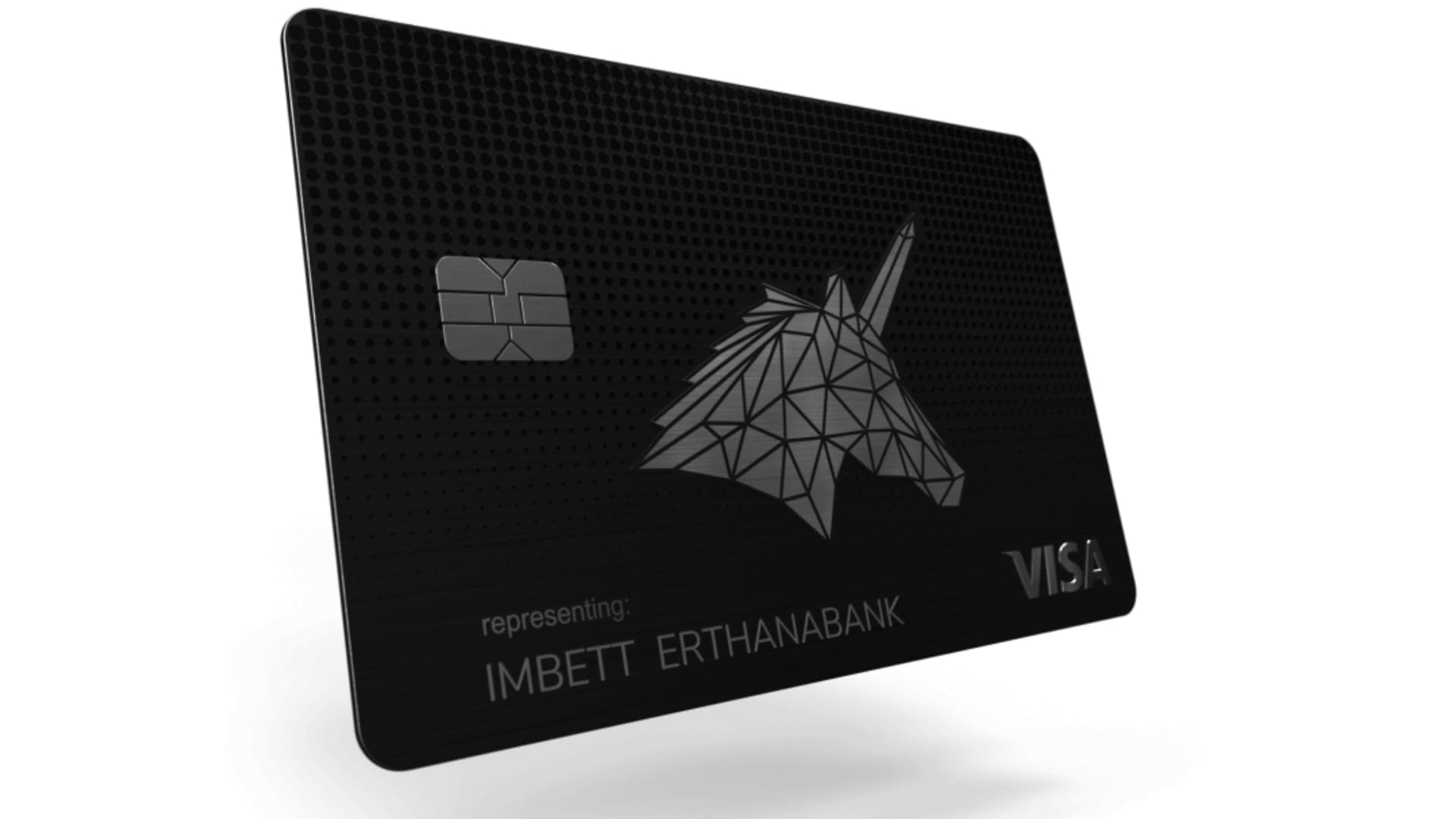
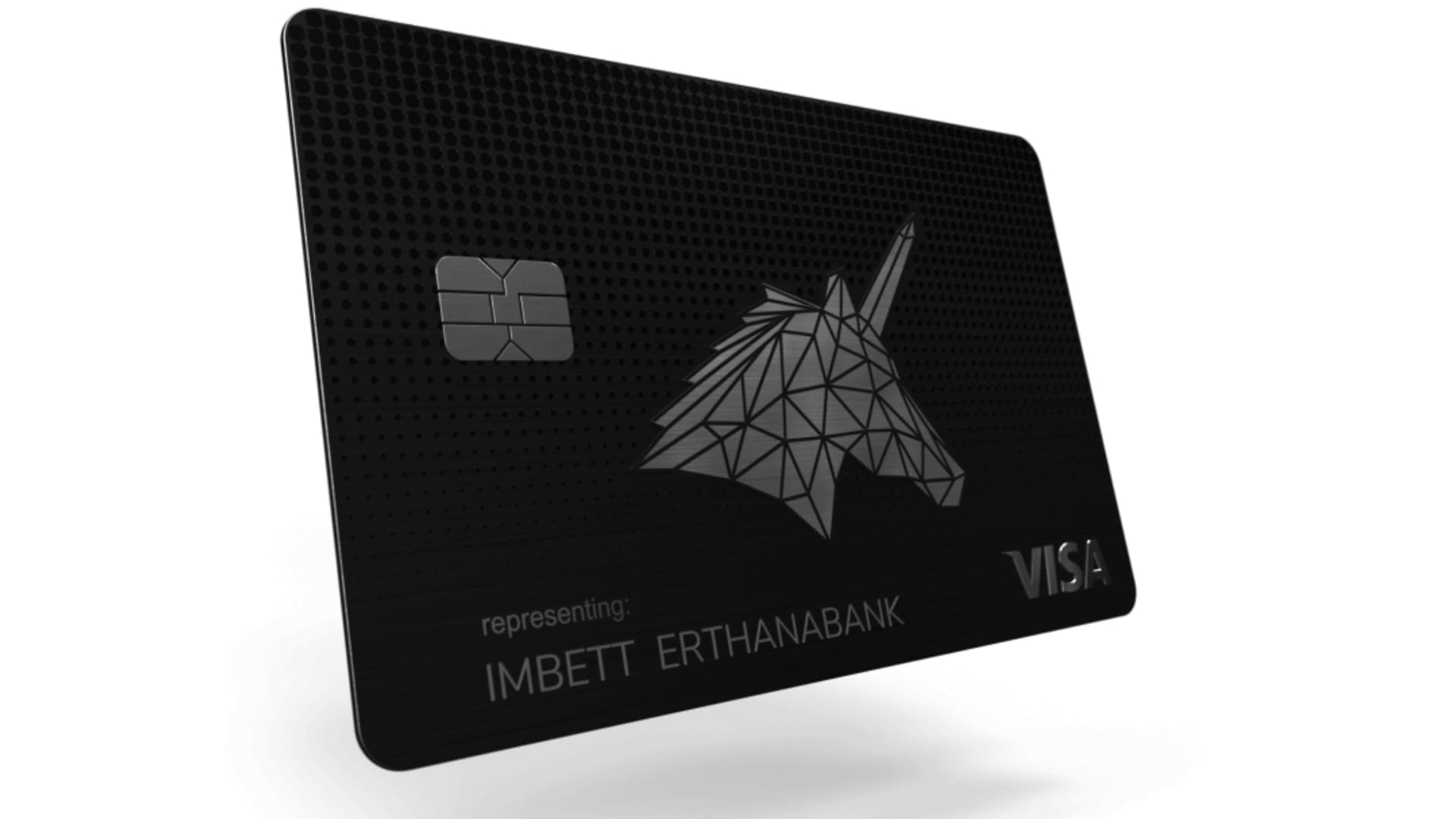
Finance
What Is Credit AI
Published: January 11, 2024
Discover how Credit AI is revolutionizing the world of finance with advanced technology and intelligent algorithms. Enhance your financial decisions and streamline processes with this groundbreaking platform.
(Many of the links in this article redirect to a specific reviewed product. Your purchase of these products through affiliate links helps to generate commission for LiveWell, at no extra cost. Learn more)
Table of Contents
Introduction
Artificial Intelligence (AI) has revolutionized various industries, including finance. In the realm of finance, one particular application of AI that has gained traction is Credit AI. Credit AI is a powerful tool that leverages advanced algorithms and machine learning to assess creditworthiness and make informed decisions.
