Home>Finance>Log-Normal Distribution: Definition, Uses, And How To Calculate
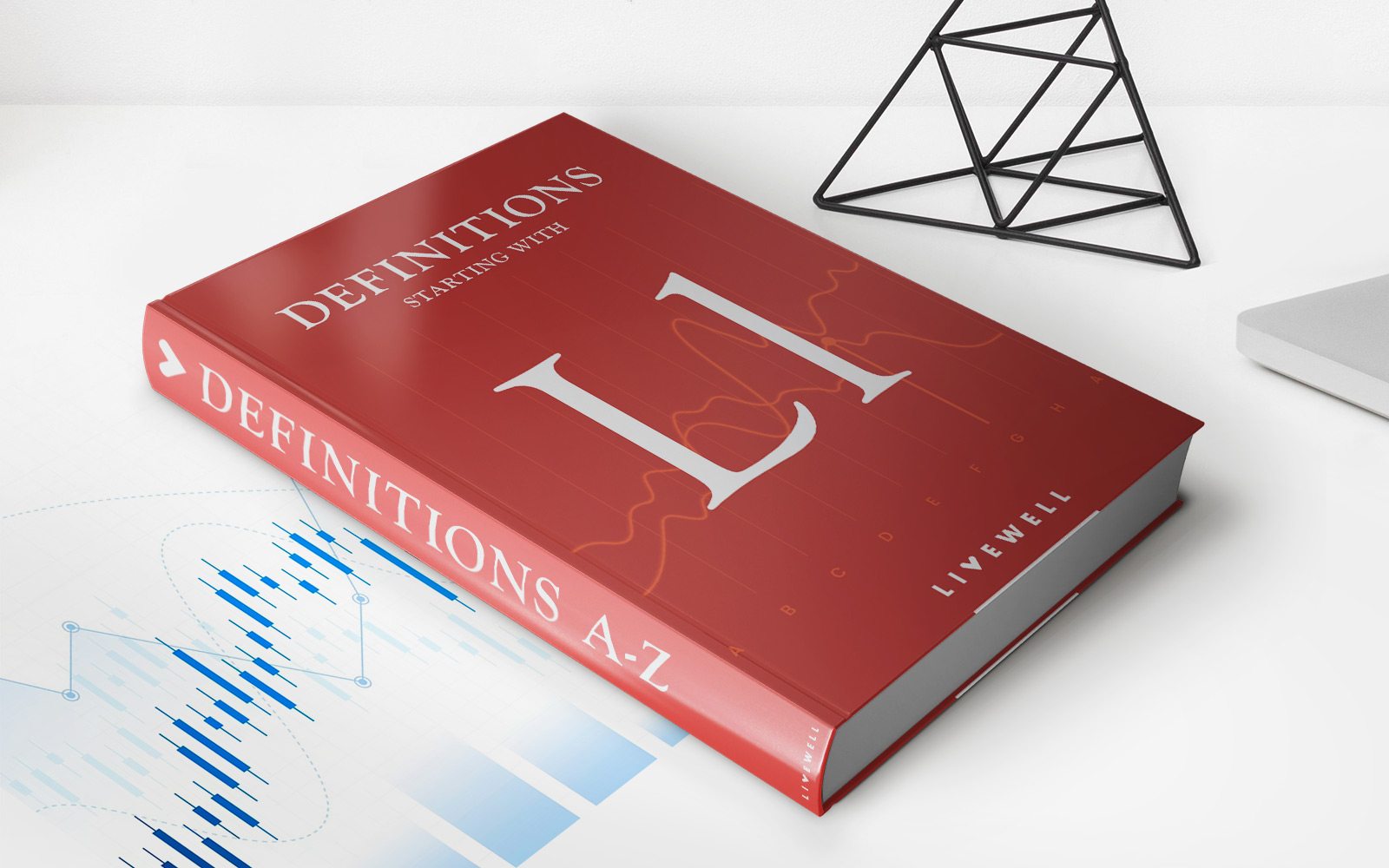
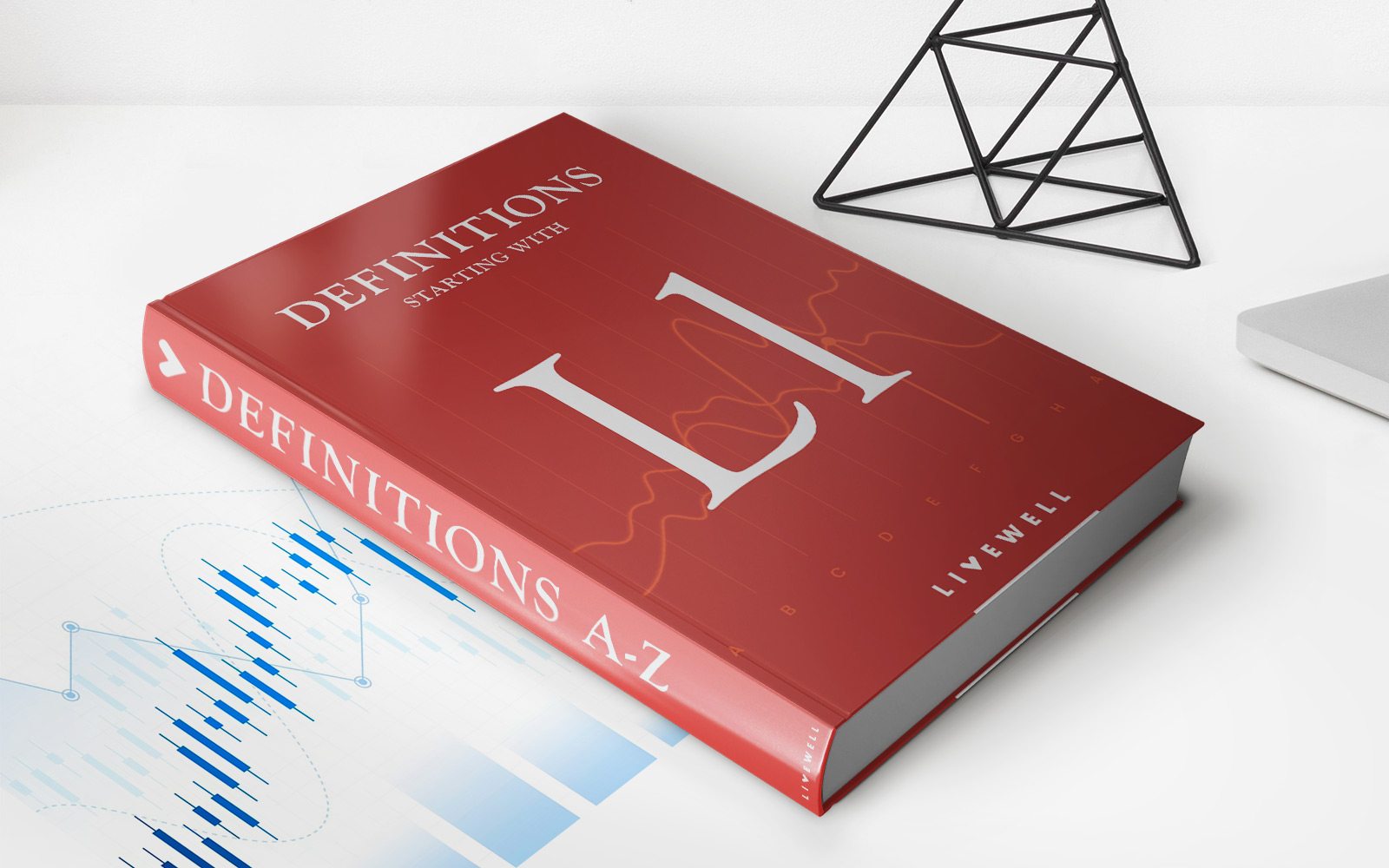
Finance
Log-Normal Distribution: Definition, Uses, And How To Calculate
Published: December 19, 2023
Learn about the log-normal distribution in finance, including its definition, uses, and how to calculate it.
(Many of the links in this article redirect to a specific reviewed product. Your purchase of these products through affiliate links helps to generate commission for LiveWell, at no extra cost. Learn more)
What is a Log-Normal Distribution?
A Log-Normal distribution is a probability distribution that resembles a normal distribution, but with one key difference. While normal distributions are symmetrical, log-normal distributions are positively skewed. In other words, log-normal distributions have a longer tail on the right side, indicating the potential for extreme positive outcomes.
Key Takeaways:
- A Log-Normal distribution is a probability distribution that is positively skewed.
- Unlike a normal distribution, which is symmetrical, log-normal distributions have a longer tail on the right side.
The log-normal distribution gets its name because it is the distribution of the logarithm of a random variable that follows a normal distribution. This means that if we have a random variable X that follows a log-normal distribution, the natural logarithm of X, denoted as ln(X), will follow a normal distribution.
The log-normal distribution is commonly used to model variables that are always positive and have a wide range of possible values. Some examples of variables that can be modeled using a log-normal distribution include:
- Stock prices: The prices of stocks in the financial markets often follow a log-normal distribution.
