Home>Finance>Residual Standard Deviation: Definition, Formula, And Examples
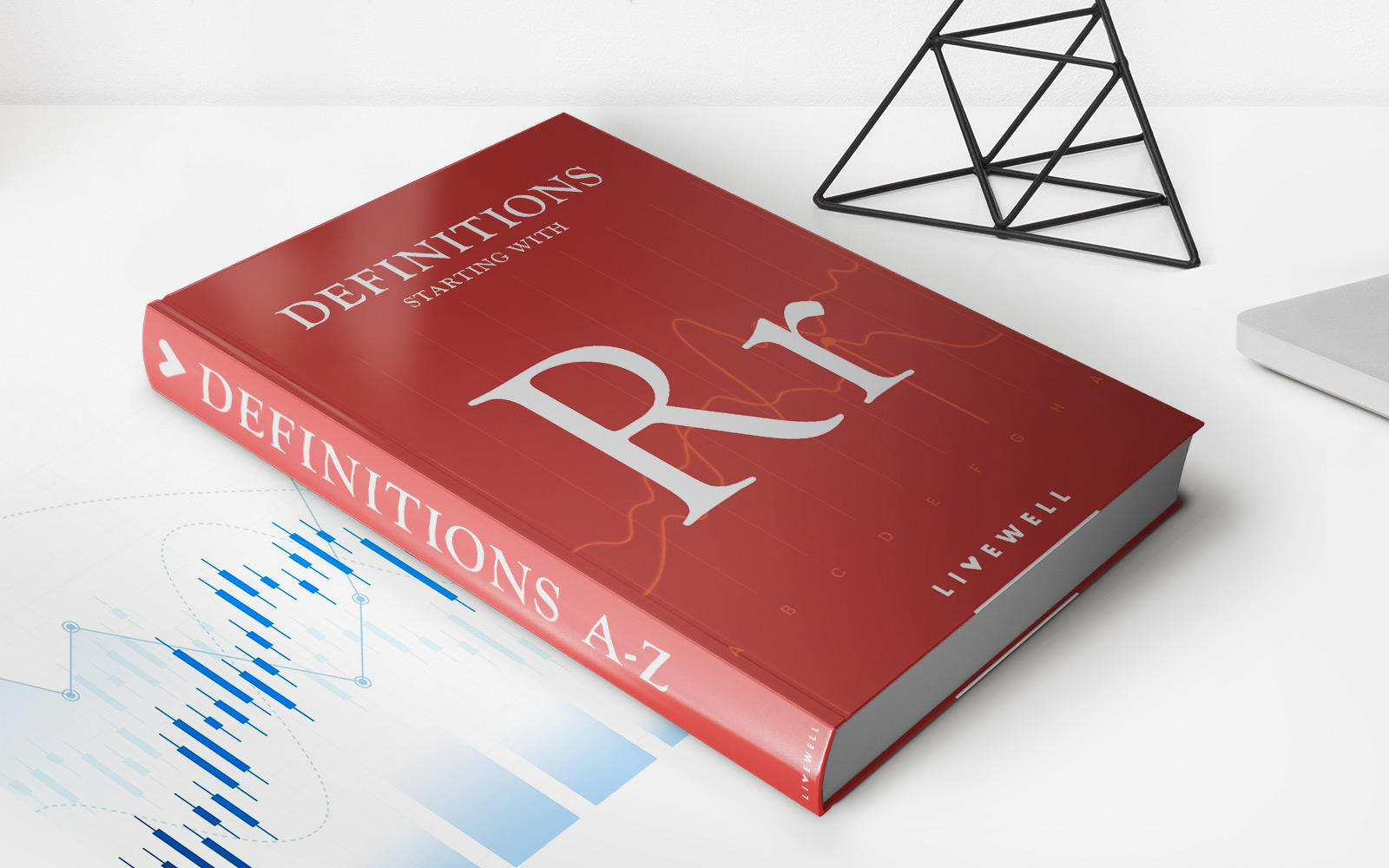
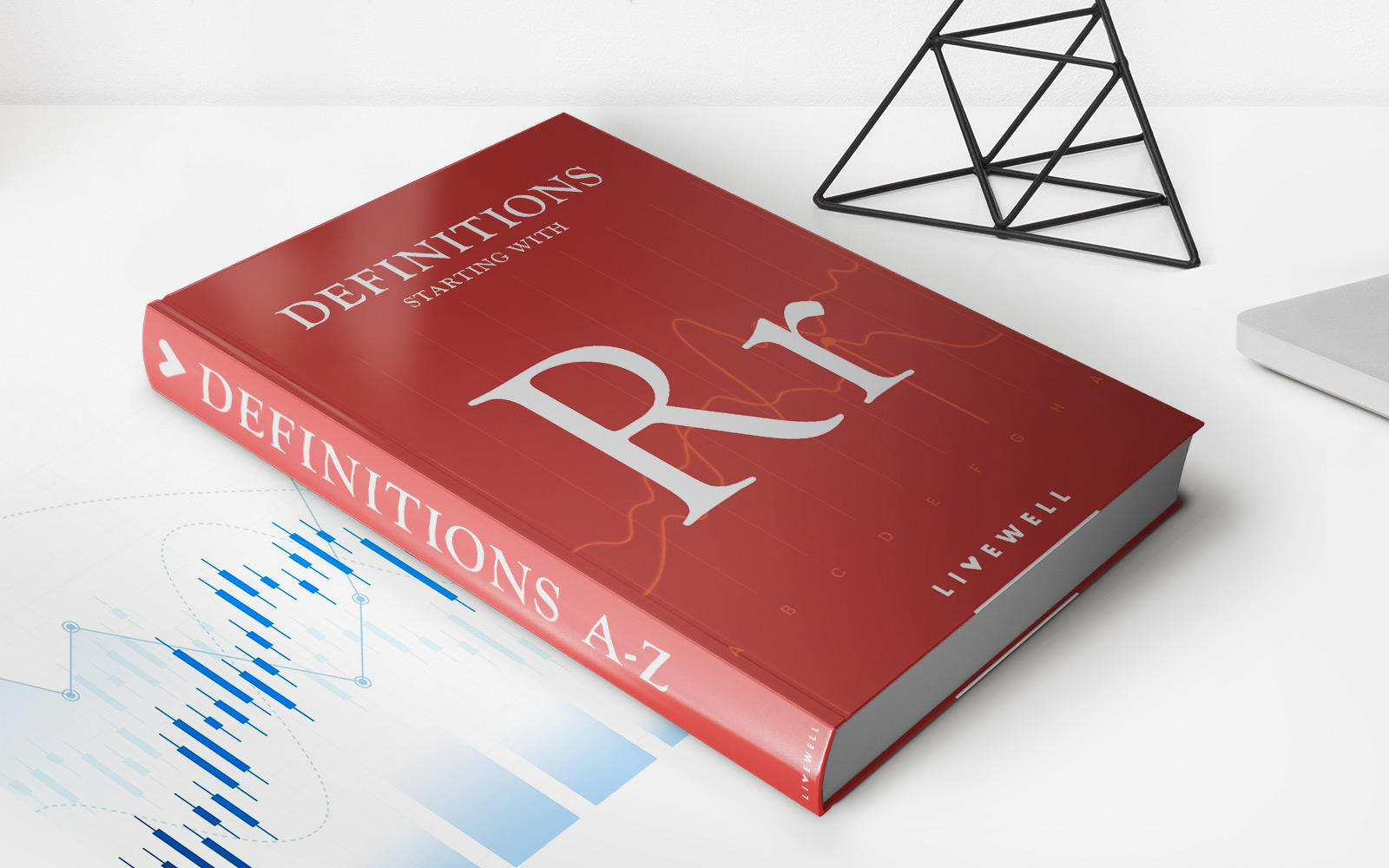
Finance
Residual Standard Deviation: Definition, Formula, And Examples
Published: January 19, 2024
Learn about the residual standard deviation in finance. Understand its definition, formula, and explore examples to enhance your understanding.
(Many of the links in this article redirect to a specific reviewed product. Your purchase of these products through affiliate links helps to generate commission for LiveWell, at no extra cost. Learn more)
Residual Standard Deviation: Definition, Formula, and Examples
Are you looking to understand the concept of residual standard deviation? In the world of finance, this term is often used to measure the dispersion of data points around a regression line. By calculating the residual standard deviation, you can gain valuable insights into the accuracy and reliability of a statistical model or prediction. In this blog post, we will explore the definition, formula, and provide examples to help you better understand residual standard deviation.
Key Takeaways:
- Residual standard deviation measures the dispersion of data points around a regression line.
- It provides insights into the accuracy and reliability of a statistical model or prediction.
Firstly, let’s define residual standard deviation. It is also known as residual standard error or root mean square error (RMSE). Residuals are the differences between the observed values and the predicted values in a regression analysis. The residual standard deviation calculates the average distance of these residuals from the regression line. Essentially, it helps us understand how much the actual observations deviate from the predicted values, giving us an indication of the accuracy and precision of the model.
The formula for calculating residual standard deviation is as follows:
Residual Standard Deviation = √(∑(observed value – predicted value)^2 / (n – 2))
Where:
- Observed value is the actual value of the data point.
- Predicted value is the value predicted by the regression line.
- n is the number of data points used in the regression analysis.
