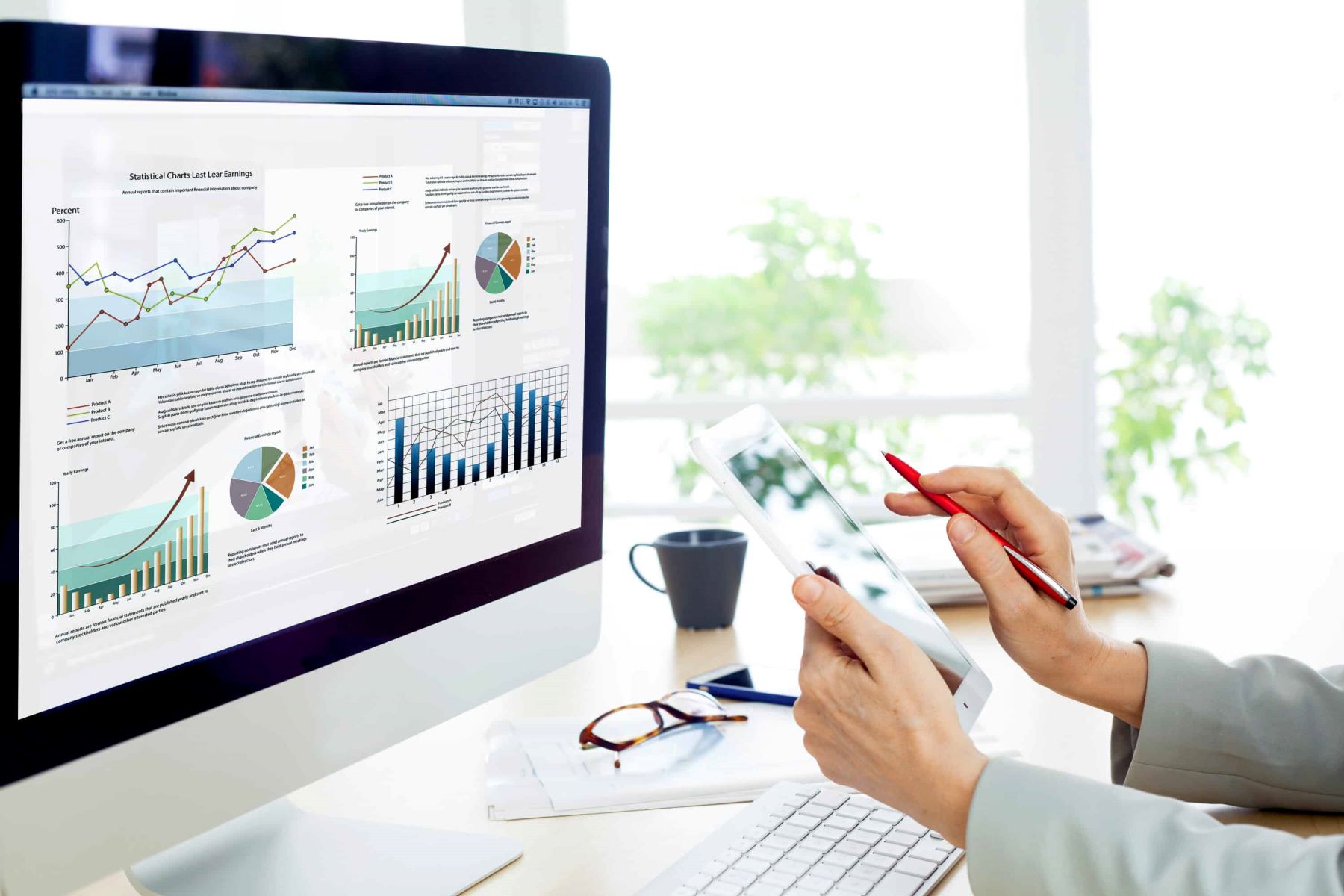
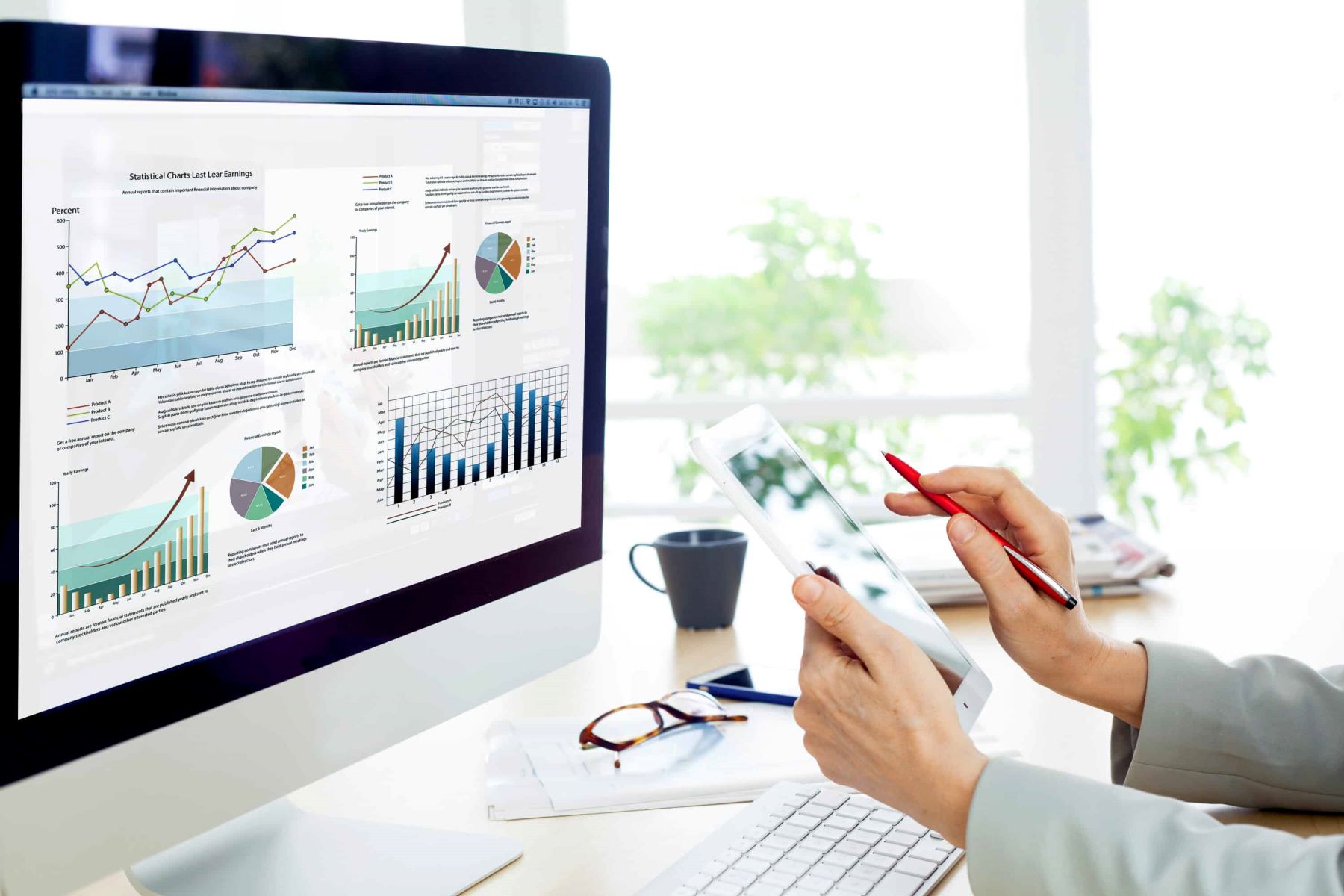
Finance
What Is Data Analytics In Accounting
Modified: February 21, 2024
Learn how data analytics is revolutionizing the field of accounting. Discover the powerful role it plays in finance and understand its impact on financial decision making.