Traditionally, credit evaluation has relied heavily on manual processes, subjective judgments, and historical data analysis. However, with the emergence of Credit AI, financial institutions are now able to automate and streamline the credit assessment process, resulting in more accurate and efficient credit decision-making.
Credit AI has the potential to transform the lending landscape by providing lenders with deeper insights into borrowers’ creditworthiness, enabling them to make more informed lending decisions. By analyzing vast amounts of data, Credit AI algorithms can identify patterns, assess risk profiles, and predict the likelihood of default more effectively than ever before.
Moreover, Credit AI has also democratized access to credit. It allows lenders to evaluate the creditworthiness of individuals who may have limited credit history or lack traditional forms of collateral. By considering various alternative data points like social media behavior, payment history, and educational background, Credit AI algorithms can provide a more comprehensive and inclusive assessment of borrowers’ creditworthiness.
However, as with any technological advancement, there are ethical considerations and potential challenges associated with the use of Credit AI. It is crucial to strike a balance between leveraging AI to improve the credit assessment process and ensuring fairness, transparency, and protection of consumer rights.
In this article, we will explore the concept of Credit AI in detail. We will delve into its definition, discuss how it works, highlight its benefits and applications, and also examine the challenges and limitations it may face. Furthermore, we will address the ethical considerations that must be taken into account when implementing Credit AI. By the end of this article, you will have a comprehensive understanding of the role that Credit AI plays in the finance industry and its impact on borrowers and lenders alike.
Definition of Credit AI
Credit AI, also known as Credit Artificial Intelligence, refers to the use of advanced algorithms and machine learning techniques to assess the creditworthiness of individuals or businesses. It is a subfield of AI that focuses specifically on automating and optimizing the credit evaluation process for lenders.
At its core, Credit AI aims to analyze vast amounts of data from various sources, including financial records, credit history, employment information, and alternative data points, to generate predictive models that determine the likelihood of a borrower’s creditworthiness. By leveraging machine learning algorithms, Credit AI algorithms can identify patterns, trends, and correlations in the data to make accurate credit assessments.
Unlike traditional credit evaluation methods that heavily rely on manual processes and subjective judgments, Credit AI offers a more objective and data-driven approach. It eliminates biases and inconsistencies associated with human decision-making, allowing lenders to make more informed lending decisions in a more efficient and consistent manner.
Credit AI algorithms can consider a wide range of factors when assessing creditworthiness. These factors may include credit history, income levels, debt-to-income ratio, financial stability, employment history, educational background, and even social media behavior. By analyzing these data points holistically, Credit AI algorithms can provide a comprehensive assessment of a borrower’s creditworthiness, even for those without a traditional credit file or collateral.
Furthermore, Credit AI can also play a crucial role in credit risk management for lenders. By continuously monitoring borrowers’ financial behaviors and market trends, Credit AI algorithms can identify early warning signs of default or financial distress. This allows lenders to take proactive measures and minimize their credit risks.
It’s important to note that Credit AI is not a substitute for human expertise and decision-making. Instead, it serves as a powerful tool to assist lenders in making more accurate and efficient credit evaluations. Lenders still play a critical role in reviewing the credit assessments generated by Credit AI algorithms and making the final lending decisions.
Overall, Credit AI is revolutionizing the credit industry by automating and optimizing credit evaluation. It allows lenders to make more data-driven, accurate, and fair credit decisions, while also expanding access to credit for individuals and businesses who may have been overlooked by traditional credit evaluation methods.
How Credit AI Works
Credit AI works by leveraging advanced algorithms and machine learning techniques to analyze vast amounts of data and generate predictive models that assess creditworthiness. The process of how Credit AI works can be broadly summarized into the following steps:
- Data Collection: Credit AI algorithms begin by collecting and aggregating various data points from multiple sources. This data may include financial records, credit history, employment information, educational background, and alternative data such as social media behavior. The more data the algorithm has access to, the more accurate its assessment can be.
- Data Preprocessing: Once the data is collected, it undergoes preprocessing to clean and transform it into a suitable format for analysis. This step involves removing outliers, dealing with missing values, and standardizing data to ensure consistency and accuracy.