Let’s delve into an example to fully grasp the concept. Suppose you are analyzing a dataset of housing prices based on factors such as area, number of bedrooms, and distance from city center. After performing a regression analysis, you obtain a residual standard deviation of $10,000. This means that, on average, the predicted housing prices deviate from the actual prices by $10,000. The residual standard deviation essentially acts as a measure of the model’s precision. The lower the value, the better the model fits the data.
Now, you may wonder, why is residual standard deviation an important metric? Here are a few reasons:
- Evaluating Model Accuracy: Residual standard deviation helps assess the accuracy and correctness of a model. By comparing the predicted values with the actual observations, you can determine how well the model captures the underlying patterns and variability in the data.
- Comparing Models: If you are considering multiple regression models, the residual standard deviation allows you to compare their performances. A lower residual standard deviation indicates a better-fitting model.
- Detecting Outliers: Large residuals may indicate the presence of outliers or influential data points that significantly affect the regression line. Examining the residuals and residual standard deviation can help identify these influential points.
- Validating Statistical Models: The residual standard deviation is a useful tool for validating the assumptions of a statistical model. By analyzing the residuals, you can determine if the model assumptions, such as linearity or homoscedasticity, are violated.
In conclusion, residual standard deviation is a crucial metric in finance for evaluating the accuracy, reliability, and performance of regression models. By quantifying the dispersion of data points around a regression line, it provides valuable insights into the precision and validity of statistical predictions. Understanding this concept can enable better decision-making in investments, risk management, and other areas where statistical modeling is employed. So, the next time you encounter residual standard deviation, you’ll be equipped with the knowledge to leverage it effectively.