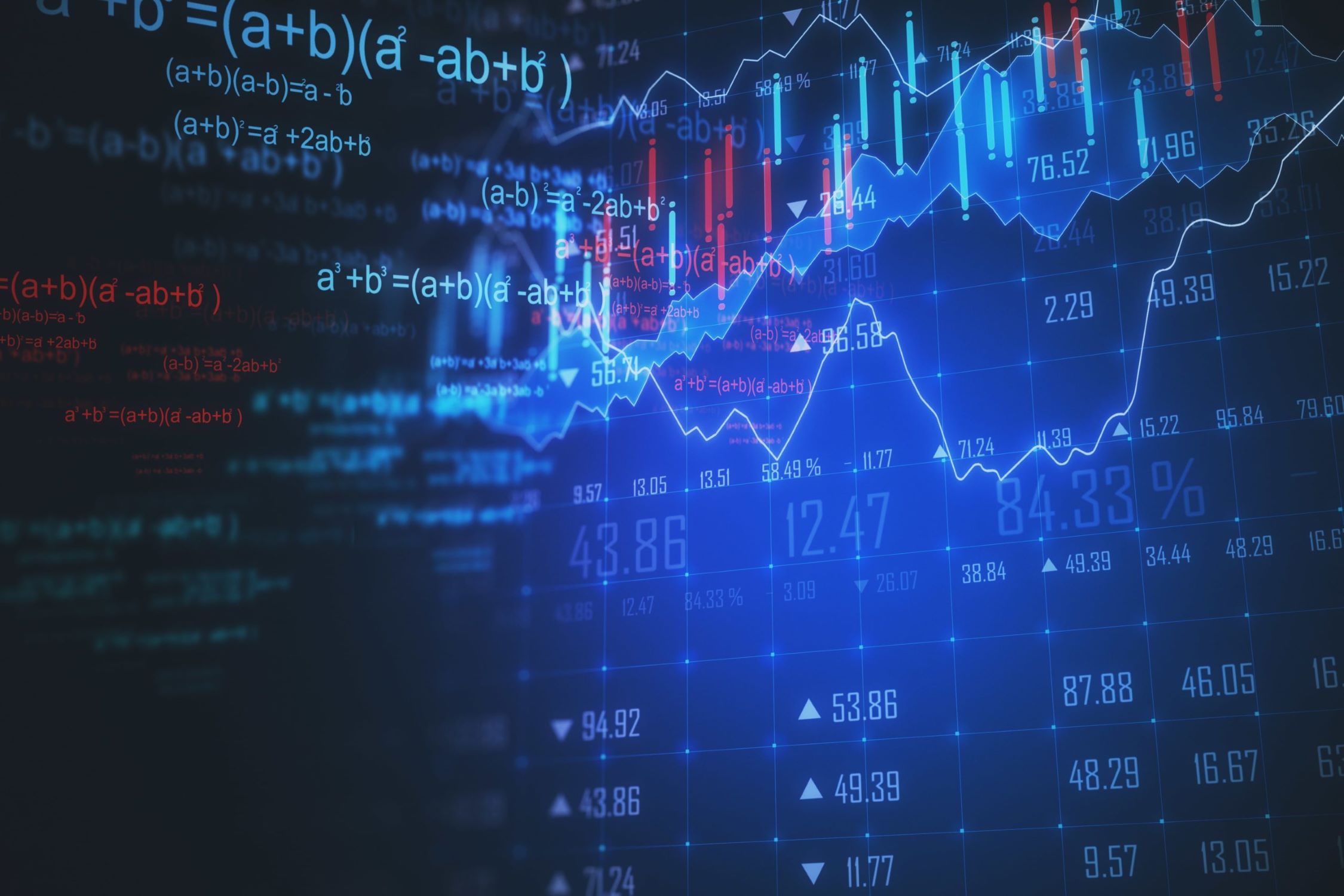
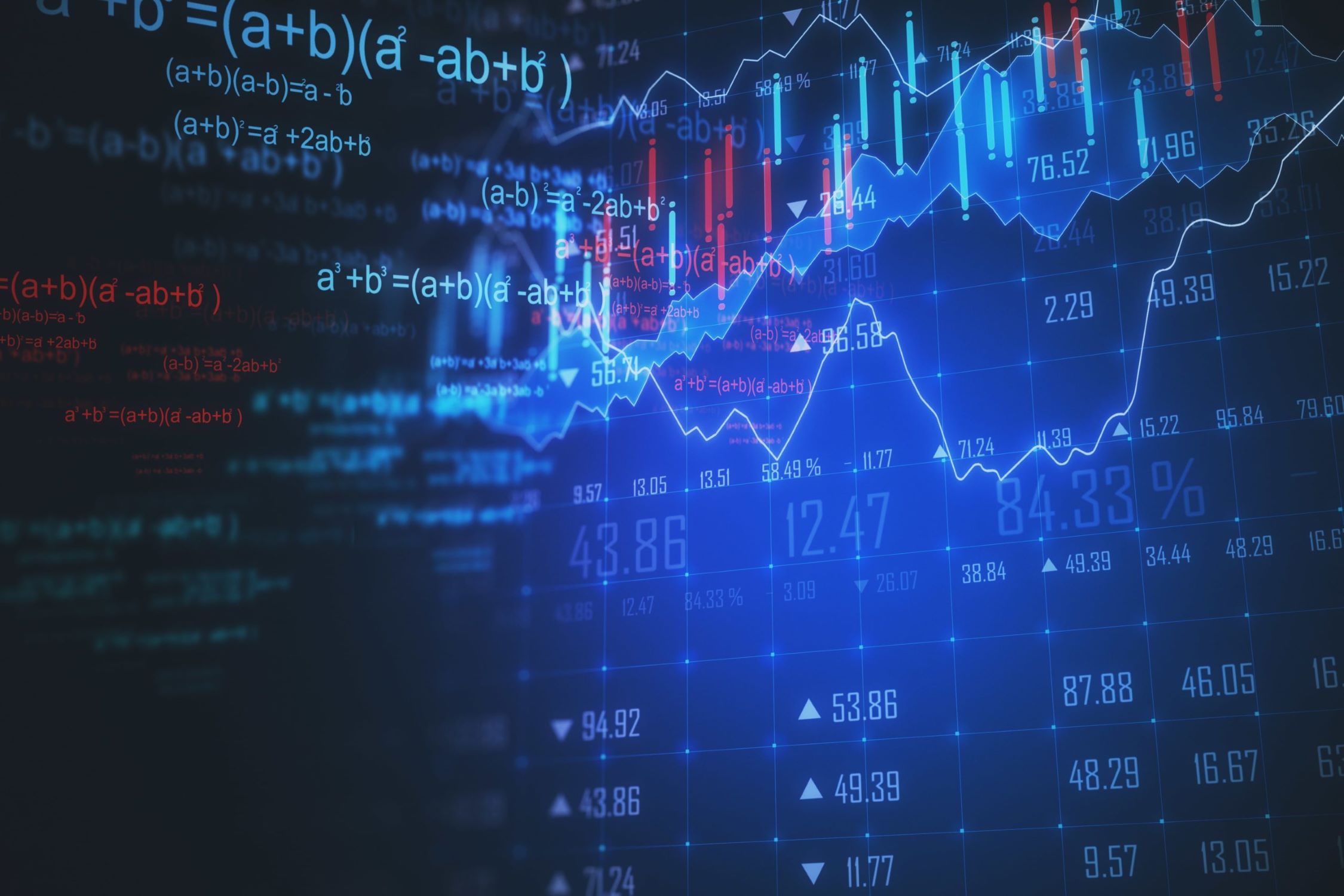
Finance
What Is Quantitative Investment?