- Feature Extraction: After the data is preprocessed, Credit AI algorithms identify the most relevant features that are likely to impact the creditworthiness of an individual or business. These features may include credit history, income levels, debt-to-income ratio, employment stability, and more. Feature extraction helps to reduce dimensionality and improve the efficiency of the algorithm.
- Model Training: In this step, the Credit AI algorithm utilizes machine learning techniques to train a predictive model based on the collected and preprocessed data. The algorithm learns from historical patterns and correlations to make predictions about future creditworthiness. This process involves selecting appropriate machine learning algorithms, fine-tuning model parameters, and evaluating the model’s performance.
- Credit Assessment: Once the model is trained, it can be used to assess the creditworthiness of new borrowers. When a borrower’s data is fed into the algorithm, it generates a credit score or probability indicating the likelihood of default or repayment. Lenders can then use this assessment to make more informed lending decisions.
- Monitoring and Updating: Credit AI algorithms are dynamic and continuously adapt to changing patterns and market conditions. Lenders can monitor borrowers’ financial behaviors and update the model as new data becomes available. This allows for real-time credit risk management and proactive measures to minimize potential defaults.
Credit AI algorithms are designed to learn and improve over time. With more data and feedback from lenders, the algorithm becomes more accurate and sophisticated in assessing creditworthiness. However, it’s important to ensure that the algorithms are regularly evaluated and validated to maintain fairness, transparency, and compliance with regulations.
Overall, the combination of data collection, preprocessing, feature extraction, model training, credit assessment, and continuous monitoring forms the foundation of how Credit AI works. It revolutionizes the credit evaluation process by automating and optimizing decision-making, ultimately providing lenders with a more accurate and efficient approach to assessing creditworthiness.
Benefits of Credit AI
Credit AI offers numerous benefits to borrowers, lenders, and the overall financial industry. Here are some of the key advantages of implementing Credit AI:
- Improved Accuracy: Credit AI algorithms analyze vast amounts of data and utilize advanced machine learning techniques to make credit assessments. This results in more accurate predictions of creditworthiness compared to traditional manual methods. By considering a wide range of factors and identifying patterns, Credit AI algorithms can better assess credit risk and make more informed lending decisions.
- Efficiency and Speed: Credit AI streamlines the credit evaluation process by automating data collection, preprocessing, model training, and credit assessment. This significantly reduces the time and effort required for lenders to evaluate borrowers’ creditworthiness. The result is faster credit approvals and a more efficient lending process.
- Expanded Access to Credit: Traditional credit evaluation methods often rely heavily on credit history and collateral, making it challenging for individuals or businesses with limited credit history or unconventional forms of collateral to access credit. Credit AI incorporates alternative data points, such as social media behavior and educational background, allowing lenders to provide credit to a broader range of borrowers who may otherwise be overlooked.
- Objective and Consistent Decision-Making: Credit AI eliminates biases and subjectivity inherent in manual credit evaluations. By relying on data-driven algorithms, Credit AI ensures more objective and consistent decision-making. This promotes fairness in lending and reduces the potential for discriminatory practices.
- Better Risk Management: Credit AI algorithms continuously monitor borrowers’ financial behaviors and market trends. This enables lenders to identify early warning signs of default or financial distress, allowing them to take proactive measures to manage risk. By better managing credit risk, lenders can protect their investments and improve the overall stability of the financial system.
- Enhanced Customer Experience: Credit AI simplifies and accelerates the credit application and approval process for borrowers. The use of automated systems and real-time credit assessments provides borrowers with a seamless and convenient experience. Additionally, the expanded access to credit offered by Credit AI allows more individuals and businesses to fulfill their financial needs.
- Data-Driven Insights: Credit AI generates a wealth of data and insights that can be valuable for lenders and the financial industry as a whole. By analyzing patterns and trends in credit assessments, lenders can gain a deeper understanding of borrower behavior and market dynamics. This information can be used to develop more targeted financial products and services.
Overall, Credit AI offers significant benefits by improving accuracy, efficiency, access to credit, decision-making, risk management, and customer experience. By embracing the power of AI, lenders can unlock new opportunities, make more informed lending decisions, and drive the growth and advancement of the financial industry.
