Home>Finance>What Is A Two-Tailed Test? Definition And Example
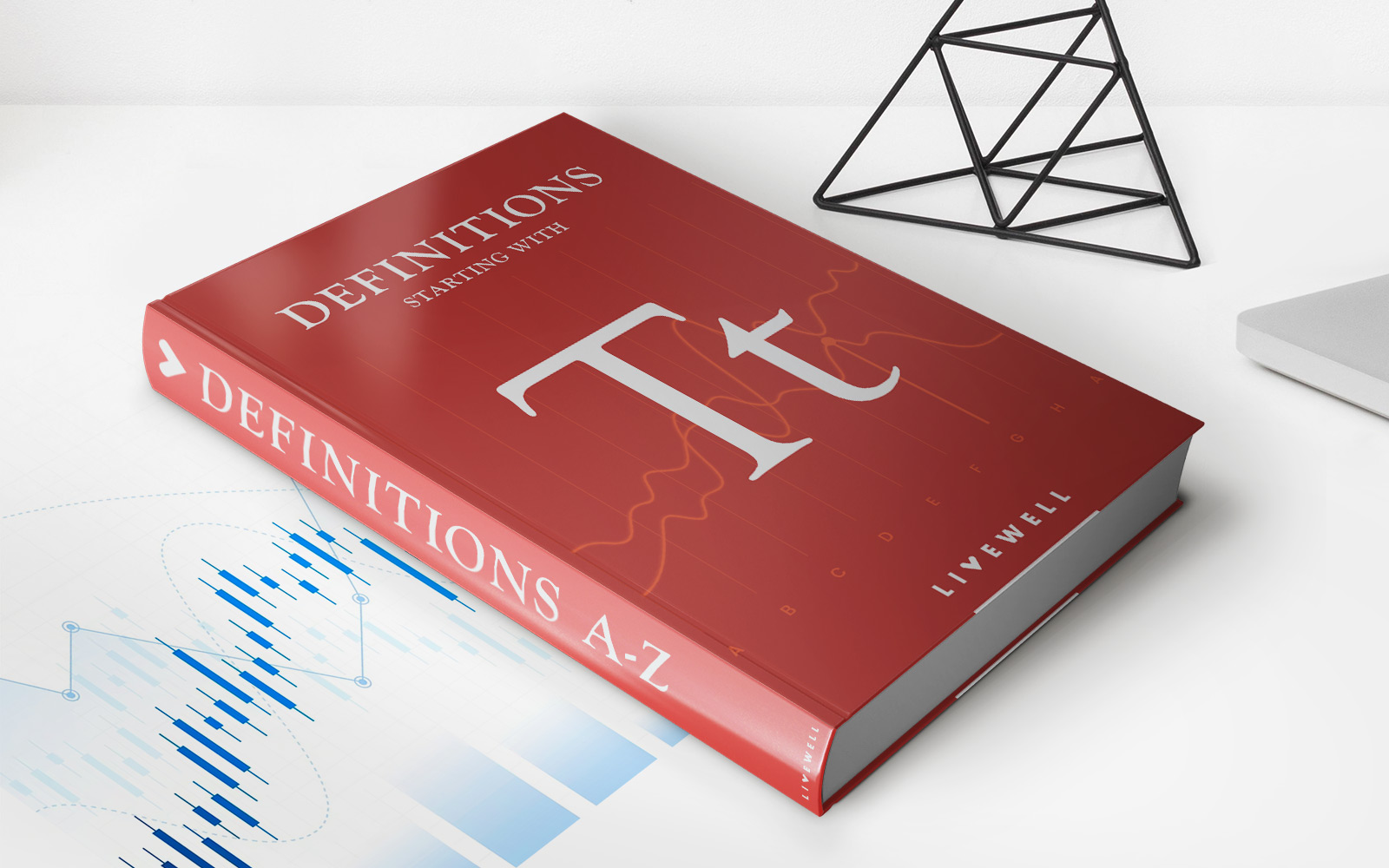
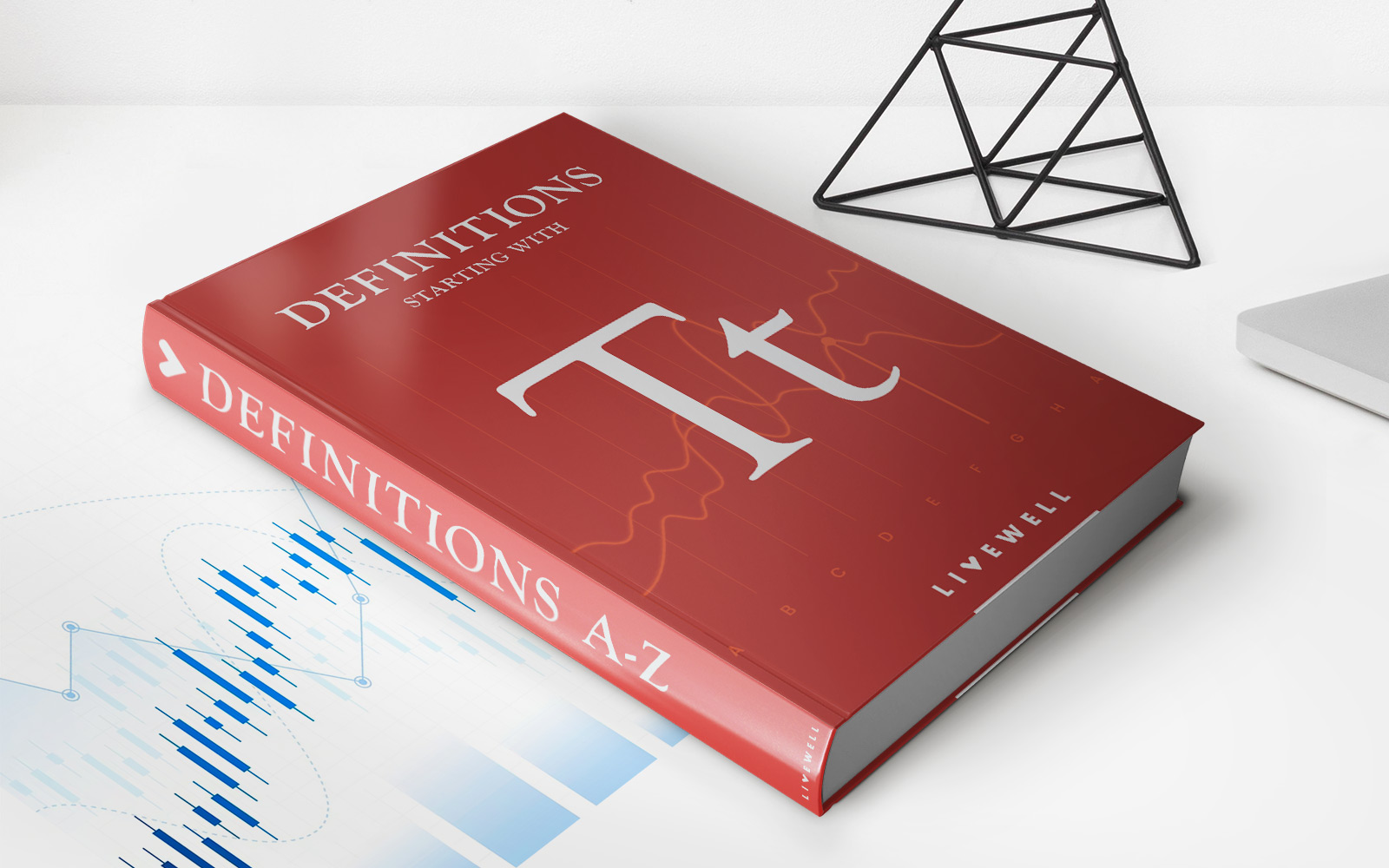
Finance
What Is A Two-Tailed Test? Definition And Example
Published: February 12, 2024
Discover the definition and example of a two-tailed test in finance. Understand how this statistical test helps evaluate hypotheses effectively.
(Many of the links in this article redirect to a specific reviewed product. Your purchase of these products through affiliate links helps to generate commission for LiveWell, at no extra cost. Learn more)
Understanding Two-Tailed Tests: Unlocking the Power of Statistical Significance
Are you familiar with the concept of two-tailed tests? If you work in finance or have an interest in statistical analysis, understanding the ins and outs of this test can be incredibly valuable. In this blog post, we will explore the definition and provide an example of a two-tailed test, explaining why it plays a crucial role in determining statistical significance. So, strap on your thinking cap and let’s dive in!
Key Takeaways:
- A two-tailed test is a statistical hypothesis that considers both extremes of a distribution, allowing us to test whether a relationship exists in either direction.
- It is particularly useful when we want to determine if a parameter is significantly different from a specific value, without relying on a preconceived direction.
What Is a Two-Tailed Test?
In statistical analysis, a two-tailed test refers to a hypothesis test that examines the relationship between variables in both directions. It allows us to assess whether the observed results are significantly different from what we would expect by chance alone, without limiting our analysis to a particular direction of effect. By considering both extremes of a distribution, a two-tailed test offers a more comprehensive approach to evaluating statistical significance.
Before diving into an example, let’s quickly recap the basics. In hypothesis testing, we start with a null hypothesis (H0) that assumes no relationship or difference between the variables of interest. The alternative hypothesis (Ha), on the other hand, represents the researcher’s claim or the proposition they aim to support with evidence.