Published: October 17, 2023
Learn about quantitative investment in finance and its role in analyzing large amounts of data to make informed investment decisions.
(Many of the links in this article redirect to a specific reviewed product. Your purchase of these products through affiliate links helps to generate commission for LiveWell, at no extra cost. Learn more)
Table of Contents
- Introduction
- Definition of Quantitative Investment
- Key Features of Quantitative Investment
- Advantages of Quantitative Investment
- Disadvantages of Quantitative Investment
- Quantitative Investment Strategies
- Common Metrics and Models Used in Quantitative Investment
- Challenges and Risks in Quantitative Investment
- Case Studies on Successful Quantitative Investment Approaches
- Conclusion
Introduction
Welcome to the world of quantitative investment! In today’s fast-paced financial landscape, where data-driven decisions are vital, the field of quantitative investment has gained significant attention. This approach to investing combines advanced mathematical models, statistical analysis, and computer algorithms to identify and exploit market opportunities.
Quantitative investment is rooted in the belief that financial markets are efficient and that patterns, trends, and anomalies can be harnessed through rigorous analysis. By relying on systematic and data-driven strategies, quantitative investors aim to achieve consistent returns and mitigate the impact of human emotion in investment decision-making.
This article will delve into the world of quantitative investment, exploring its key features, advantages, and disadvantages. We will also discuss various quantitative investment strategies and the common metrics and models used in this approach. Additionally, we will outline the challenges and risks involved in quantitative investment and explore some case studies that demonstrate its potential for success.
Whether you are an aspiring investor looking to learn more about quantitative investment or a seasoned finance professional seeking to expand your knowledge base, this article will provide you with valuable insights into the world of quantitative investment.
Definition of Quantitative Investment
Quantitative investment, also known as quantitative finance or algorithmic trading, is an investment approach that relies on the use of mathematical models, statistical analysis, and computer algorithms to make investment decisions. It involves the systematic collection, analysis, and interpretation of vast amounts of data to identify patterns, correlations, and trends in financial markets.
Unlike traditional investment methods that heavily rely on the expertise and intuition of fund managers, quantitative investment aims to remove subjective biases by relying on data-driven strategies. The goal is to uncover hidden opportunities and exploit market inefficiencies through rigorous analysis and computational power.
Quantitative investment strategies typically involve developing and implementing trading algorithms that generate buy and sell signals based on predefined rules and criteria. These algorithms can process large amounts of data in real time, allowing for rapid decision-making and execution.
Quantitative investors not only rely on historical price data but also incorporate a wide range of financial metrics, macroeconomic data, and other relevant factors into their models. These models can cover various asset classes, including stocks, bonds, commodities, and currencies.
The use of quantitative techniques in investment management has become increasingly prevalent in recent years, thanks to advancements in technology, availability of vast amounts of financial data, and the growing demand for systematic and data-driven approaches in investment decision-making.
Overall, quantitative investment provides a structured and disciplined approach to investing, aiming to generate consistent returns by leveraging mathematical models, statistical analysis, and technology to gain an edge in the financial markets.
Key Features of Quantitative Investment
Quantitative investment offers several key features that set it apart from traditional investment approaches. Understanding these features is essential for investors looking to delve into the world of quantitative investment. Let’s explore the key features of quantitative investment:
- Data-driven approach: Quantitative investment relies on extensive data analysis to drive investment decisions. It involves the collection, cleaning, and processing of vast amounts of financial data to identify patterns and trends. This data-driven approach helps mitigate the impact of emotional biases that can often skew investment decisions.
- Systematic strategies: Quantitative investment employs systematic strategies that follow predefined rules and criteria. These strategies are designed to operate consistently and objectively, without being influenced by human emotions or gut feelings. This systematic approach ensures a disciplined and consistent investment process.
