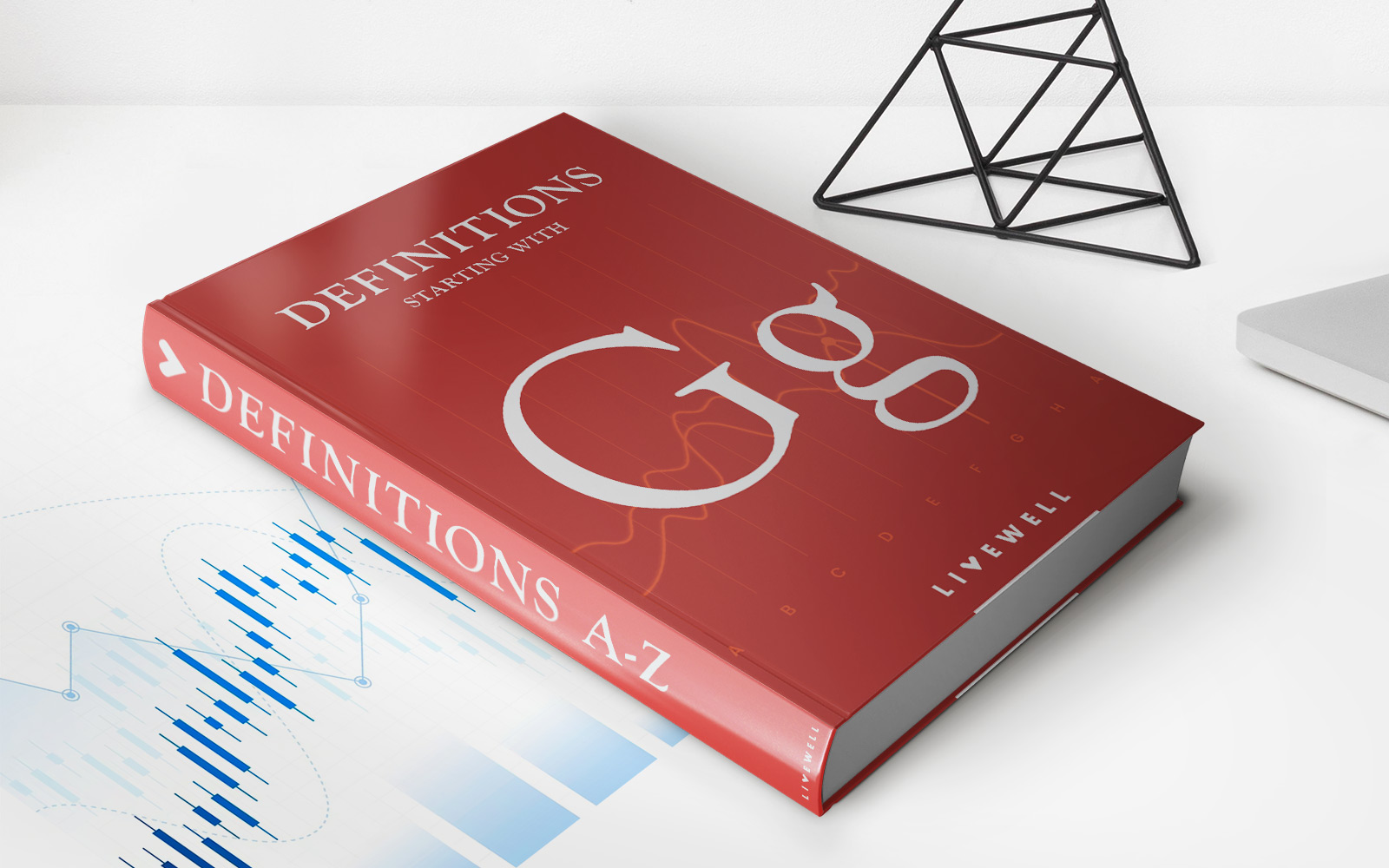
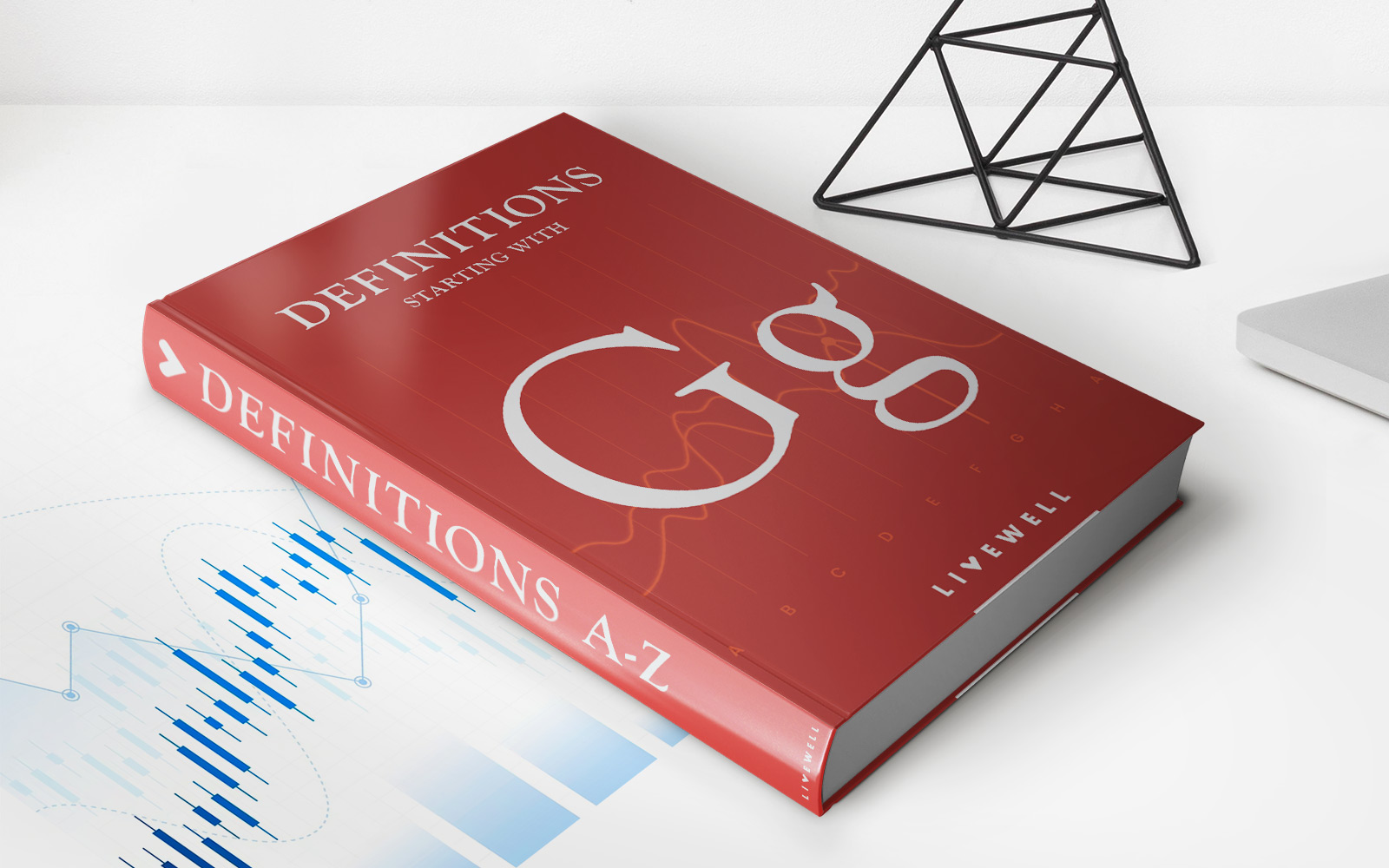
Finance
GARCH Model: Definition And Uses In Statistics
Published: November 30, 2023
Learn what a GARCH model is and how it is used in the field of finance. Understand its definition, applications, and significance in statistics.
(Many of the links in this article redirect to a specific reviewed product. Your purchase of these products through affiliate links helps to generate commission for LiveWell, at no extra cost. Learn more)
The GARCH Model: Unlocking the Secrets of Statistical Analysis
Have you ever wondered how analysts predict and measure volatility in financial markets? The GARCH model is an incredibly powerful tool that is widely used in statistics to analyze and forecast volatility. In this article, we will explore the definition of the GARCH model and delve into its various uses and applications. So, let’s dive in and uncover the fascinating world of GARCH!
Key Takeaways:
- The GARCH model is a statistical model used to analyze and forecast volatility in financial markets.
- Volatility measures the degree of variation or uncertainty in market prices.
What is the GARCH Model?
The Generalized Autoregressive Conditional Heteroskedasticity (GARCH) model is a popular statistical model used to analyze and forecast time series data with heteroskedasticity. In simpler terms, it helps us understand and predict volatility – the degree of variation or uncertainty – in financial markets. Volatility is a crucial aspect of financial analysis as it can significantly impact investment decisions.
How Does the GARCH Model Work?
The GARCH model combines Autoregressive (AR) and Moving Average (MA) techniques with Conditional Heteroskedasticity (ARCH) to capture the time-varying volatility patterns in financial data. By incorporating lagged values of the squared residuals, the model allows for the dependence of current market volatility on past volatilities and shocks, making it ideal for capturing the clustering and persistence of volatility.
The GARCH model is defined by two main components:
- ARCH Component: This component accounts for the lagged squared residuals and captures the volatility clustering phenomenon. It ensures that high volatility periods are followed by high volatility periods and vice versa.