(Many of the links in this article redirect to a specific reviewed product. Your purchase of these products through affiliate links helps to generate commission for LiveWell, at no extra cost. Learn more)
Table of Contents
- Introduction
- Definition of Data Analytics in Accounting
- Importance of Data Analytics in Accounting
- Benefits of Data Analytics in Accounting
- Challenges of Implementing Data Analytics in Accounting
- Best Practices for Data Analytics in Accounting
- Tools and Techniques for Data Analytics in Accounting
- Case Studies of Successful Data Analytics in Accounting
- Future Trends of Data Analytics in Accounting
- Conclusion
Introduction
Data analytics has become an essential tool in the field of accounting. With the increasing availability of vast amounts of financial data, organizations are now able to leverage advanced analytical techniques to gain valuable insights and make informed decisions. Data analytics in accounting involves the use of statistical models, algorithms, and other technological tools to extract meaningful patterns and trends from financial data.
Gone are the days of manual data entry and analysis, as businesses now have access to powerful software and platforms that can handle large datasets efficiently. These advancements not only streamline the accounting process but also enable accountants and financial professionals to uncover hidden insights and mitigate risks.
Accounting data analytics covers various aspects of financial management, including financial planning and analysis, risk assessment, fraud detection, and performance analysis. It helps organizations understand their financial health, identify areas of improvement, and make data-driven decisions to achieve their goals.
In this article, we will explore the definition, importance, benefits, challenges, best practices, tools and techniques, case studies, and future trends of data analytics in accounting. By the end, you will have a comprehensive understanding of how data analytics is transforming the accounting industry and how it can benefit your organization.
Definition of Data Analytics in Accounting
Data analytics in accounting refers to the use of advanced analytical techniques and tools to extract meaningful insights from financial data. It involves collecting, organizing, analyzing, and interpreting financial information to provide valuable insights and support decision-making processes.
Accounting data analytics utilizes statistical models, algorithms, and data visualization techniques to identify patterns, correlations, and trends within financial datasets. It goes beyond the traditional financial reporting and analysis methods by leveraging technology to uncover hidden insights and provide a more comprehensive understanding of an organization’s financial performance.
The main goal of data analytics in accounting is to make sense of the vast amounts of financial data generated by organizations. This data can include transactional records, financial statements, customer information, market data, and more. By analyzing this data, accountants and financial professionals can gain valuable insights into various aspects of the business, such as revenue growth, cost optimization, risk assessment, and fraud detection.
Data analytics in accounting is not limited to retrospective analysis; it also focuses on predictive and prescriptive analytics. Predictive analytics involves forecasting future trends and events based on historical data, while prescriptive analytics recommends optimal courses of action based on data analysis results.
With the advancement of technology, accounting data analytics has become more accessible and efficient. Automated accounting systems, data visualization tools, and machine learning algorithms enable accountants to process and analyze large volumes of data quickly and accurately. This allows for real-time monitoring and decision-making, leading to more agile and proactive financial management.
Importance of Data Analytics in Accounting
Data analytics plays a crucial role in modern accounting practices by revolutionizing the way financial data is interpreted and utilized. Here are some key reasons why data analytics is important in accounting:
1. Enhanced Financial Decision-making: Data analytics provides accountants and financial professionals with powerful insights that enable more informed decision-making. By analyzing financial data, organizations can identify trends, patterns, and potential risks, allowing them to make strategic decisions that drive financial growth and profitability.
2. Improved Efficiency: Manual data analysis can be time-consuming and prone to errors. Data analytics automates and streamlines the accounting process, reducing the need for manual data entry and analysis. This leads to increased productivity, accuracy, and efficiency in managing financial data.
3. Risk Management: Data analytics helps to identify and mitigate financial risks. By analyzing historical and real-time data, organizations can detect anomalies, patterns of fraudulent activities, and potential compliance violations. This ensures that proper internal controls are in place to minimize financial risks and protect the organization’s reputation.
4. Cost Optimization: Data analytics provides insights into cost drivers and expenditure patterns within an organization. By analyzing financial data, accountants can identify areas of inefficiency and implement cost-saving measures. This leads to better financial management and resources allocation, resulting in improved profitability.