- Advanced mathematical models: Quantitative investment relies on advanced mathematical models to extract meaningful insights from complex financial data. These models may include statistical techniques, regression analysis, time series analysis, and machine learning algorithms to identify market patterns and relationships.
- Risk management: Quantitative investment places great emphasis on risk management. Through sophisticated risk models and algorithms, quantitative investors aim to identify and manage various forms of risk, including market risk, credit risk, and liquidity risk. This disciplined approach helps mitigate the impact of unforeseen events and market fluctuations.
- Technology and automation: Quantitative investment heavily relies on technology and automation to gather, process, and execute investment strategies. High-speed computers, powerful algorithms, and advanced trading systems enable quantitative investors to analyze vast amounts of data and execute trades in real time. This technological advantage allows for quicker decision-making and execution compared to traditional investment approaches.
These key features of quantitative investment demonstrate the unique characteristics and advantages that quantitative strategies bring to the investment landscape. By leveraging data-driven analysis, systematic methodologies, advanced mathematical models, risk management techniques, and technological advancements, quantitative investment aims to generate consistent and optimized returns for investors.
Advantages of Quantitative Investment
Quantitative investment offers several advantages that have contributed to its popularity among investors and finance professionals. These advantages stem from its systematic and data-driven approach, as well as the utilization of advanced mathematical models and technology. Let’s explore the key advantages of quantitative investment:
- Objectivity and Discipline: Quantitative investment strategies are built on predefined rules and criteria, eliminating emotions and biases from the investment decision-making process. By relying on data-driven models and systematic approaches, quantitative investors can make consistent and rational investment decisions without succumbing to market noise or subjective judgment.
- Backed by Research and Analysis: Quantitative investment involves extensive research and analysis using historical data, financial metrics, and mathematical models. This rigorous approach helps identify patterns, trends, and relationships in financial markets that may not be apparent to human analysis. Through thorough testing and validation, quantitative strategies are based on robust empirical evidence.
- Faster Decision-Making: Quantitative investment leverages technology and automation to analyze vast amounts of data and execute trades in real time. This allows for faster decision-making and execution compared to traditional investment approaches. Rapid response to market opportunities and adjustments to risk exposure can be made efficiently, which can be advantageous in volatile market conditions.
- Ability to Handle Large Data Sets: Quantitative investment is designed to handle large data sets and extract valuable insights from them. By processing and analyzing large amounts of data, quantitative strategies can capture market inefficiencies that may be missed by manual analysis. This ability to handle big data gives quantitative investors a potential edge in identifying profitable investment opportunities.
- Risk Management: Quantitative investment places great emphasis on risk management. Through the use of sophisticated risk models and algorithms, quantitative investors can identify and manage different types of risks, such as market risk, credit risk, and liquidity risk. This disciplined approach helps protect investment portfolios from unexpected market events and minimize downside risks.
The advantages of quantitative investment make it an attractive option for investors seeking a systematic and data-driven approach to portfolio management. The objectivity, research-backed strategies, faster decision-making, ability to handle large data sets, and robust risk management techniques contribute to the potential for generating consistent returns and reducing investment biases.
Disadvantages of Quantitative Investment
While quantitative investment offers numerous advantages, it is important to be aware of the potential disadvantages and challenges associated with this approach. Understanding these drawbacks can help investors make informed decisions regarding the suitability of quantitative strategies for their investment goals. Let’s explore some of the key disadvantages of quantitative investment:
- Overreliance on Historical Data: Quantitative investment models heavily rely on historical data to identify patterns and generate investment signals. However, market conditions and dynamics can change, rendering historical data less relevant or misleading. It is important to continuously update and validate models to ensure they remain effective in the current market environment.
- Complexity and Model Risk: Developing and maintaining quantitative investment models can be complex and resource-intensive. The use of sophisticated mathematical models and algorithms introduces the risk of model errors or inaccuracies. Models may not capture all market dynamics and can be vulnerable to unforeseen events or structural shifts in the market.