A two-tailed test departs from the conventional “one-tailed” test by allowing us to assess the significance of a relationship in either direction. It essentially splits the alpha level (typically set at 0.05) into two equal halves, allocating 0.025 to each tail of the distribution. This means that we will reject the null hypothesis if the observed result falls into either tail, indicating a statistically significant difference.
Example of a Two-Tailed Test
Let’s say you are a financial analyst investigating whether a new investment strategy has a significant impact on portfolio returns. You hypothesize that the investment strategy will lead to either significantly higher or lower average returns compared to the market average.
You collect data from a sample of investors who implemented the new strategy and perform a two-tailed test to determine if the strategy has an effect. The null hypothesis states that there is no significant difference between the investment strategy returns and the market average. The alternative hypothesis states that there is a significant difference, either positive or negative.
After conducting the necessary calculations, you obtain your test statistic and compare it with the critical values for your chosen level of significance. If the test statistic falls into either tail of the distribution, beyond the critical value, you reject the null hypothesis and conclude that there is evidence of a significant difference in returns.
It is crucial to note that if the test statistic falls within the range of non-rejection, it does not provide evidence to support the alternative hypothesis. It simply means that there is insufficient evidence to refute the null hypothesis.
Conclusion
A two-tailed test is a powerful tool in statistical analysis, allowing researchers to explore the significance of a relationship in either direction. By considering both extremes of a distribution, it provides a more comprehensive assessment of statistical significance.
So the next time you embark on research in finance or any other field that requires hypothesis testing, remember the importance of a two-tailed test. With its ability to unveil meaningful relationships, it will assist you in drawing more accurate conclusions from your statistical analysis.
Make sure to bookmark this blog post as a handy reference whenever you encounter a two-tailed test in the future. Happy analyzing!