5. Fraud Detection: Data analytics tools can detect suspicious transactions and patterns that indicate potential fraud. By analyzing financial data, accountants can identify anomalies, unusual behavior, and fraudulent activities. This helps organizations prevent financial losses and maintain the integrity of their financial statements.
6. Financial Planning and Forecasting: Data analytics provides the ability to project future financial outcomes based on historical data. By analyzing past trends and patterns, organizations can make accurate financial forecasts, create realistic budgets, and develop effective financial plans to achieve their goals.
7. Compliance and Regulation: With the increasing complexity of financial regulations, data analytics helps organizations ensure compliance with laws and regulations. By analyzing financial data, accountants can identify any discrepancies or non-compliant practices, allowing for timely corrective actions and reducing the risk of penalties or legal issues.
In summary, data analytics is essential in accounting as it enables organizations to make data-driven decisions, improve efficiency, manage risks, optimize costs, detect fraud, plan for the future, and ensure compliance. By leveraging the power of data, accounting professionals can become strategic partners in driving financial success for their organizations.
Benefits of Data Analytics in Accounting
Data analytics in accounting offers numerous benefits that revolutionize how financial data is analyzed and utilized. Let’s explore some of the key advantages:
1. Improved Decision-Making: Data analytics empowers accountants and financial professionals with valuable insights that enable more informed and strategic decision-making. By analyzing financial data, organizations can identify trends, patterns, and potential risks, allowing for better financial planning and resource allocation.
2. Increased Efficiency and Accuracy: Manual data entry and analysis can be time-consuming and prone to errors. Data analytics automates and streamlines these processes, reducing the risk of human error and increasing overall efficiency. This allows accountants to spend more time on value-added tasks, such as financial analysis and strategic planning.
3. Enhanced Risk Management: Data analytics helps organizations identify and mitigate financial risks. By analyzing historical and real-time data, accountants can detect anomalies, patterns of fraudulent activities, and potential compliance violations. This enables the implementation of proper internal controls to minimize financial risks and protect the organization’s reputation.
4. Cost Optimization: Data analytics provides insights into cost drivers and expenditure patterns within an organization. By analyzing financial data, accountants can identify areas of inefficiency, optimize costs, and implement cost-saving measures. This leads to improved financial management and better resource allocation.
5. Fraud Detection and Prevention: Data analytics tools can help detect suspicious transactions and patterns that indicate potential fraud. By analyzing financial data, accountants can identify anomalies, unusual behavior, and fraudulent activities. This allows organizations to take proactive measures to prevent financial losses and maintain the integrity of their financial statements.
6. Enhanced Financial Planning and Forecasting: Data analytics enables organizations to make accurate financial forecasts and projections based on historical data. By analyzing past trends and patterns, organizations can create realistic budgets, develop effective financial plans, and set achievable goals. This helps in better financial planning and enables organizations to adapt to market changes more effectively.
7. Compliance with Regulations: With the increasing complexity of financial regulations, data analytics helps organizations ensure compliance. By analyzing financial data, accountants can identify any discrepancies or non-compliant practices and take timely corrective actions. This reduces the risk of penalties or legal issues and ensures adherence to regulatory requirements.
8. Improved Stakeholder Communication: Data analytics provides visually engaging dashboards and reports that make it easier to communicate financial information to stakeholders. Visual representations of financial data help stakeholders understand complex financial concepts and make well-informed decisions.
9. Competitive Advantage: Organizations that effectively utilize data analytics in accounting gain a competitive edge. By leveraging the power of data, they can identify market trends, customer behavior, and emerging opportunities. This enables them to make proactive decisions and stay ahead of the competition.
Overall, data analytics in accounting offers significant benefits, including enhanced decision-making, increased efficiency and accuracy, improved risk management, cost optimization, fraud detection and prevention, better financial planning, regulatory compliance, improved stakeholder communication, and a competitive advantage. Organizations that embrace data analytics in their accounting practices can gain valuable insights and drive financial success.