- GARCH Component: This component includes the lagged conditional variances and helps capture the persistence of volatility. The GARCH component allows for the inclusion of previous volatility shocks, enabling the model to accurately capture and predict future volatility patterns.
Applications of the GARCH Model
The GARCH model finds extensive applications in various fields, particularly in finance and economics. Here are some key areas where the GARCH model is commonly utilized:
- Risk Management: The GARCH model is a powerful tool for assessing and managing financial risk. By accurately predicting volatility, it helps investors and financial institutions make informed decisions to mitigate risk and optimize investment portfolios.
- Option Pricing: Volatility plays a crucial role in option pricing models. The GARCH model’s ability to accurately forecast future volatility is instrumental in pricing options, providing valuable insights for traders and investors.
- Portfolio Optimization: The GARCH model’s volatility forecasts act as key inputs in portfolio optimization strategies. It helps investors determine the optimal allocation of assets to maximize returns while considering risk tolerance.
- Forecasting Financial Returns: By capturing and modeling volatility patterns, the GARCH model can be used to forecast future financial returns. These forecasts are essential for financial planning, trend analysis, and risk assessment.
It’s important to note that while the GARCH model is widely regarded as a powerful analytical tool, it has limitations as well. The model assumes that volatility follows a specific pattern and does not account for all unforeseen events or outliers. Therefore, it is essential to interpret the results with caution and consider other factors that may impact market volatility.
In Conclusion
The GARCH model is an indispensable tool for analyzing and forecasting volatility in financial markets. Its ability to capture the complex dynamics of market volatility makes it a go-to choice for risk managers, investors, and financial analysts. By incorporating autoregressive and moving average components with conditional heteroskedasticity, the GARCH model unlocks insights into the clustering, persistence, and future behavior of volatility. So, the next time you come across predictions or assessments of market volatility, you now have a deeper understanding of the underlying statistical model at work!