Home>Finance>Type 1 Error: Definition, False Positives, And Examples
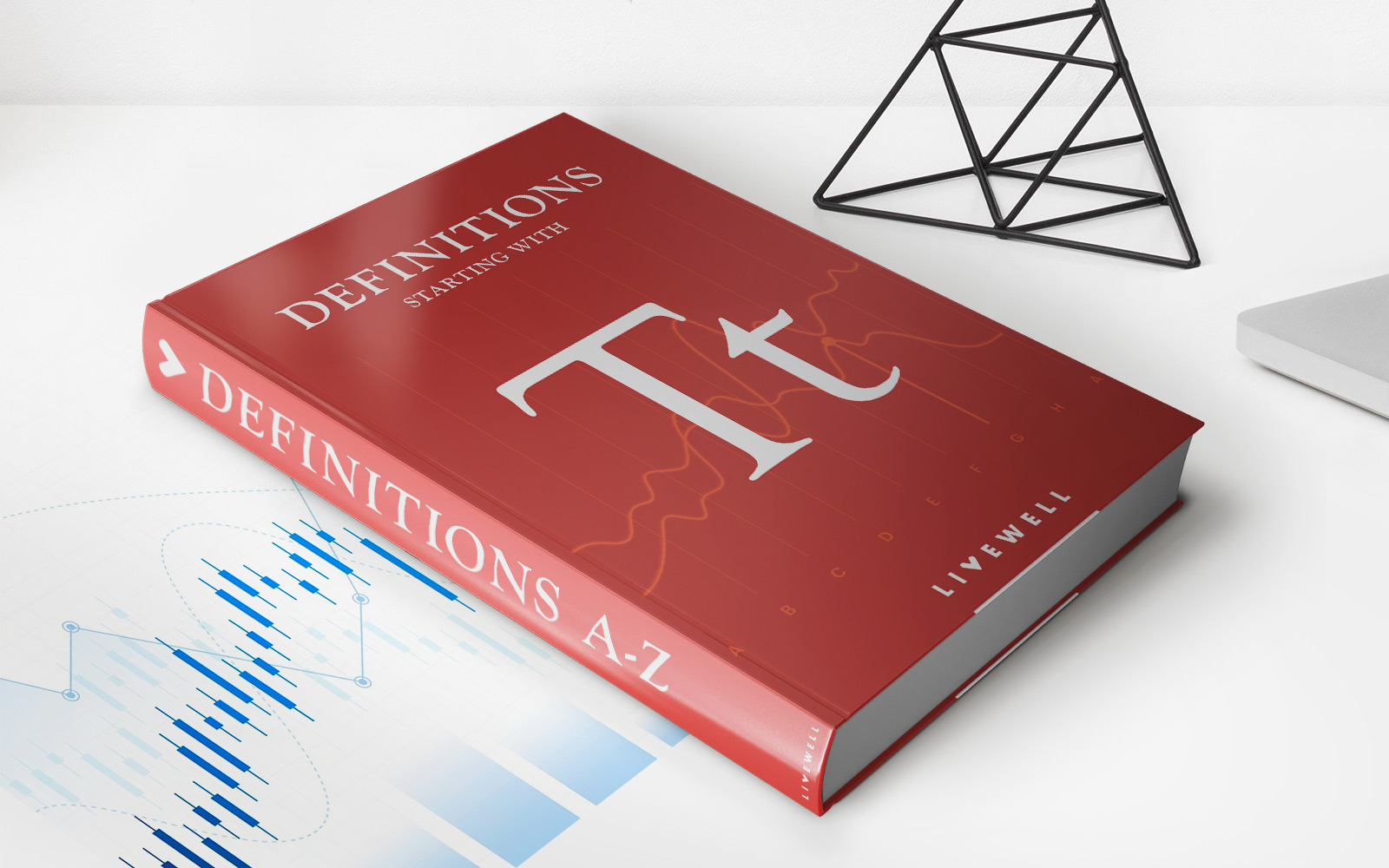
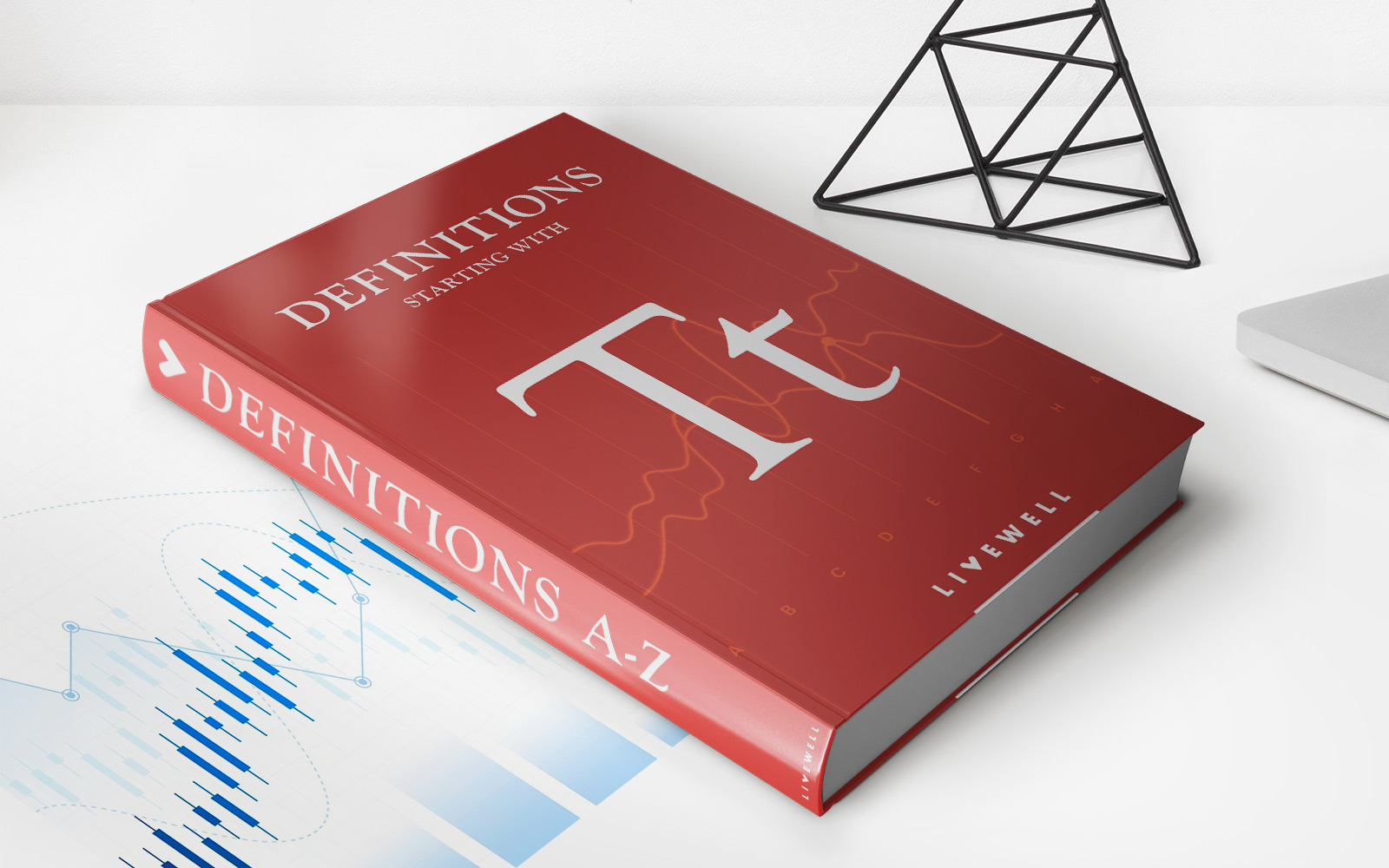
Finance
Type 1 Error: Definition, False Positives, And Examples
Published: February 12, 2024
Learn about type 1 errors in finance, including their definition, false positives, and examples. Gain insights into the significance of avoiding these errors in financial decision-making.
(Many of the links in this article redirect to a specific reviewed product. Your purchase of these products through affiliate links helps to generate commission for LiveWell, at no extra cost. Learn more)
Understanding Type 1 Error: The Sneaky False Positives
Imagine this scenario: You’re conducting a critical experiment to test a new drug that could potentially save lives. The results are in, and it appears that the drug is effective in treating the targeted condition. The excitement kicks in as you imagine the life-changing impact this discovery could have. But wait! Is the drug really effective, or is it just a Type 1 error?
Key Takeaways
- A Type 1 error occurs when a null hypothesis is mistakenly rejected.
- It leads to false positives, where a positive result is observed, but no effect or relationship exists.
In the field of statistics, a Type 1 error occurs when a null hypothesis is mistakenly rejected, meaning that there is a positive result when, in reality, no effect or relationship exists. In simple terms, it’s a false positive.
Let’s explore this concept further and understand why Type 1 errors can wreak havoc on scientific and statistical analysis.
Anatomy of a Type 1 Error
When conducting any experiment or test, researchers work with two opposing hypotheses:
- The null hypothesis (H0): This hypothesis suggests that there is no effect or relationship between variables. It assumes that any observed differences or correlations are due to random chance alone.
- The alternative hypothesis (Ha): This hypothesis suggests that there is an effect or relationship between variables. It proposes that the observed differences or correlations are not due to random chance but rather a genuine effect.