Challenges of Implementing Data Analytics in Accounting
While data analytics in accounting offers numerous benefits, there are several challenges that organizations may encounter during implementation. These challenges should be considered and addressed to ensure the successful integration of data analytics into accounting practices. Let’s explore some of the main challenges:
1. Data Quality and Integrity: The accuracy and reliability of data are crucial for effective data analytics. Inaccurate or incomplete data can lead to misleading insights and flawed decision-making. Therefore, organizations must ensure data quality and integrity by implementing proper data governance practices, data validation processes, and data cleansing techniques.
2. Data Integration and Compatibility: Organizations often have data stored in various systems and formats. Integrating and analyzing data from different sources can be a challenge. This requires effective data integration strategies, data mapping, and ensuring compatibility between different systems and tools used for data analytics.
3. Data Security and Privacy: With large amounts of sensitive financial data being analyzed, data security and privacy are paramount. Organizations need to implement robust security measures, access controls, encryption techniques, and comply with data protection regulations to protect the confidentiality and integrity of financial data.
4. Skill Gap: Implementing data analytics in accounting requires professionals with the right skills and knowledge. However, there may be a shortage of skilled personnel who can effectively use data analytics tools and interpret the results. Organizations must invest in training and upskilling their accounting teams to bridge this skill gap.
5. Technical Infrastructure: Data analytics requires advanced technological infrastructure to handle large volumes of data and perform complex analyses. Organizations need to invest in appropriate hardware, software, and IT infrastructure to support data analytics initiatives. This includes considerations such as storage capacity, processing power, and network bandwidth.
6. Change Management: Implementing data analytics in accounting often involves significant changes in processes, workflows, and organizational culture. Resistance to change and lack of buy-in from stakeholders can hinder implementation efforts. Effective change management strategies, communication, and training are necessary to ensure successful adoption of data analytics.
7. Cost Considerations: Implementing data analytics in accounting can involve significant upfront costs. Organizations need to invest in infrastructure, software licenses, training, and hiring skilled professionals. It is important to weigh the potential benefits against the costs and develop a clear business case for implementing data analytics.
8. Scalability: As organizations grow, the volume and complexity of data also increase. Scaling up data analytics capabilities to handle larger datasets and more complex analyses can be a challenge. Organizations need to ensure their data analytics infrastructure and processes are scalable to meet future needs.
9. Regulatory and Ethical Considerations: The use of data analytics in accounting must comply with legal and ethical guidelines. Organizations need to ensure they are using data responsibly, protecting customer privacy, and adhering to applicable laws and regulations governing data usage.
By addressing these challenges, organizations can successfully implement data analytics in their accounting practices and harness the full potential of financial data to drive informed decision-making and financial success.
Best Practices for Data Analytics in Accounting
Implementing data analytics in accounting requires following best practices to ensure accurate and meaningful insights. Here are some key best practices for effective data analytics in accounting:
- Define Clear Goals: Clearly define the objectives and goals of the data analytics initiative in accounting. Identify the key questions to be answered and the problems to be solved. This will help focus the analysis and ensure that it aligns with the organization’s strategic priorities.
- Collect Relevant and Accurate Data: Ensure that the data collected for analysis is accurate, complete, and relevant to the objectives. This includes validating the data sources, cleaning data to remove inconsistencies, and addressing any data quality issues. Data accuracy is crucial for accurate and reliable insights.
- Use the Right Tools: Select the appropriate data analytics tools and technologies that align with the organization’s needs and goals. This may include using data visualization tools, statistical software, machine learning algorithms, and cloud-based analytics platforms. Choose user-friendly tools that can handle large datasets and provide the required functionalities.
- Implement Data Governance: Establish data governance policies and procedures to ensure data integrity, security, and compliance. Develop guidelines for data collection, storage, and usage. Implement access controls to protect sensitive financial information and ensure compliance with relevant regulations such as data privacy laws.
- Involve Stakeholders: Engage stakeholders such as accountants, finance professionals, and decision-makers from different departments in the data analytics process. Understand their needs, challenges, and expectations. Collaborate with stakeholders to define the scope of analysis and validate insights to ensure their relevance and usefulness.