- Data Limitations: While quantitative investment relies on vast amounts of data, the quality, accuracy, and availability of data can vary. Incomplete or inaccurate data can lead to flawed analysis and decision-making. Data limitations may also arise when dealing with less liquid or unstructured markets, where reliable and comprehensive data may be scarce.
- Black Box Nature: Some quantitative investment strategies can be seen as “black boxes” due to the complex algorithms and models involved. This lack of transparency can make it difficult for investors to fully understand and evaluate the underlying investment process. Lack of transparency can also hinder the ability to explain investment decisions to clients or regulators.
- Changing Market Conditions: Quantitative investment strategies may struggle to adapt in rapidly changing market conditions or during periods of market stress. Models that perform well under certain market conditions may not perform as expected in different market environments. Continuous monitoring and adjustment of models are necessary to ensure they remain effective.
It is important to note that these disadvantages do not negate the potential benefits of quantitative investment. However, they highlight the need for careful consideration and active management to address the challenges inherent in this approach. Successful quantitative investors employ risk management strategies, ongoing research and development, and constant monitoring to mitigate these disadvantages and maximize the potential of quantitative investment.
Quantitative Investment Strategies
Quantitative investment encompasses a wide range of strategies that utilize mathematical models, statistical analysis, and computer algorithms to make investment decisions. These strategies aim to capitalize on market inefficiencies, identify profitable opportunities, and manage risk. Let’s explore some common quantitative investment strategies:
- Momentum Investing: This strategy seeks to identify and capitalize on trends in asset prices. It involves buying assets that have shown positive price momentum and selling assets that have shown negative momentum. Momentum investors believe that assets with recent positive performance tend to continue their upward trend, while assets with negative performance are likely to continue declining.
- Mean Reversion: The mean reversion strategy assumes that asset prices tend to revert to their mean or average price over time. This strategy involves buying assets that have recently experienced negative price movements, expecting them to bounce back, and selling assets that have recently experienced positive price movements, expecting them to decline in value.
- Arbitrage: Arbitrage strategies aim to exploit price discrepancies between different markets or assets. Quantitative investors look for mispriced assets or divergences in prices and take advantage of them by simultaneously buying and selling related securities to lock in profits. Arbitrage strategies often require sophisticated models, real-time data, and low-latency trading systems.
- Pairs Trading: Pairs trading involves identifying two closely related assets that historically move together. Quantitative investors simultaneously take a long position in one asset and a short position in the other, expecting the prices to converge. This strategy aims to profit from the relative performance difference between the two assets, irrespective of the overall market movement.
- Fundamental Factor Models: Fundamental factor models utilize financial metrics and ratios, such as earnings, revenue, and book value, to assess the intrinsic value of securities. These models rank securities based on their relative attractiveness and select portfolios that are expected to outperform the market. Fundamental factor models seek to identify undervalued securities and avoid overvalued ones.
- Quantitative Trend Following: Trend following strategies aim to capture and profit from sustained trends in asset prices. Quantitative investors analyze historical price data to identify trends and generate buy or sell signals accordingly. Trend following strategies often include risk management techniques to protect against false signals and minimize downside risk.
It’s important to note that these strategies are not exhaustive, and quantitative investors often combine different approaches or develop their own unique strategies based on their research and expertise. Successful implementation of quantitative investment strategies requires thorough testing, rigorous risk management, continuous monitoring, and adaptation to changing market conditions.
Common Metrics and Models Used in Quantitative Investment
Quantitative investment relies on the use of various financial metrics and mathematical models to analyze and interpret market data. These metrics and models provide insights into market trends, valuation, risk, and other important factors. Let’s explore some common metrics and models used in quantitative investment:
- Price-to-Earnings (P/E) Ratio: The P/E ratio is a commonly used valuation metric that compares a company’s stock price to its earnings per share. It helps investors assess whether a stock is overvalued or undervalued relative to its earnings potential.