When analyzing the data obtained from an experiment, researchers set a critical value or threshold, known as the significance level (α). This threshold determines what level of evidence is required to reject the null hypothesis and accept the alternative hypothesis.
A Type 1 error occurs when the null hypothesis is rejected, even though it is true. In other words, it wrongly concludes that there is an effect or relationship when, in reality, there is none.
Real-World Examples of Type 1 Errors
To give you a clearer understanding of Type 1 errors, let’s explore a couple of real-life examples:
- A medical test for a rare disease: Imagine a medical test designed to detect a rare disease that only affects 0.1% of the population. The test is 99% accurate, meaning that it correctly identifies positive cases 99% of the time. However, it also has a 5% chance of producing a false positive result. Now, let’s assume that out of 1000 people, only one actually has the disease. If all 1000 people take the test, we would expect approximately 10 false positives (5% of the 999 healthy people). This shows how Type 1 errors can occur even when using seemingly reliable tests.
- Legal decisions: In a court of law, the presumption is that an individual is innocent until proven guilty. However, a Type 1 error, in this case, would occur if an innocent person is wrongly convicted. The Justice system acknowledges this risk and establishes strict protocols and standards of evidence to minimize the chances of a Type 1 error in criminal cases.
Type 1 errors can have significant consequences, leading to wasted resources, misinterpretation of data, and even disastrous medical or legal outcomes. Therefore, it is crucial for researchers, statisticians, and decision-makers to understand the concept of Type 1 errors and take appropriate measures to minimize their occurrence.
In Conclusion
Understanding the concept of Type 1 error is essential when analyzing data and test results. It serves as a reminder of the importance of applying critical thinking and statistical measures to avoid drawing false conclusions.
So, the next time you come across a positive result that seems too good to be true, remember the lurking presence of Type 1 errors and be cautious before accepting it at face value.