Home>Finance>Seasonal Adjustment: Definition, Calculation Methods, Example
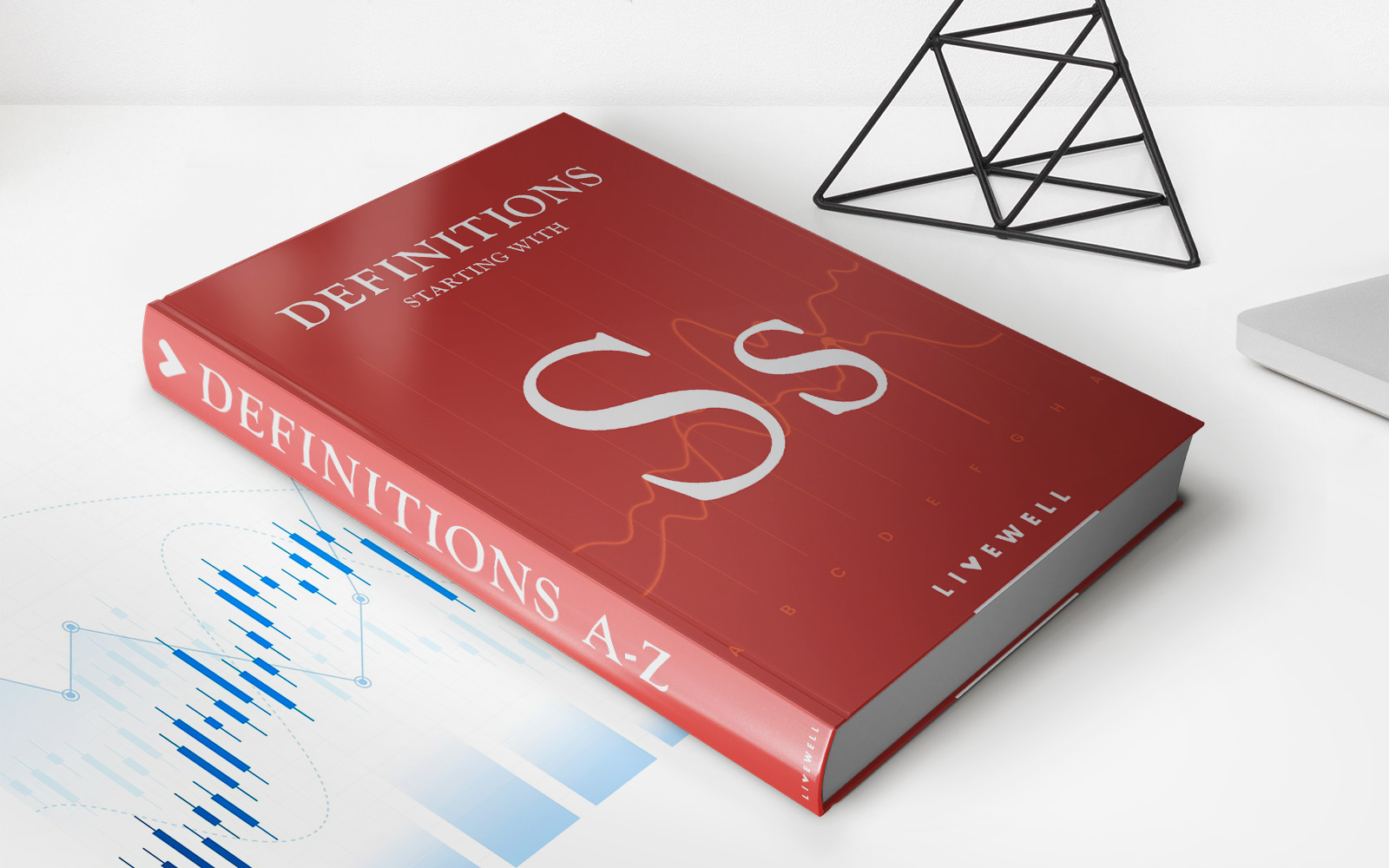
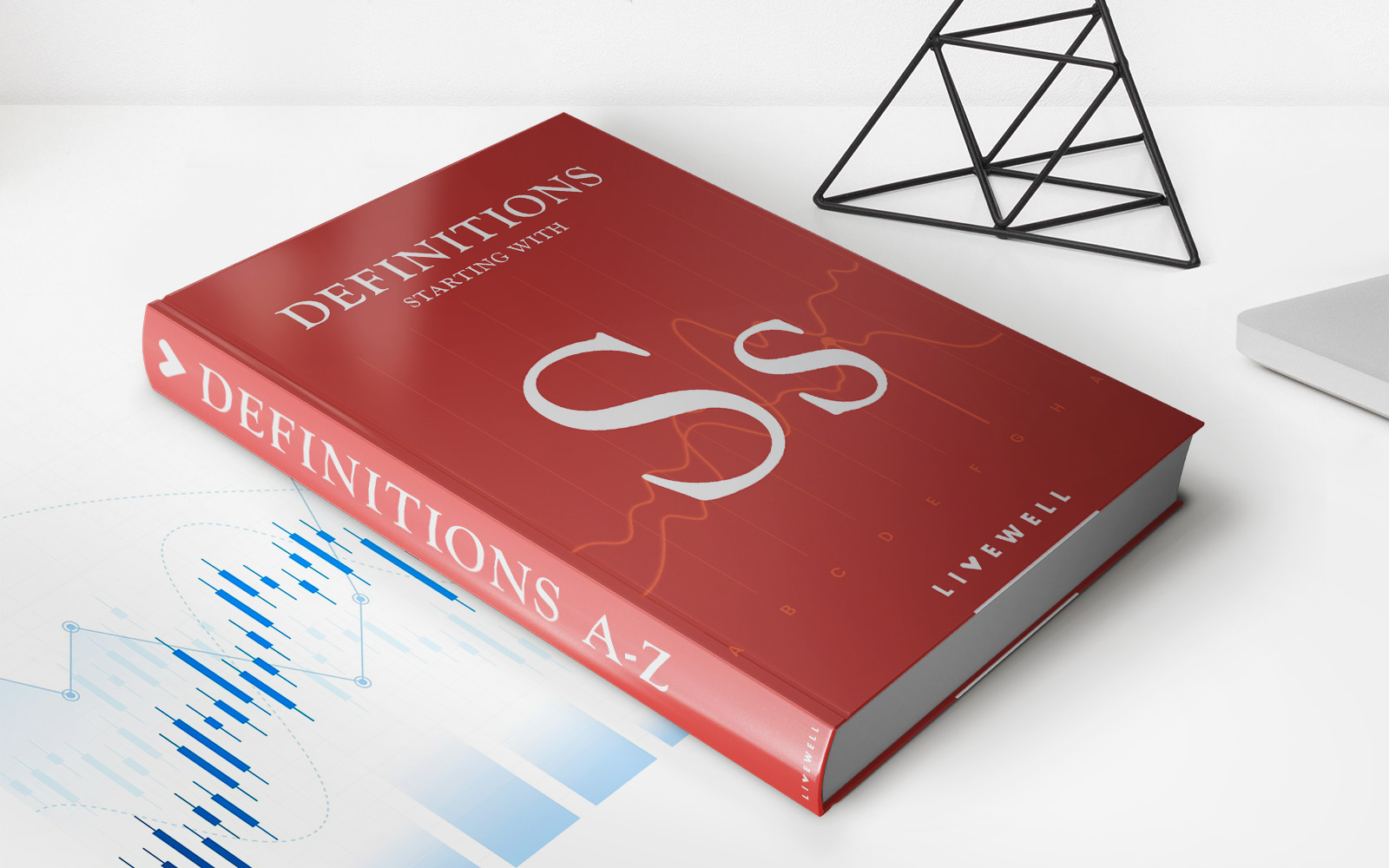
Finance
Seasonal Adjustment: Definition, Calculation Methods, Example
Published: January 23, 2024
Learn what seasonal adjustment is in finance, how it is calculated, and get an example. Understand the significance of seasonal adjustment in financial analysis.
(Many of the links in this article redirect to a specific reviewed product. Your purchase of these products through affiliate links helps to generate commission for LiveWell, at no extra cost. Learn more)
Understanding Seasonal Adjustment: Definition, Calculation Methods, Example
Gaining a deeper understanding of seasonal adjustment can be immensely beneficial in the field of finance. Seasonal adjustment allows data analysts, economists, and decision-makers to make accurate comparisons and predictions by removing the influence of seasonal patterns. In this blog post, we’ll explore what seasonal adjustment entails, the various calculation methods used, and provide a real-life example to illustrate its practical applications.
Key Takeaways:
- Seasonal adjustment helps to eliminate the effects of predictable seasonal patterns from economic and financial data.
- There are multiple calculation methods for seasonal adjustment, including the X-12-ARIMA, TRAMO-SEATS, and Census X-13ARIMA-SEATS techniques.
What is Seasonal Adjustment?
Seasonal adjustment is a statistical technique used to remove the predictable patterns or fluctuations that occur within a specific time frame, often referred to as seasons. These patterns can be influenced by various factors such as weather, holidays, cultural events, and other recurring events that affect economic and financial data.
The primary goal of seasonal adjustment is to obtain a more accurate representation of the underlying trend and help data analysts identify irregular movements or anomalies that may be obscured by seasonal effects.
Calculation Methods for Seasonal Adjustment
There are several calculation methods used for seasonal adjustment, each with its unique approach. Some of the commonly employed techniques include:
1. X-12-ARIMA: This method uses an automated procedure to identify and estimate seasonal patterns in time series data. It incorporates autoregressive integrated moving average (ARIMA) modeling to capture the seasonal fluctuations accurately.
2. TRAMO-SEATS: Developed by the Bank of Spain and the Bank of Italy, TRAMO-SEATS is a program that combines a time series regression model (TRAMO) with the Signal Extraction in ARIMA Time Series (SEATS) technique. This approach allows for the decomposition of a time series into its different components, including trend, seasonal, irregular, and calendar effects.
3. Census X-13ARIMA-SEATS: This is a widely recognized method developed by the U.S. Census Bureau that combines ARIMA and SEATS approaches. It provides highly accurate seasonal adjustment by detecting and correcting outliers and estimating seasonal and trend components of the data.
An Example: Unemployment Rate
To illustrate the practical application of seasonal adjustment, let’s consider the example of the unemployment rate in a specific region. Unemployment rates tend to exhibit seasonal patterns due to various factors such as hiring cycles, school graduations, and the tourism industry.
By applying seasonal adjustment techniques to the unemployment rate data, analysts can eliminate the anticipated fluctuations caused by these factors. This allows for more accurate comparisons of unemployment rates across different months or years and facilitates the identification of underlying economic trends, such as long-term unemployment rate changes or potential labor market improvements.
In conclusion, understanding seasonal adjustment and its calculation methods is essential for data analysts and finance professionals to make informed decisions. By removing the influence of seasonal patterns, analysts can gain better insights into industry trends, business performance, and economic indicators. Implementing seasonal adjustment techniques empowers decision-makers to optimize their strategies and adapt to changing market conditions effectively.