- Return on Equity (ROE): ROE measures a company’s profitability by comparing its net income to its shareholders’ equity. It indicates how effectively a company is generating profits from its invested capital and can help assess its financial health and growth potential.
- Standard Deviation: Standard deviation measures the volatility or variability of an asset’s returns. It helps quantify the risk associated with an investment by assessing how much an asset’s returns deviate from its average return. Lower standard deviation indicates lower risk, while higher standard deviation suggests higher risk.
- Sharpe Ratio: The Sharpe ratio is a risk-adjusted performance measure that considers the excess return of an investment relative to its volatility. It helps evaluate the risk-adjusted return of an investment and can be used for comparing different investment opportunities or portfolios.
- Capital Asset Pricing Model (CAPM): CAPM is a widely used model in finance that estimates the expected return of an investment based on its systematic risk or beta. It provides a framework for assessing the appropriate risk-adjusted return for an asset, taking into account its relationship with the overall market.
- Moving Averages: Moving averages are commonly used to identify trends and potential trading signals. They smooth out price data by averaging it over a specific time period. For example, a 50-day moving average calculates the average price over the past 50 trading days, providing insights into the trend direction.
- Machine Learning Models: Machine learning techniques, such as regression analysis, decision trees, and neural networks, are increasingly used in quantitative investment. These models can analyze large volumes of data and identify complex patterns that may not be discernible through traditional statistical methods. Machine learning models can be applied to various aspects of quantitative investment, including prediction, classification, and anomaly detection.
These metrics and models are just a snapshot of the wide range of tools and techniques used in quantitative investment. The selection of specific metrics and models depends on the investment strategy, asset class, and the investor’s preferences. It is important for quantitative investors to continuously refine and test their models, adapt to changing market dynamics, and incorporate new metrics or models as needed.
Challenges and Risks in Quantitative Investment
While quantitative investment has its advantages, it also comes with its fair share of challenges and risks. Understanding and managing these challenges is crucial for successful implementation of quantitative strategies. Let’s explore some of the main challenges and risks in quantitative investment:
- Data Quality and Availability: Quantitative investment heavily relies on data, and the quality and availability of data can pose challenges. Inaccurate or incomplete data can lead to flawed analysis and unreliable results. It’s important to ensure data integrity, validate data sources, and address any limitations in data availability.
- Model Assumptions and Limitations: Quantitative models are based on assumptions about market behavior and relationships. These assumptions may not always hold true, leading to model inaccuracies and limitations. It’s important to regularly assess and update models, validate assumptions, and consider alternative approaches when necessary.
- Model Overfitting: Overfitting occurs when a model is excessively fine-tuned to historical data, resulting in poor performance on new or unseen data. This can lead to false signals or overly optimistic expectations. To mitigate overfitting, robust testing, rigorous validation, and proper out-of-sample testing should be conducted.
- Changing Market Conditions: Quantitative strategies may struggle to adapt to changing market conditions. Models that worked well in the past may lose effectiveness when market dynamics shift. It’s important to monitor and adapt models to ensure they remain relevant and effective in different market environments.
- Execution Risks: Execution risks arise from the process of implementing trades based on quantitative signals. Factors such as market liquidity, order placement, and execution speed can impact the actual performance achieved compared to model-driven expectations. Proper trade execution strategies and technology infrastructure are crucial for minimizing execution risks.
- Regulatory and Compliance Risks: Quantitative investment involves complex models and algorithms, which may raise regulatory and compliance concerns. Proper documentation, transparency, and adherence to applicable regulations are essential to ensure compliance with legal and ethical standards. Compliance with risk management guidelines and reporting requirements is also critical.
- Human Error and Behavioral Biases: While quantitative investment aims to remove subjective biases, human error can still occur in data collection, model development, or execution. Additionally, human biases can influence the interpretation of quantitative signals or override systematic decisions. Careful oversight, robust validation processes, and adherence to disciplined investment processes can help mitigate such risks.
