Home>Finance>Hypothesis To Be Tested: Definition And 4 Steps For Testing With Example
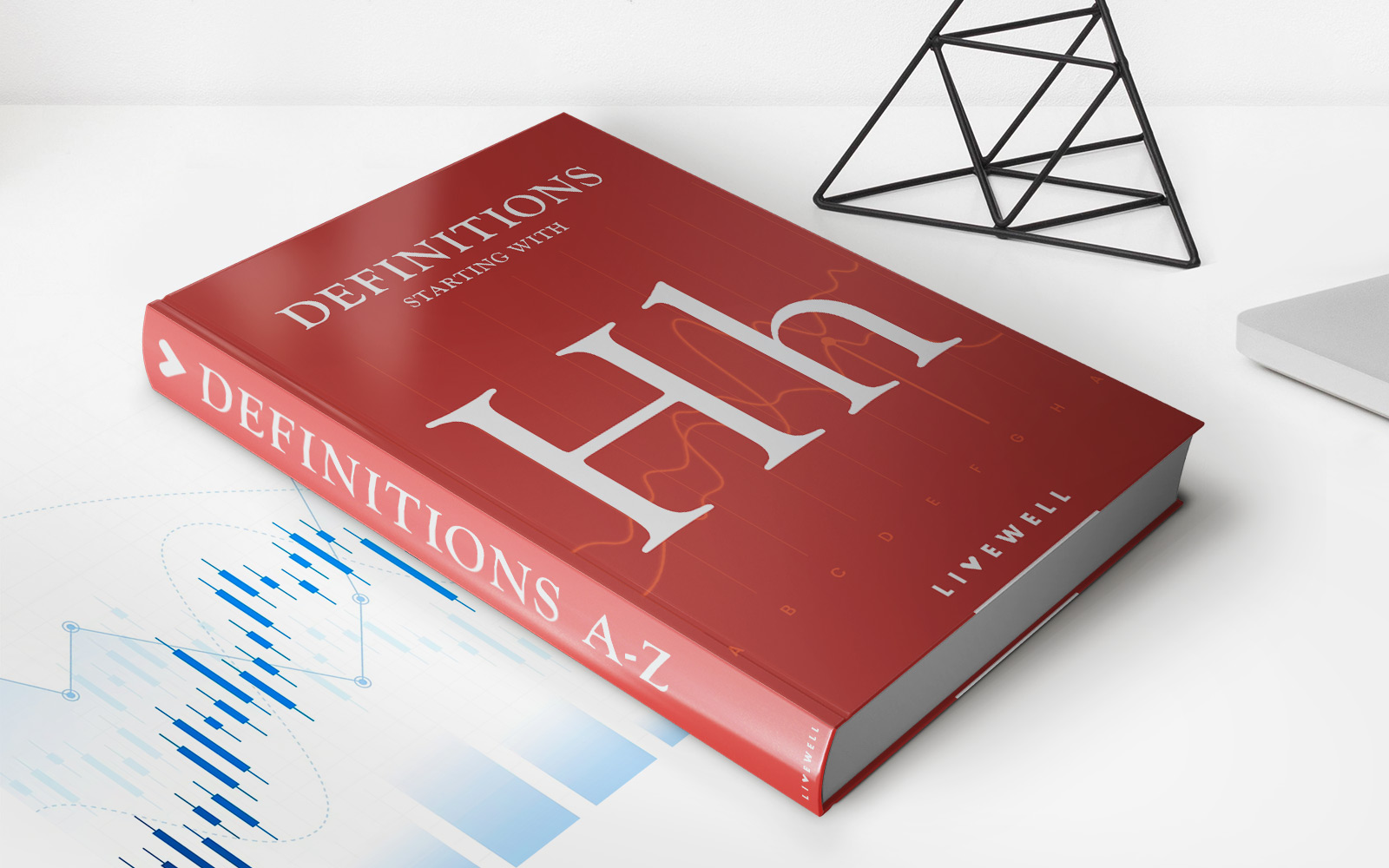
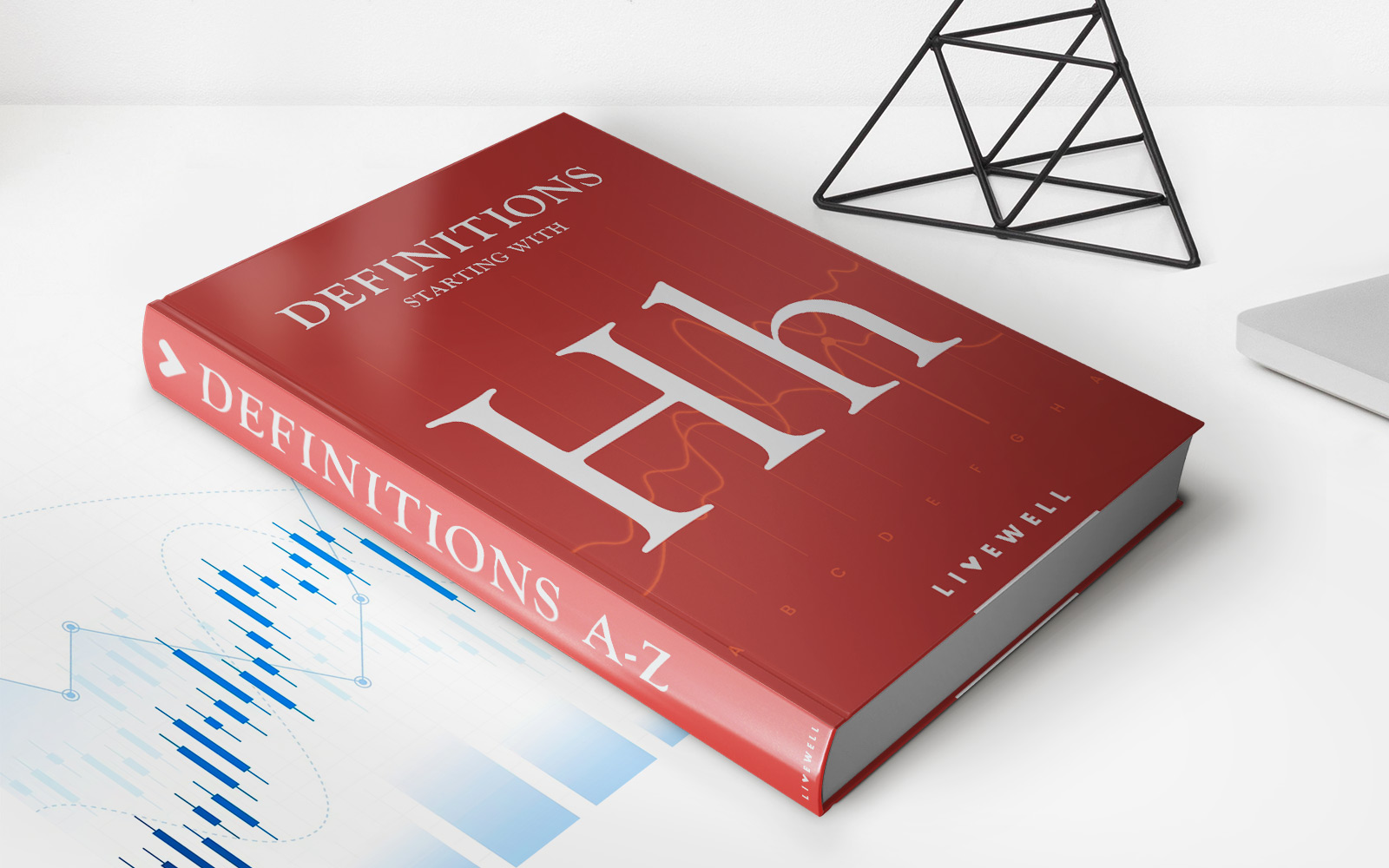
Finance
Hypothesis To Be Tested: Definition And 4 Steps For Testing With Example
Published: December 6, 2023
Learn about the definition of a hypothesis to be tested and the 4 steps involved in testing it, with a practical example in finance.