- Perform Regular Data Analysis: Regularly analyze financial data to identify trends, patterns, and anomalies. This will help monitor financial performance, detect potential risks, and highlight opportunities for improvement. Perform both retrospective and real-time analysis to gain a comprehensive understanding of the financial situation.
- Adopt Data Visualization Techniques: Utilize data visualization techniques to present the analysis results in a clear and comprehensible manner. Visualizations such as charts, graphs, and dashboards can simplify complex financial information and facilitate better decision-making. Visualizations should be visually appealing, easy to interpret, and relevant to the analysis objectives.
- Continuously Improve: Data analytics is an ongoing process. Continuously review and refine the data analytics practices to improve accuracy, efficiency, and relevance. Seek feedback from users and stakeholders to identify areas for improvement. Keep up-to-date with emerging technologies and industry best practices to stay ahead of the curve.
- Ensure Ethical Data Usage: Adhere to ethical standards and legal requirements when using financial data for analysis. Obtain appropriate consent for data usage and ensure compliance with data privacy regulations. Protect the confidentiality, integrity, and privacy of financial information to maintain trust with stakeholders.
- Invest in Training and Skill Development: Provide training and skill development opportunities for accountants and finance professionals to enhance their data analytics capabilities. This includes technical training on data analytics tools, as well as developing critical thinking and data interpretation skills to derive meaningful insights from financial data.
By following these best practices, organizations can effectively leverage data analytics in accounting to improve decision-making, optimize financial performance, and gain a competitive edge in today’s data-driven business environment.
Tools and Techniques for Data Analytics in Accounting
Data analytics in accounting involves the use of various tools and techniques to extract valuable insights from financial data. Let’s explore some of the commonly used tools and techniques in data analytics:
1. Spreadsheet Software: Spreadsheet software such as Microsoft Excel is widely used in accounting for data analysis. It allows accountants to organize and manipulate financial data, perform calculations, and create visualizations using built-in functions and formulas. Excel is a versatile tool that can handle both small and large datasets.
2. Data Visualization Software: Data visualization tools like Tableau, Power BI, and Qlik allow accountants to create interactive and visually appealing dashboards, graphs, and charts. These tools enable the representation of complex financial data in a more understandable and engaging manner, making it easier to identify trends and patterns.
3. Statistical Analysis Software: Statistical analysis software such as SPSS and SAS are commonly used in accounting to analyze financial data. These tools provide advanced statistical techniques for data exploration, regression analysis, forecasting, and hypothesis testing. They enable accountants to perform in-depth statistical analysis and uncover meaningful insights.
4. Data Mining: Data mining techniques involve extracting patterns and relationships from large datasets. It is useful for identifying anomalies, fraud, and trends in financial data. Advanced data mining tools such as RapidMiner and KNIME use algorithms like association rules, clustering, and classification to uncover hidden insights in accounting data.
5. Machine Learning: Machine learning algorithms can be applied to financial data for predictive analytics and fraud detection. Techniques such as decision trees, random forests, and neural networks can analyze historical financial data to make accurate predictions and identify fraudulent transactions. Python libraries such as scikit-learn and TensorFlow are commonly used for implementing machine learning models in accounting.
6. Text Mining: Text mining techniques involve analyzing unstructured financial data such as financial reports, news articles, and customer feedback. It helps extract valuable insights and sentiments from textual data sources. Text mining tools like Natural Language Processing (NLP) algorithms can analyze financial reports to identify key trends and sentiments related to financial performance.
7. Robotic Process Automation (RPA): RPA uses software robots to automate repetitive and rule-based accounting tasks. It can extract data from multiple systems, perform data entry, reconcile accounts, and generate financial reports. RPA tools like UiPath and Automation Anywhere enhance efficiency and accuracy by reducing manual intervention and freeing accountants to focus on more value-added tasks.
8. Big Data Analytics: With the increasing volume and complexity of financial data, big data analytics tools are used to analyze large and diverse datasets. Technologies like Apache Hadoop and Apache Spark enable distributed processing of massive volumes of financial data. These tools can handle structured and unstructured data to extract insights quickly and effectively.
