Home>Finance>Serial Correlation: Definition, How To Determine, And Analysis
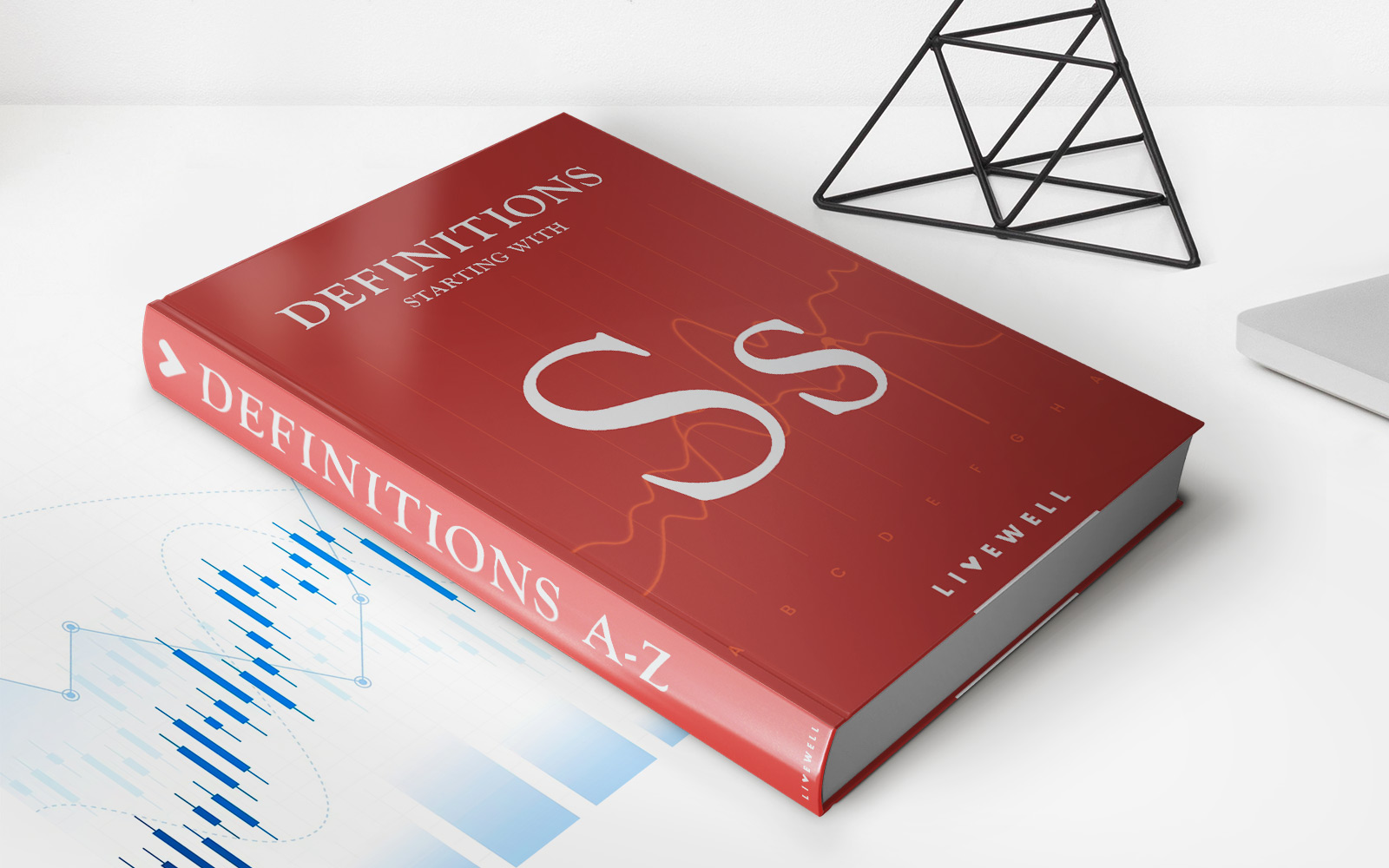
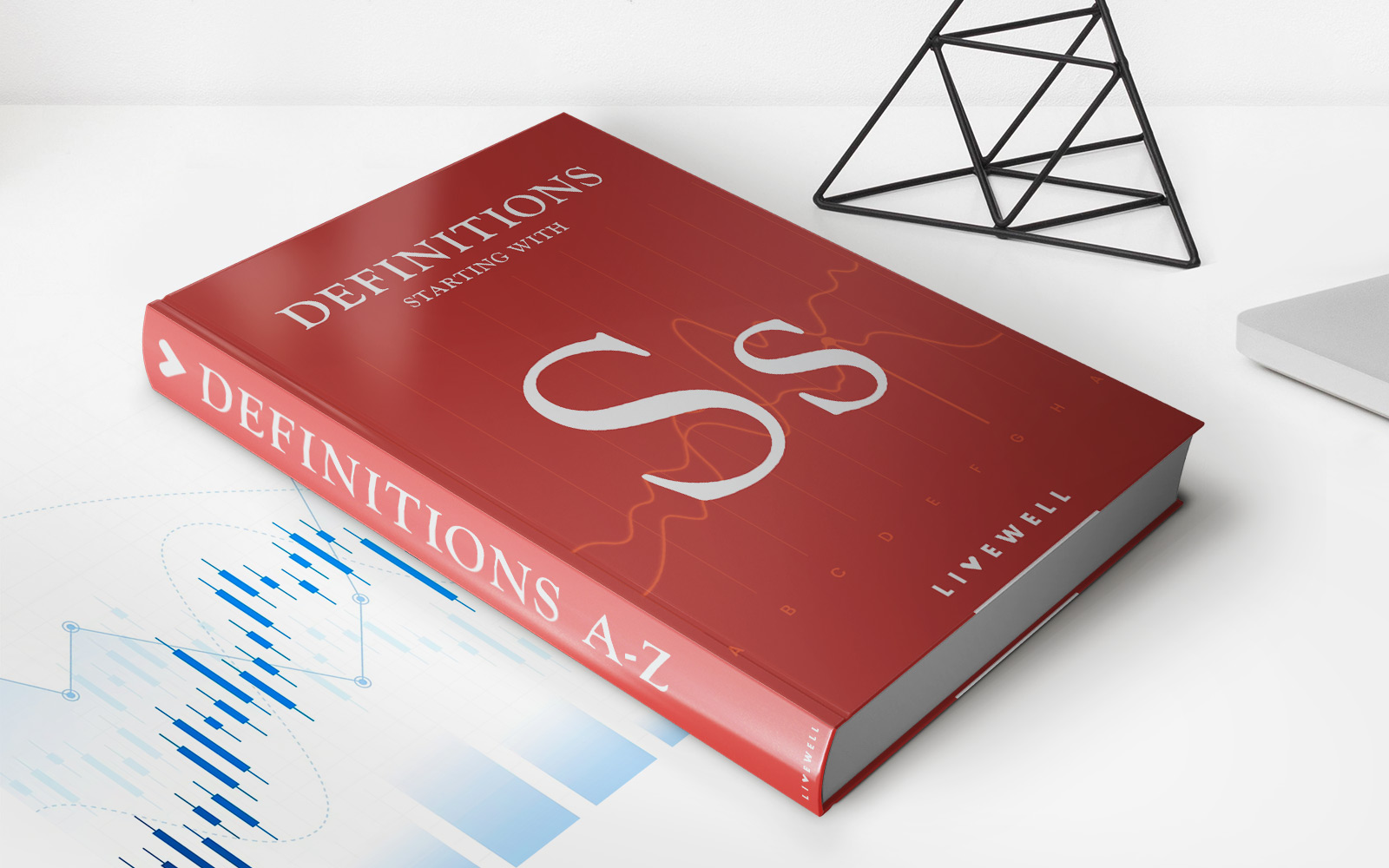
Finance
Serial Correlation: Definition, How To Determine, And Analysis
Published: January 27, 2024
Learn about serial correlation in finance, including its definition, how to determine it, and the analysis methods used to identify its presence.
(Many of the links in this article redirect to a specific reviewed product. Your purchase of these products through affiliate links helps to generate commission for LiveWell, at no extra cost. Learn more)
Serial Correlation: Definition, How to Determine, and Analysis
Welcome to our Finance category where we explore the fascinating world of numbers, trends, and analysis. In this blog post, we will delve into the concept of serial correlation – a key factor influencing financial data analysis. Ever wondered how to determine and analyze trends in your financial data? You’ve come to the right place! We will break down the definition of serial correlation, discuss how to determine it, and explore its impact on financial analysis.
Key Takeaways:
- Serial correlation refers to the relationship between consecutive observations in a dataset. It measures the degree to which the values in a dataset follow a pattern or show a trend over time.
- By determining the presence and strength of serial correlation in financial data, analysts can make more accurate predictions and identify potential opportunities or risks in the market.
What is Serial Correlation?
Serial correlation, also known as autocorrelation, is a statistical measure that helps analysts understand the relationship between consecutive observations in a dataset. It allows them to identify patterns, trends, and dependencies in the data over time. Understanding serial correlation is crucial for financial analysts as it helps them make informed decisions based on historical data and predict future market behavior.
Serial correlation is typically measured using correlation coefficients such as the Pearson correlation or the Durbin-Watson statistic. These measures range from -1 to 1, indicating the strength and direction of the correlation. A positive value indicates a positive correlation, meaning that consecutive data points tend to move together in the same direction. Conversely, a negative value indicates a negative correlation, suggesting an inverse relationship between consecutive data points.