(Many of the links in this article redirect to a specific reviewed product. Your purchase of these products through affiliate links helps to generate commission for LiveWell, at no extra cost. Learn more)
Unlocking the Secrets of Hypothesis Testing in Finance: A Step-by-Step Guide
Greetings, finance enthusiasts! Today, we dive into the fascinating world of hypothesis testing – a powerful tool used by financial analysts and researchers to make informed decisions and drive data-driven strategies. In this blog post, we’ll explore the definition of hypothesis testing, outline the four crucial steps involved, and provide a real-life example to help you better understand its application in the financial realm.
Key Takeaways:
- Hypothesis testing is a statistical method used to validate or reject assumptions made about a specific population based on sample data.
- The four steps of hypothesis testing include stating the hypothesis, setting the significance level, calculating the test statistic, and interpreting the results to make a conclusion.
What is Hypothesis Testing?
In finance, where decisions can have significant financial implications, hypothesis testing plays a vital role in minimizing risks and maximizing returns. Put simply, hypothesis testing is a statistical method used to validate or reject assumptions made about a specific population based on sample data. By applying this method, financial analysts can make confident predictions or decisions based on the available evidence.
Now, let’s dive into the four essential steps involved in hypothesis testing:
Step 1: State the Hypothesis
The first step in hypothesis testing is to clearly state the null hypothesis (H0) and the alternative hypothesis (Ha). The null hypothesis represents the assumption we want to test, while the alternative hypothesis suggests an alternate outcome or direction. For example, in finance, we might want to test whether a particular investment strategy outperforms the market average (null hypothesis) or if it underperforms (alternative hypothesis).
Step 2: Set the Significance Level
The significance level (α) represents the probability of rejecting the null hypothesis when it is true. Typically, it is set at 0.05, indicating a 5% chance of making a Type I error (rejecting the null hypothesis when it is actually true). The significance level helps us determine the critical value or test statistic necessary for making a decision.
Step 3: Calculate the Test Statistic
The test statistic is a numerical value that summarizes the sample data and allows us to compare it with the expected population values. The choice of test statistic depends on the nature of the hypothesis being tested. Commonly used test statistics in finance include t-tests, z-tests, and chi-square tests. By calculating the test statistic, we can quantify the likelihood of observing the sample data given the null hypothesis.
Step 4: Interpret the Results and Make a Conclusion
Finally, after calculating the test statistic, we compare it with the critical value associated with our chosen significance level. If the test statistic falls within the critical region, we reject the null hypothesis in favor of the alternative. Conversely, if it falls outside the critical region, we fail to reject the null hypothesis. By interpreting the results, we can draw conclusions and make data-driven decisions in the financial domain.
Let’s illustrate these steps with a real-life example: Suppose a finance researcher wants to determine if a new investment strategy results in a significantly higher average return than the market average. Here’s how they would go about testing this hypothesis:
- Hypotheses: The null hypothesis (H0) would state that the new investment strategy’s average return is equal to the market average, while the alternative hypothesis (Ha) would state that the new strategy’s average return is higher.
- Significance Level: Set α at 0.05.
- Test Statistic: Calculate the t-statistic by comparing the sample average return with the market average return, considering sample size and variability.
- Interpret the Results: If the calculated t-statistic falls within the critical region, we reject the null hypothesis, indicating that the new investment strategy indeed results in a significantly higher average return.
By following these four steps, financial analysts can leverage hypothesis testing to gain insights, validate assumptions, and make informed decisions, ultimately leading to financial success.
Understanding the power of hypothesis testing is crucial to thriving in the ever-changing landscape of finance. Incorporating this statistical tool enables finance professionals to analyze data effectively, manage risks, and confidently navigate the complex world of investments. So, embrace the power of hypothesis testing and unlock a world of possibilities in finance!