9. Cloud-based Analytics: Cloud-based analytics platforms like Microsoft Azure and Amazon Web Services (AWS) provide scalable and cost-effective solutions for data analytics in accounting. These platforms offer various analytics services and storage capabilities, allowing organizations to leverage powerful analytics capabilities without investing in on-premises infrastructure.
It’s essential to select the right tools and techniques based on the organization’s specific needs and goals. A combination of these tools and techniques, coupled with skilled data analysts and accountants, can maximize the potential of data analytics in accounting and drive better financial decision-making.
Case Studies of Successful Data Analytics in Accounting
Several organizations have successfully implemented data analytics in their accounting practices, leading to improved financial management, risk mitigation, and decision-making. Here are three case studies illustrating successful data analytics initiatives in accounting:
1. Company XYZ: Company XYZ, a global manufacturing company, implemented data analytics in their accounting department to streamline financial reporting and analysis. By leveraging data analytics tools and techniques, they were able to automate the extraction and consolidation of financial data from multiple subsidiaries and systems.
The organization created interactive dashboards that provided real-time insights into revenue, cost of goods sold, and operating expenses. These visualizations helped the finance team identify cost-saving opportunities and make data-driven decisions to improve profitability.
Data analytics also enabled the detection of fraudulent activities by identifying unusual patterns in financial transactions. This allowed Company XYZ to take proactive measures to mitigate the risks and strengthen their internal controls.
2. Company ABC: Company ABC, a retail chain, utilized data analytics in their accounting department to optimize inventory management and pricing strategies. By analyzing sales data, customer purchasing patterns, and inventory levels, they were able to identify slow-moving inventory and make data-driven decisions for inventory replenishment.
Data analytics also helped Company ABC understand customer behavior and preferences. By analyzing customer data, they were able to personalize marketing campaigns and promotions, resulting in higher customer engagement and increased sales.
The implementation of data analytics in pricing strategies allowed Company ABC to optimize their product pricing based on market demand, competitor analysis, and customer segmentation. This resulted in improved profit margins and enhanced competitiveness in the market.
3. Company PQR: Company PQR, a financial services firm, implemented data analytics in their accounting practices to enhance fraud detection and compliance efforts. By analyzing transactional data, customer behavior patterns, and historical fraud cases, they were able to develop advanced fraud detection models.
These models utilized machine learning algorithms to identify anomalies and suspicious activities in real-time. Through automated monitoring of financial transactions, Company PQR could quickly identify and investigate potential fraudulent activities, significantly reducing financial losses and reputational risks.
Data analytics also played a crucial role in regulatory compliance for Company PQR. By analyzing financial data, they were able to ensure adherence to anti-money laundering (AML) and know your customer (KYC) regulations. They could identify high-risk customers, monitor their transactions, and generate accurate reports for regulatory audits.
These case studies demonstrate how organizations across different industries have successfully implemented data analytics in their accounting practices. By leveraging the power of data, these organizations achieved improved financial management, enhanced decision-making, and reduced risks.
It’s important to note that the success of these initiatives was not only due to the use of data analytics tools but also the effective collaboration between accountants, data analysts, and other stakeholders. A combination of skilled personnel, relevant data, and appropriate tools is crucial for achieving successful outcomes in data analytics implementation.
Future Trends of Data Analytics in Accounting
Data analytics in accounting is constantly evolving, driven by advancements in technology and the changing business landscape. Here are some key future trends that are expected to shape the field of data analytics in accounting:
1. Artificial Intelligence (AI) and Machine Learning (ML): AI and ML technologies will continue to play a significant role in data analytics in accounting. These technologies can analyze large volumes of financial data, identify patterns, and make accurate predictions. AI-powered chatbots may also be used to assist with financial inquiries, automating routine tasks and enhancing customer service.
2. Natural Language Processing (NLP): NLP techniques will enable accountants to extract insights from unstructured financial data, such as financial statements, contracts, and regulatory filings. By using NLP algorithms, accountants can analyze textual data to gain insights and identify potential risks or opportunities.