How to Determine Serial Correlation?
- Plot your data: Start by visualizing your dataset using line graphs or scatter plots. Observing the plotted data can give you an initial sense of whether there might be serial correlation present.
- Calculate correlation coefficients: Use statistical measures such as the Pearson correlation or the Durbin-Watson statistic to determine the degree of serial correlation in your data. A correlation coefficient close to 1 or -1 suggests a strong serial correlation, while a value close to 0 indicates the absence of serial correlation.
- Perform hypothesis tests: Conduct hypothesis tests, such as the t-test or the chi-square test, to assess the statistical significance of the serial correlation. A significant result indicates that the observed serial correlation is unlikely due to chance alone, thus strengthening the validity of your findings.
Analysis and Implications
Serial correlation plays a significant role in financial data analysis, providing insights into trends, seasonality, and potential future market movements. Here are a few key implications:
- Financial Forecasting: By understanding serial correlation, analysts can make more accurate predictions about future market behavior. They can identify trends, cyclical patterns, and seasonality in the data, enabling them to forecast future prices or returns.
- Risk Management: Serial correlation analysis helps analysts identify patterns of positive or negative streaks in financial data. For example, detecting extended periods of positive serial correlation in stock prices might indicate bullish market behavior, while negative serial correlation could suggest bearish trends. By recognizing such patterns, investors can adjust their risk management strategies accordingly.
Serial correlation provides a powerful tool for financial analysts, allowing them to gain deeper insights into the behavior of financial data over time. By understanding the presence and strength of serial correlation, analysts can make more informed decisions, refine their forecasting models, and manage risks more effectively.
So, next time you dive into the world of financial data analysis, remember the importance of serial correlation. It could be the key to unlocking valuable insights and enhancing your financial decision-making process.