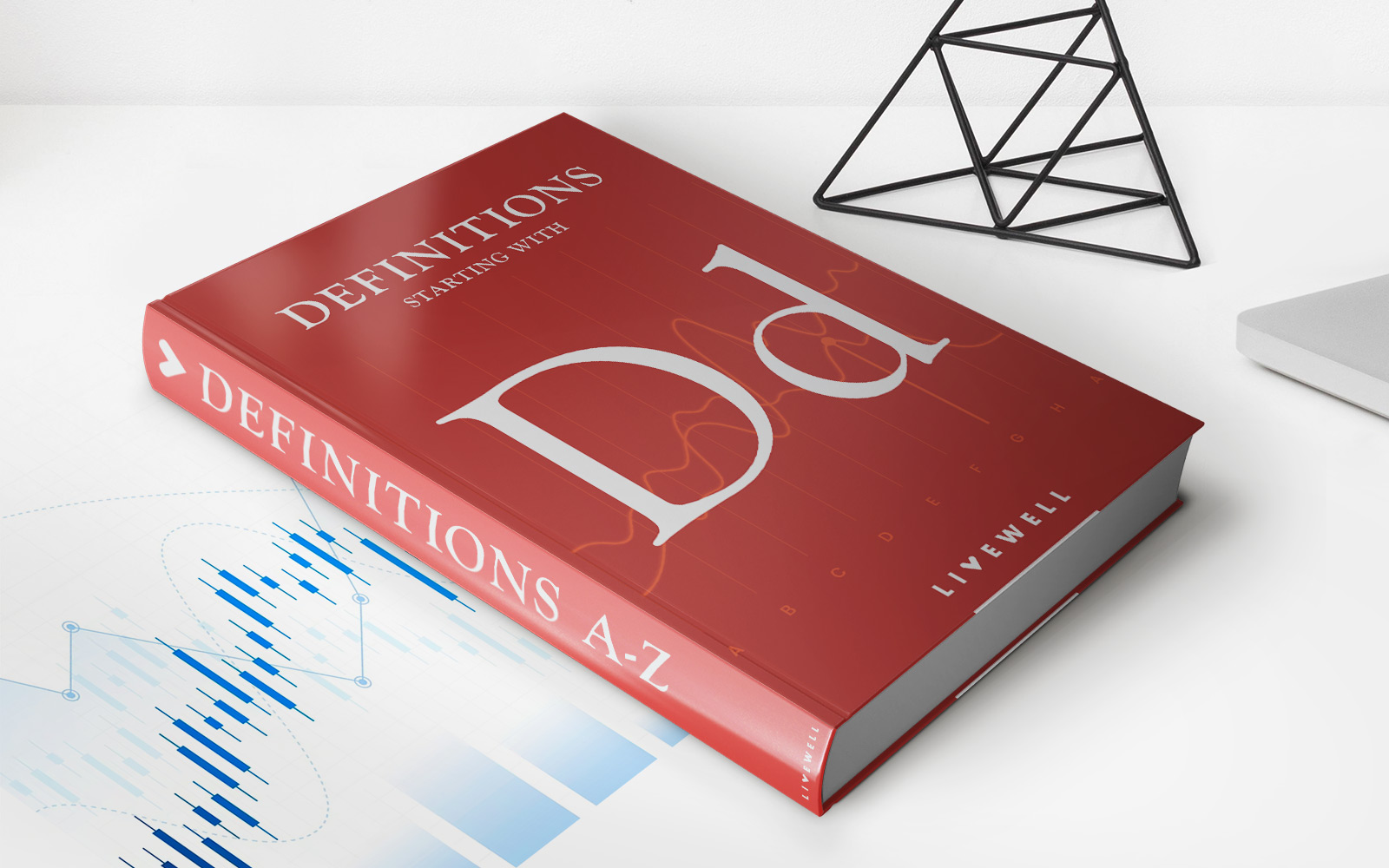
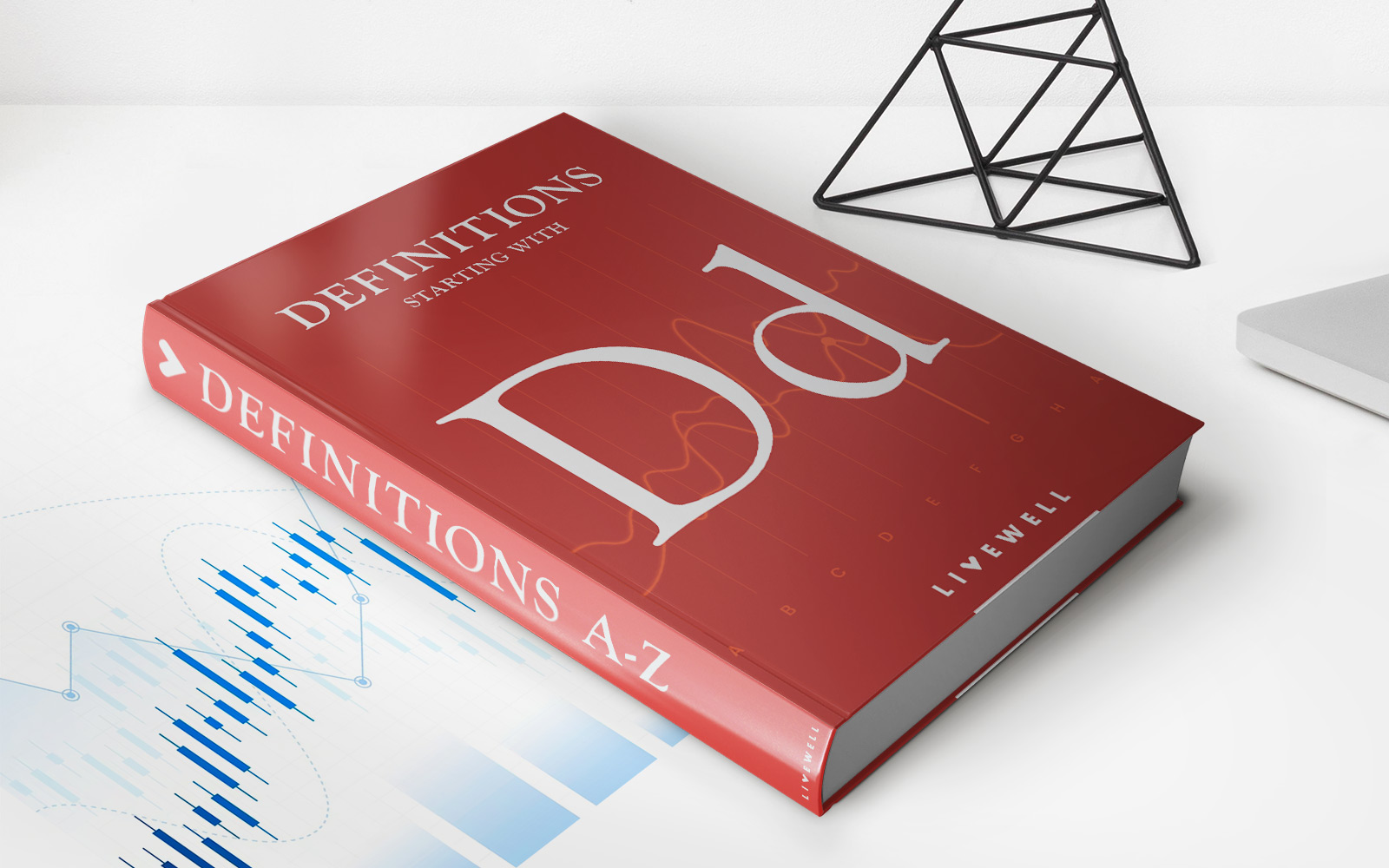
Finance
Default Model Definition
Published: November 9, 2023
Discover the default model definition in the finance industry and understand its importance in managing financial risks and optimizing decision-making processes.
(Many of the links in this article redirect to a specific reviewed product. Your purchase of these products through affiliate links helps to generate commission for LiveWell, at no extra cost. Learn more)
Understanding Default Model Definition in Finance
When it comes to the world of finance, there are numerous concepts and models that play a crucial role in making informed decisions. One such concept is Default Model Definition. So, what exactly is a Default Model? In simple terms, it is a mathematical model used to predict the likelihood of default for a borrower, typically in the context of credit risk assessment. In this blog post, we will delve deeper into the intricacies of Default Model Definition, its significance, and how it is applied in the financial industry.
Key Takeaways:
- Default models are used to assess the probability of a borrower defaulting on their financial obligations.
- These models employ various statistical techniques and historical data to generate accurate predictions.
Why are Default Models Important?
In the realm of finance, accurate risk assessment plays a crucial role in determining the lending practices of banks and financial institutions. Default models provide a quantitative framework for assessing credit risk and help in maintaining a healthy loan portfolio. Here are two key reasons why default models are important:
- Improved Decision-Making: By utilizing default models, lenders can gain valuable insights into the creditworthiness of potential borrowers. These models consider a wide range of factors such as credit scores, income levels, employment history, and payment patterns to evaluate the risk associated with lending money. Based on these insights, lenders can make informed decisions about loan approvals, interest rates, and credit limits, reducing the chances of defaults and financial losses.