Quantitative investment involves a constant balancing act between risk and reward. It requires ongoing monitoring, robust risk management, and continuous improvement to address the challenges and risks inherent in this approach. By carefully managing these challenges, investors can enhance the effectiveness and success of their quantitative investment strategies.
Case Studies on Successful Quantitative Investment Approaches
Quantitative investment has gained traction among investors due to its potential for generating consistent returns and managing risk. Here are a few notable case studies that highlight successful quantitative investment approaches:
- Renaissance Technologies: Renaissance Technologies, founded by James Simons, is considered one of the most successful quantitative hedge funds. Their Medallion Fund, which employs complex mathematical models and high-frequency trading, has consistently delivered impressive returns. Over a span of more than 30 years, Medallion Fund has achieved annualized returns of over 35%, even after accounting for fees and expenses.
- Two Sigma: Two Sigma, founded by David Siegel and John Overdeck, is another prominent quantitative investment firm. Their strategies utilize machine learning and data analysis to identify market signals. Two Sigma’s funds have delivered strong performance, with their Absolute Return fund recording double-digit annualized returns over the years.
- AQR Capital Management: AQR Capital Management, founded by Cliff Asness, employs a systematic and factor-based approach to investing. Their investment strategies focus on factors such as value, momentum, and quality. AQR’s funds have demonstrated solid performance, supported by their rigorous research and risk management methodologies.
- Quantitative Trading Firms: Several quantitative trading firms have excelled in the field of high-frequency trading. These firms, such as Citadel Securities, Jump Trading, and DRW, utilize advanced algorithms and technology to analyze vast amounts of data and execute trades rapidly. They have achieved significant success by taking advantage of market microstructure and exploiting short-term price discrepancies.
- Smart Beta Strategies: Smart beta strategies, which aim to capture factors like value, volatility, or quality in a rules-based manner, have gained popularity among investors. Asset management firms like BlackRock and Invesco offer smart beta ETFs that provide systematic exposure to these factors. These strategies have shown to outperform traditional index strategies over the long term.
These case studies highlight the success stories of quantitative investment approaches implemented by various firms and individuals. However, it is important to note that past performance is not indicative of future results, and risks are inherent in any investment strategy. Furthermore, success in quantitative investment requires continuous adaptation, risk management, and adherence to disciplined investment processes.
Conclusion
Quantitative investment has revolutionized the world of finance, offering a systematic and data-driven approach to investing. By harnessing advanced mathematical models, statistical analysis, and technology, quantitative investors aim to identify profitable opportunities, manage risk, and achieve consistent returns.
Throughout this article, we have explored the definition of quantitative investment, its key features, advantages, and disadvantages. We have delved into various quantitative investment strategies, such as momentum investing, mean reversion, arbitrage, and fundamental factor models. Additionally, we have discussed the common metrics and models used in quantitative investment, as well as the challenges and risks inherent in this approach.
While quantitative investment offers numerous benefits, it is not without its challenges. The reliance on historical data, model assumptions, and changing market conditions requires continuous adaptation and careful risk management. The availability and quality of data, as well as the potential for human error and biases, also present challenges in implementing successful quantitative strategies.
Despite the challenges, quantitative investment has demonstrated its potential for success through various case studies of firms like Renaissance Technologies, Two Sigma, and AQR Capital Management. These firms’ strong performance showcases the effectiveness of their quantitative investment approaches in generating consistent returns over the long term.
In conclusion, quantitative investment provides investors with a systematic and disciplined framework for decision-making. By utilizing advanced models, rigorous analysis, and technology, quantitative investors strive to gain an edge in the financial markets. However, it is crucial to remember that successful quantitative investment requires ongoing refinement, validation, risk management, and adaptability to changing market dynamics. By understanding the principles and complexities of quantitative investment, investors can make informed decisions and potentially enhance their investment outcomes.