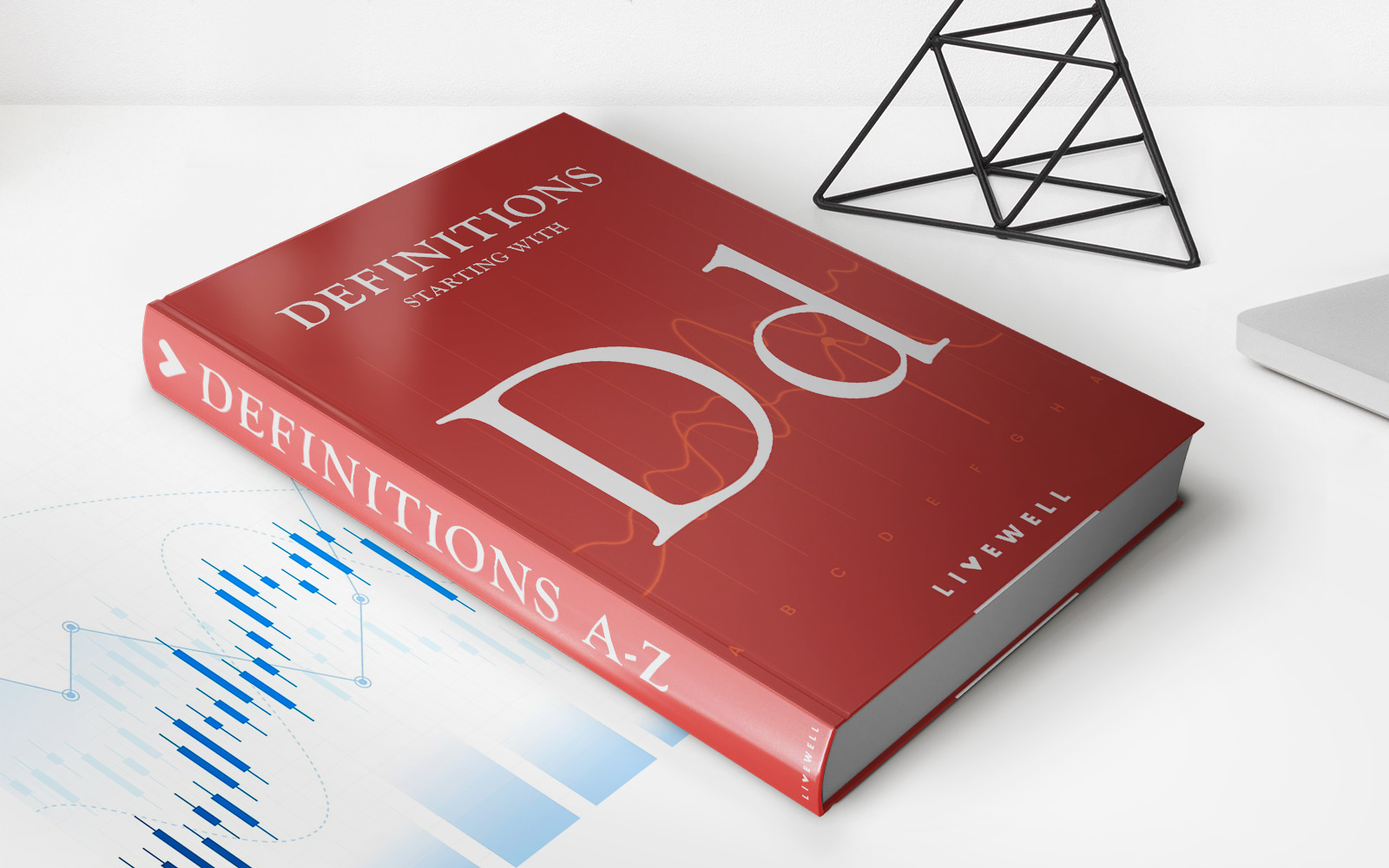
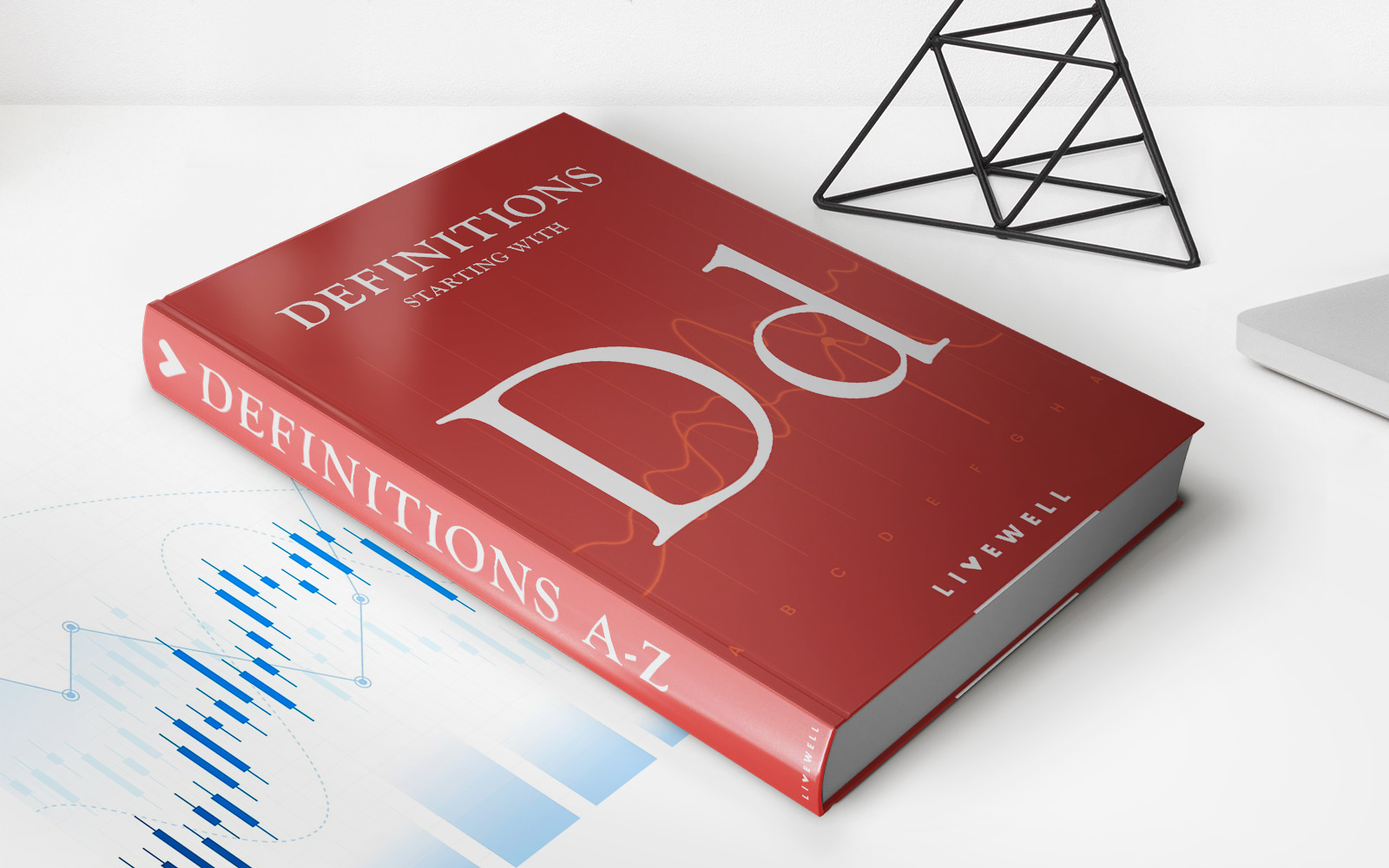
Finance
Data Smoothing: Definition, Uses, And Methods
Published: November 7, 2023
Learn about data smoothing in finance, including its definition, uses, and various methods. Enhance your financial analysis and decision-making with this essential technique.
(Many of the links in this article redirect to a specific reviewed product. Your purchase of these products through affiliate links helps to generate commission for LiveWell, at no extra cost. Learn more)
Finance 101: A Guide to Data Smoothing
Welcome to the Finance section of our blog! In this article, we will dive into the fascinating world of Data Smoothing. Whether you’re a financial analyst, a business owner, or just someone interested in understanding the ins and outs of finance, data smoothing is a concept that you’ll want to familiarize yourself with. So, what exactly is data smoothing, and how can it be used?
Key Takeaways:
- Data smoothing involves removing noise or irregularities from a dataset to reveal underlying trends or patterns.
- It is commonly used in finance for forecasting, trend analysis, and creating more accurate financial models.
Understanding Data Smoothing
Data smoothing is a statistical technique that aims to remove the random fluctuations or noise from a dataset, making it easier to identify underlying trends or patterns. It helps to reveal the true nature of the data by eliminating short-term irregularities and highlighting long-term movements. By smoothing out the data, analysts can gain a clearer picture of the overall trend and make more informed decisions.
Data smoothing is particularly valuable in finance, where accurate predictions and trend analysis play a crucial role in decision-making. It allows financial professionals to make reliable forecasts, identify potential risks, and create more robust financial models. Whether you’re dealing with stock prices, sales figures, or economic indicators, data smoothing can help enhance the accuracy of your analysis.
Why is data smoothing important in finance?