- Income distribution: The distribution of income in a population is often modeled using a log-normal distribution.
- Size of earthquakes: The magnitude of earthquakes is often modeled using a log-normal distribution.
- Population sizes: The sizes of populations in biology and ecology can often be modeled using a log-normal distribution.
Now that we understand what a log-normal distribution is and its various uses, let’s dive into how to calculate it.
How to Calculate a Log-Normal Distribution
To calculate a log-normal distribution, you’ll need a few key parameters:
- Mean (µ): The mean of the underlying normal distribution.
- Standard deviation (σ): The standard deviation of the underlying normal distribution.
Once you have these parameters, you can use the following formulas to calculate the probability density function (PDF) and cumulative distribution function (CDF) of the log-normal distribution:
PDF: f(x) = (1 / (xσsqrt(2π))) * exp(-(ln(x) – µ)² / (2σ²))
CDF: F(x) = 0.5 * (1 + erf((ln(x) – µ) / (σsqrt(2))))
Where:
- x = the value at which you want to evaluate the PDF or CDF
- exp() = the exponential function
- erf() = the error function
- sqrt() = the square root function
By plugging in the appropriate values for µ, σ, and x, you can calculate the probability density or cumulative probability for any given value in a log-normal distribution.
Conclusion
The log-normal distribution is a useful tool for modeling variables that are always positive and have a wide range of possible values. It is commonly used in finance, economics, and other fields where skewed distributions are observed. By understanding the concept of the log-normal distribution and how to calculate it, you can better analyze and interpret data in various real-world scenarios.