Applications of Credit AI
Credit AI has a wide range of applications across the finance industry, impacting various aspects of lending and credit evaluation. Here are some of the key applications of Credit AI:
- Credit Scoring: Credit AI is commonly used for credit scoring, which involves assessing the creditworthiness of individuals or businesses. By analyzing multiple data points, such as credit history, income levels, debt-to-income ratio, and alternative data, Credit AI algorithms generate credit scores or probabilities that help lenders make informed lending decisions.
- Alternative Lending: Credit AI has facilitated the rise of alternative lending platforms. These platforms utilize Credit AI algorithms to assess creditworthiness based on non-traditional data sources, such as social media behavior and educational background. This allows lenders to offer loans to individuals or businesses who may have limited credit history or unconventional collateral.
- Fraud Detection: Credit AI can be used for fraud detection in credit applications. By analyzing patterns and anomalies within the data, Credit AI algorithms can identify potential fraudulent activities, such as identity theft or false income claims. This helps to mitigate financial risks and protect lenders’ interests.
- Credit Portfolio Management: Credit AI plays a vital role in credit portfolio management for financial institutions. By continuously monitoring borrowers’ financial behaviors and analyzing market trends, Credit AI algorithms provide insights into the creditworthiness and risk profiles of borrowers within a lender’s portfolio. This allows lenders to proactively manage credit risk and optimize their loan portfolios.
- Credit Risk Assessment: Credit AI assists in credit risk assessment by predicting the likelihood of default or repayment based on historical data and patterns. Lenders can use this information to allocate appropriate provisions for potential loan losses and manage their overall credit risk exposure more effectively.
- Loan Underwriting: Credit AI simplifies and automates the loan underwriting process by assessing various parameters related to creditworthiness. This allows lenders to make quicker and more accurate decisions, resulting in faster loan approvals and a smoother customer experience.
- Credit Limit Determination: Credit AI algorithms can help determine appropriate credit limits for individual borrowers by analyzing their financial profile, income stability, and repayment history. This ensures that borrowers are offered credit limits that align with their ability to repay, reducing the risk of default and promoting responsible lending.
These are just a few examples of the diverse applications of Credit AI in the finance industry. As technology continues to evolve, new applications and use cases for Credit AI are likely to emerge, further transforming the way credit is evaluated, and granting access to finance for a broader range of individuals and businesses.
Challenges and Limitations of Credit AI
While Credit AI offers numerous benefits, there are also several challenges and limitations that need to be addressed. It is important to consider these factors to ensure the ethical and responsible use of Credit AI in the finance industry. Here are some of the key challenges and limitations:
- Data Bias and Discrimination: Credit AI algorithms heavily rely on historical data to make credit assessments. If the historical data contains biases or discriminatory patterns, the algorithms can perpetuate unfair lending practices. It is crucial to ensure that the data used is diverse, representative, and free from biases to prevent discrimination against certain individuals or groups.
- Lack of Explainability: The complexity of Credit AI algorithms often results in a lack of transparency and explainability. It can be challenging to understand the factors or variables that contribute to credit decisions. This lack of explainability raises concerns about fairness, accountability, and the ability for borrowers to dispute or challenge credit assessments.
- Data Privacy and Security: Credit AI relies on vast amounts of personal and financial data. It is essential to handle this data with utmost care and ensure compliance with privacy regulations, such as GDPR or CCPA. Moreover, the security of the data and protection against cyber threats must be a top priority to mitigate the risk of data breaches or misuse.
- Algorithmic Bias: Credit AI algorithms can inadvertently incorporate biases and prejudices present in the data they learn from. If not addressed, these biases can lead to discriminatory outcomes, reinforcing existing social and economic disparities. It is vital to constantly evaluate and monitor algorithms to identify and mitigate any bias in their decision-making process.
- Overreliance on Historical Data: Credit AI algorithms heavily depend on historical data to make predictions about creditworthiness. However, historical data may not always be a reliable predictor of future behavior, especially during economic downturns or unforeseen events. It is important to strike a balance between using historical data and incorporating real-time or dynamic factors to make accurate credit assessments.
- Limited Access for Unbanked Individuals: While Credit AI expands access to credit for many individuals, it may still present challenges for unbanked individuals who lack traditional financial records or alternative data sources. These individuals may continue to face barriers in accessing credit despite the potential benefits offered by Credit AI.
- Model Risk and Instability: Credit AI models may suffer from instability or provide inaccurate assessments if not properly validated or calibrated. External factors or changes in market conditions can impact model performance over time. Continuous monitoring and evaluation of the models are necessary to maintain their accuracy and reliability.