- Portfolio Management: Banks and financial institutions often have a diverse range of loans and credit products. Default models enable them to analyze and manage the risk associated with their loan portfolio. By assessing the overall credit risk, lenders can appropriately allocate their resources, establish risk mitigation strategies, and continuously monitor the health of their portfolio.
How are Default Models Developed and Applied?
Default models are typically built using historical data and statistical techniques. The process involves collecting relevant data on borrowers, such as their credit history, income, employment stability, and demographic information. This dataset is then used to create a mathematical model that predicts the likelihood of default based on the given variables.
Various statistical methods, such as logistic regression, decision trees, or machine learning algorithms, can be applied to analyze the data and identify the key factors that contribute to default risk. These models are then tested and validated using historical data to ensure their accuracy and reliability.
Once developed, default models can be used in different financial scenarios. For example, banks can utilize them during the loan origination process to assess the creditworthiness of potential borrowers. These models can also be utilized for ongoing credit monitoring and to determine the need for risk mitigation strategies, such as adjusting interest rates or modifying credit terms.
Conclusion
Default Model Definition is a critical aspect of risk assessment in the financial industry. By utilizing these models, lenders can make more informed decisions about lending practices, optimize their loan portfolios, and minimize the chances of defaults. Understanding the intricacies of default models is essential for finance professionals and individuals seeking credit, as it provides valuable insights into credit risk assessment.
Remember, default models are continually evolving with advancements in technology and data analysis techniques. Staying abreast of these developments can help finance professionals stay ahead in the ever-changing world of credit risk management.