3. Cloud Computing: The adoption of cloud-based analytics platforms will continue to grow in accounting. Cloud computing offers scalability, cost-efficiency, and easy access to data analytics tools and resources. It enables organizations to leverage powerful analytics capabilities without the need for extensive on-premises infrastructure.
4. Blockchain Technology: Blockchain technology has the potential to transform accounting practices by providing secure and transparent financial transactions. Accountants can leverage blockchain to ensure the accuracy and integrity of financial data, streamline audits, and enhance fraud detection and prevention.
5. Predictive Analytics: The focus on predictive analytics will grow, enabling accountants to forecast future financial outcomes based on historical data. Advanced predictive models can help organizations anticipate market trends, customer behavior, and potential risks, allowing for more proactive decision-making and strategic planning.
6. Real-time Financial Reporting: Real-time financial reporting will become more prevalent, enabling organizations to have up-to-date insights into their financial performance. Advances in data analytics tools and technologies will allow for faster processing and analysis of financial data, facilitating real-time decision-making.
7. Integration of External Data Sources: Organizations will increasingly integrate external data sources, such as social media data, market data, and economic indicators, with their internal financial data. This integration will provide a more comprehensive view of the business environment and enable better-informed decision-making.
8. Data Ethics and Governance: As the importance of data ethics becomes more apparent, organizations will focus on implementing robust data governance frameworks to ensure responsible and ethical use of financial data. Privacy, security, and compliance with data protection regulations will be prioritized in data analytics practices.
9. Visualization and Storytelling: Data visualization techniques will continue to evolve, allowing accountants to present financial insights in a more engaging and compelling manner. Interactive dashboards, infographics, and storytelling techniques will be utilized to communicate complex financial information effectively to stakeholders.
These future trends indicate a continuing shift towards more advanced and integrated data analytics practices in accounting. Organizations that stay updated with these trends and embrace emerging technologies will gain a competitive advantage in financial management, decision-making, and risk mitigation.
Conclusion
Data analytics has become a game-changer in the field of accounting, revolutionizing financial management, decision-making, and risk mitigation. By leveraging advanced analytical techniques and tools, organizations can extract valuable insights from financial data and translate them into actionable strategies.
In this article, we explored the definition of data analytics in accounting and highlighted its importance in enhancing financial decision-making, improving efficiency, managing risks, and ensuring compliance. We discussed the benefits of data analytics, including improved efficiency, enhanced risk management, cost optimization, fraud detection, and better financial planning and forecasting.
We also addressed the challenges that organizations may face when implementing data analytics in accounting, such as data quality, data integration, skill gaps, and change management. By understanding and addressing these challenges, organizations can pave the way for successful implementation and utilization of data analytics.
Moreover, we discussed best practices for data analytics in accounting, emphasizing the need to define clear goals, collect relevant and accurate data, use the right tools, involve stakeholders, and continuously improve processes. Implementing these best practices will ensure accurate and meaningful insights that drive informed decision-making.
Furthermore, we explored various tools and techniques used in data analytics, including spreadsheet software, data visualization software, statistical analysis software, and machine learning algorithms. These technological advances empower accountants and financial professionals to leverage the power of data and extract valuable insights from financial information.
Lastly, we delved into case studies that showcased successful data analytics initiatives in accounting, demonstrating how organizations across different industries have achieved improved financial management, optimized inventory, enhanced fraud detection, and compliance efforts.
Looking forward, we discussed future trends of data analytics in accounting, such as artificial intelligence, blockchain technology, predictive analytics, and real-time financial reporting. These trends will shape the future of accounting and offer opportunities for organizations to leverage data analytics for strategic financial success.
In conclusion, data analytics is transforming the accounting landscape, enabling organizations to gain deeper insights into financial data, make informed decisions, and optimize financial performance. By embracing data analytics and adopting best practices and emerging trends, organizations can unlock the full potential of financial data, gaining a competitive edge in today’s data-driven business world.