Addressing these challenges and limitations is crucial to ensure that Credit AI is used responsibly and ethically. Collaboration between regulators, industry stakeholders, and technology experts is essential to establish standards, guidelines, and best practices to mitigate these risks and ensure that the benefits of Credit AI are realized without compromising consumer protection and fairness.
Ethical Considerations in Credit AI
As Credit AI continues to shape the landscape of credit evaluation, it is essential to address the ethical considerations associated with its implementation. Here are some key ethical considerations that must be taken into account:
- Fairness and Bias: Credit AI algorithms should be designed and monitored to ensure fairness and prevent biases. It is crucial to avoid discriminating against individuals or groups based on factors such as race, gender, age, or other protected characteristics. Regular audits and assessments of the algorithms should be conducted to identify and mitigate any biases that may be embedded in the data or algorithmic processes.
- Transparency and Explainability: It is important to ensure transparency and explainability in the credit assessment process. Borrowers should have access to clear and understandable explanations regarding the factors that contribute to their creditworthiness assessment. Lenders and financial institutions should strive to disclose the use of Credit AI and provide avenues for borrowers to address concerns or disputes regarding their credit assessments.
- Data Privacy and Security: Protecting the privacy and security of borrower data is paramount. Lenders should strictly adhere to privacy regulations and implement robust security measures to safeguard personal and financial information from unauthorized access or misuse. Borrowers should have control over their data and the ability to provide informed consent for its use in credit evaluation.
- Consent and Opt-Out: Borrowers should have the right to give informed consent for their data to be used in Credit AI algorithms. Additionally, they should be provided with the option to opt-out of having their data used for credit assessments if they so choose. Providing borrowers with control over their data fosters transparency, respect for autonomy, and the ability to make informed decisions regarding their financial information.
- Accountability and Oversight: There should be clear mechanisms in place to ensure accountability and oversight of Credit AI systems. Regulators play a vital role in monitoring the use of Credit AI, assessing compliance with ethical guidelines, and addressing any concerns related to potential misuse or unfair practices. Financial institutions should also establish internal governance frameworks to ensure responsible and ethical use of Credit AI.
- Continual Evaluation and Improvement: Credit AI systems should be subject to continual evaluation and improvement. This involves monitoring for biases, reassessing models for accuracy, and ensuring the algorithms adapt to changing socio-economic conditions. Regular auditing and validation should occur to identify any ethical issues that may arise and implement appropriate corrective measures.
By addressing these ethical considerations, Credit AI can be deployed in a responsible and inclusive manner. Collaboration between industry stakeholders, regulators, and technology experts is essential to establish guidelines, foster transparency, and ensure that the use of Credit AI aligns with ethical standards and societal expectations.
Conclusion
Credit AI has emerged as a transformative force in the finance industry, revolutionizing the way creditworthiness is assessed. Through advanced algorithms and machine learning techniques, Credit AI offers numerous benefits, including improved accuracy, efficiency, expanded access to credit, and better risk management. It has the potential to democratize lending and provide individuals and businesses with fair and inclusive opportunities to access financial resources.
However, as with any technological advancement, there are important considerations to address. Ethical concerns such as fairness, bias, explainability, data privacy, and accountability must be at the forefront when implementing Credit AI. Striking the right balance between leveraging AI for credit assessment while ensuring transparency, protection of consumer rights, and compliance with regulations is essential.
The challenges and limitations associated with Credit AI, such as data bias, lack of explainability, and overreliance on historical data, need to be carefully managed. The continuous evaluation, monitoring, and improvement of algorithms, as well as collaboration between regulators, industry stakeholders, and experts, are vital to address these challenges responsibly.
Overall, Credit AI has the potential to revolutionize the lending landscape, making credit assessment more accurate, efficient, and accessible. By embracing the benefits of Credit AI while focusing on ethical considerations, lenders can unlock opportunities for growth, provide fair access to credit, and foster a more inclusive financial system.
As Credit AI continues to evolve, it is essential to prioritize ethical practices, transparency, and consumer protection. By doing so, we can harness the power of AI to drive innovation, improve financial services, and make a positive impact on the lives of borrowers and lenders alike.