In the finance world, data is constantly subject to noise and fluctuations. These fluctuations can be caused by various factors, such as market volatility, seasonal variations, or one-time events. Data smoothing helps to filter out these short-term disturbances, allowing analysts to focus on the long-term trends and patterns that provide valuable insights.
Methods of Data Smoothing
There are several methods of data smoothing that analysts can employ, depending on the nature of the data and the desired outcomes. Here are some commonly used techniques:
- Moving Averages: This method calculates the average value of a set number of previous data points, creating a smoothed trend line. It is simple to use and can effectively eliminate short-term fluctuations.
- Exponential Smoothing: This technique assigns exponentially decreasing weights to older data points, giving more importance to recent observations. It is especially useful for time series data.
- Loess Smoothing: This non-parametric technique fits local regression curves to subsets of the data, allowing for flexible smoothing without assuming a specific relationship.
- Savitzky-Golay Filters: This method applies polynomial regression to a moving window of data points, effectively smoothing out the dataset.
Each method has its strengths and weaknesses, and the choice of technique depends on the specific requirements of the analysis. Financial analysts often experiment with different smoothing techniques to find the one that best fits their data and objectives.
Conclusion
Data smoothing is an essential tool in the world of finance. By removing the noise and irregularities from datasets, it allows analysts to uncover valuable insights and make more accurate predictions. Whether you’re analyzing stock prices, sales data, or economic indicators, data smoothing can help you identify trends and patterns that might otherwise go unnoticed.
As with any statistical technique, it’s important to understand the limitations and assumptions underlying data smoothing methods. Choosing the right technique and interpreting the results correctly are crucial for gaining meaningful insights. So, the next time you’re delving into financial analysis, don’t forget to apply data smoothing techniques for a clearer view of the data.
Stay tuned for more finance-related articles in our blog. Happy smoothing!